Yield modeling of snap bean based on hyperspectral sensing: a greenhouse study
JOURNAL OF APPLIED REMOTE SENSING(2020)
摘要
Farmers and growers typically use approaches based on the crop environment and local meteorology, many of which are labor-intensive, to predict crop yield. These approaches have found broad acceptance but lack real-time and physiological feedback for near-daily management purposes. This is true for broad-acre crops, such as snap bean, which is valued at hundreds of millions of dollars in the annual agricultural market. We aim to investigate the relationships between snap bean yield and plant spectral and biophysical information, collected using a hyperspectral spectroradiometer (400 to 2500 nm). The experiment focused on 48 single snap bean plants (cv. Huntington) in a controlled greenhouse environment during the growth period (69 days). We used applicable accuracy and precision metrics from partial least squares regression and cross-validation methods to evaluate the predictive ability of two harvest stages, namely an early-harvest and late-harvest stage, against our yield indicator (bean pod weight). Four different spectral data sets were used to investigate whether such oversampled, hyperspectral data sets could accurately and precisely model observed variability in yield, in terms of the coefficient of determination (R-2) and root-mean-square error (RMSE). The objective of our approach hinges on the philosophy that selected spectral bands from this study, i.e., those that best explain yield variability, can be downs ampled from a hyperspectral system for use in a more cost-effective, operational multispectral sensor. Our results suggested the optimal period for spectral evaluation of snap bean yield is 20 to 25 or 32 days prior to harvest for the early- and late-harvest stages, respectively, with the best model performing at a low RMSE (3.02 g plant(-1)) and a high coefficient of determination (R-2 = 0.72). An unmanned aerial systems-mounted, affordable, and wavelength-programmable multispectral imager, with bands corresponding to those identified, could provide a near real-time and reliable yield estimate prior to harvest. (C) The Authors. Published by SPIE under a Creative Commons Attribution 4.0 Unported License.
更多查看译文
关键词
precision agriculture,yield prediction,snap bean,hyperspectral,partial least squares regression
AI 理解论文
溯源树
样例
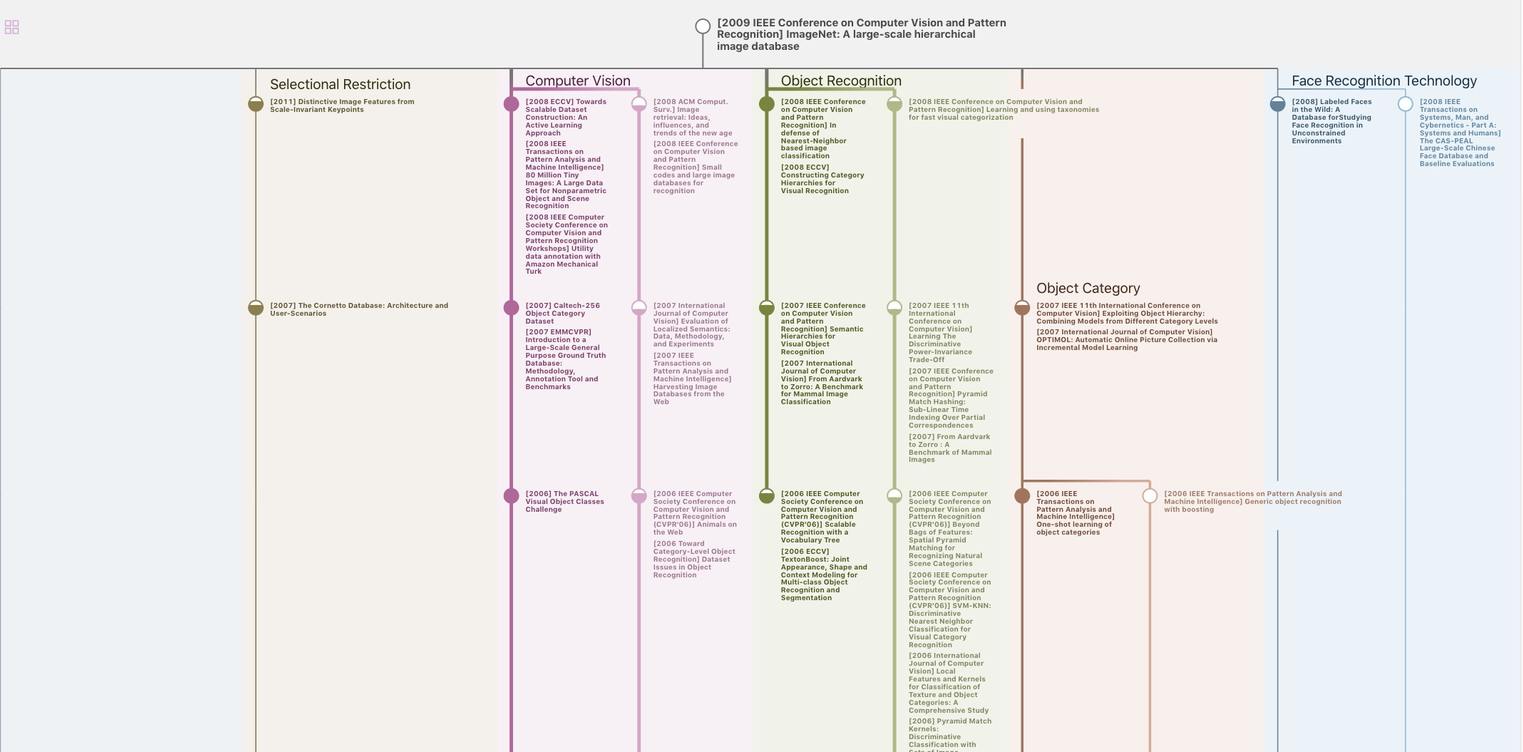
生成溯源树,研究论文发展脉络
Chat Paper
正在生成论文摘要