Deterministic construction of compressed sensing matrices from constant dimension codes
Discrete Applied Mathematics(2020)
摘要
Compressed sensing theory provides a new approach to acquire data as a sampling technique and makes sure that sparse signals can be reconstructed from few measurements. The construction of compressed sensing matrices is a central problem in compressed sensing theory. In this paper, the deterministic sparse compressed sensing matrices from constant dimension codes are constructed and the coherence of the matrices are computed. Furthermore, the maximum sparsity of recovering the sparse signals by using our matrices is obtained. Meanwhile, the numerical simulations are made among our matrices from constant dimension codes, R. DeVore’s matrices, matrices from p-ary BCH codes and random matrices. Moreover, the deterministic sparse compressed sensing matrices can be constructed from certain Steiner structures. Finally, a general analysis indicates that the deterministic sparse compressed sensing matrices from constant dimension codes can recover signals of arbitrary sparsity order with any number of budged rows in some Euclid spaces with proper dimensions.
更多查看译文
关键词
Compressed sensing,Coherence,Sparsity,Restricted isometry property,Constant dimension codes
AI 理解论文
溯源树
样例
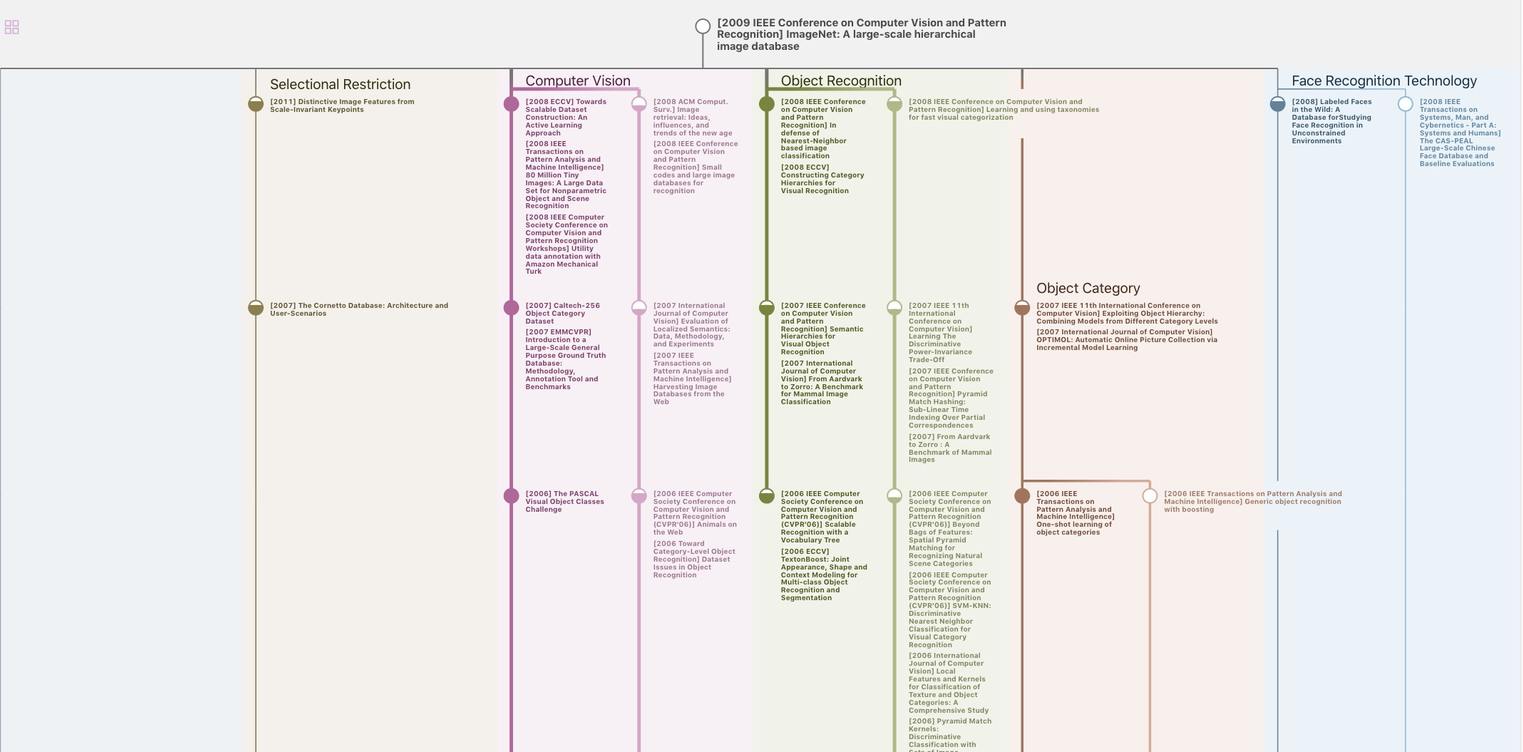
生成溯源树,研究论文发展脉络
Chat Paper
正在生成论文摘要