Multi-Gradient Convolutional Neural Network Based In-Loop Filter For Vvc
2020 IEEE International Conference on Multimedia and Expo (ICME)(2020)
摘要
While recent researches on convolutional neural network (CNN) based in-loop filters for High Efficiency Video Coding (HEVC) have achieved great success, the performance of these models on the new standard Versatile Video Coding (VVC) may degrade due to many novel adopted techniques which make the compression process more fine and capture more image details. In this work, the performances on VVC of two CNN based in-loop filters proposed for HEVC are investigated and a multi-gradient convolutional neural network based in-loop filter (MGNLF) for VVC is proposed. The proposed model exploits the divergence and second derivative of frame, which contain much potential image structural information, like contour information, to restore more detail information to further improve the quality of frames. Experimental results demonstrate our approach can significantly improve the coding performance. On average, 3.29% BD-Rate reduction is achieved on luma component under all intra configuration compared with the original VVC with DBF and SAO enabled, which also outperforms other state-of-the-art approaches for VVC.
更多查看译文
关键词
Versatile Video Coding,in-loop filter,multi-gradient,convolutional neural network
AI 理解论文
溯源树
样例
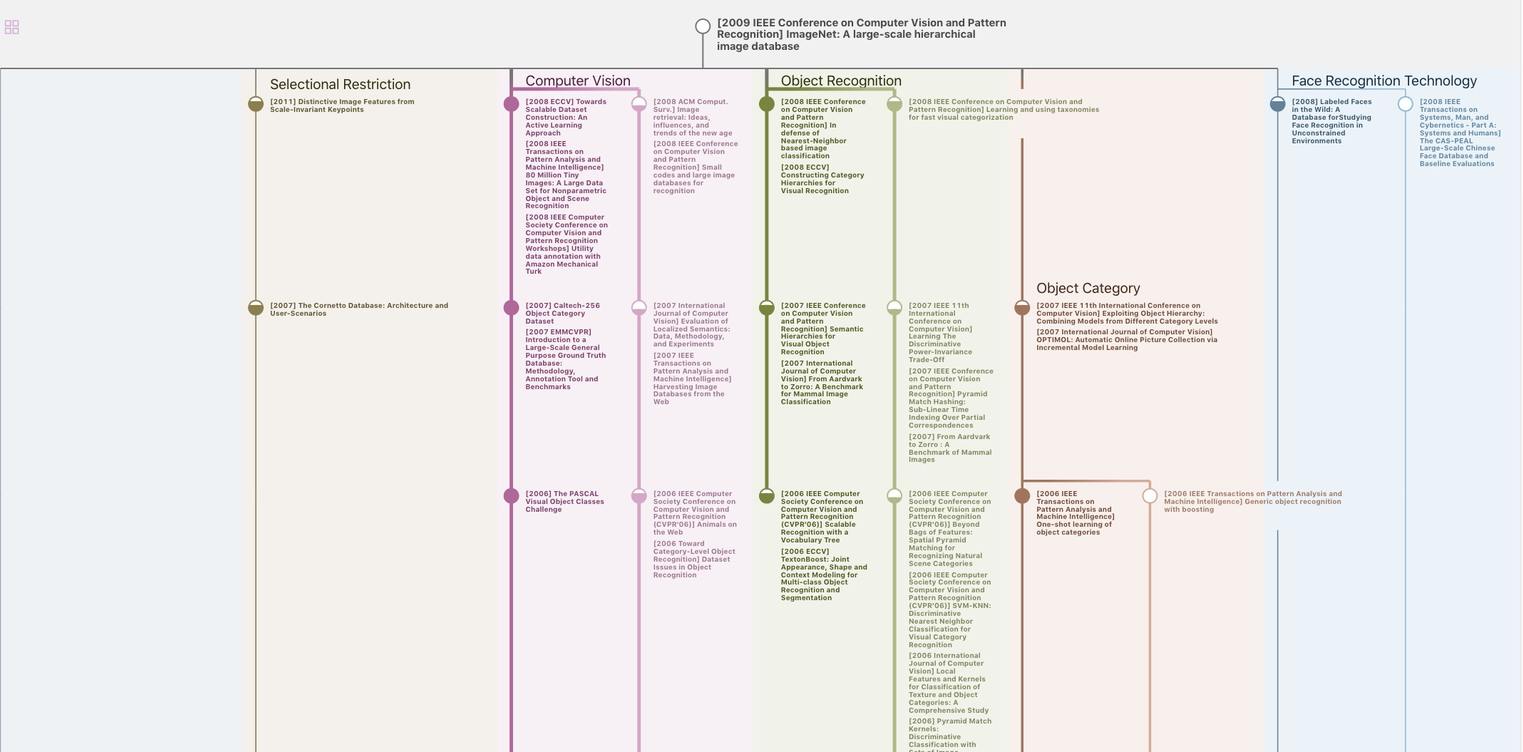
生成溯源树,研究论文发展脉络
Chat Paper
正在生成论文摘要