Network Architecture Reasoning Via Deep Deterministic Policy Gradient
2020 IEEE International Conference on Multimedia and Expo (ICME)(2020)
摘要
In this paper, we introduce global compression learning (GCL) for finding reduced network architecture from a pre-trained network by removing both intra-layer and inter-layer structural redundancy. To accomplish this, we first derive architecture features from a binary representation of the network structure that effectively characterize the relationships between different layers. We then leverage reinforcement learning to iteratively compress the network via deep deterministic policy gradient based on the learned architecture features. To void extensive exploration of the huge space of network architectures, we bound feasible solutions within a small subspace by following a strict accuracy loss tolerance. Benchmarking tests show GCL outperforms the state-of-the-art models. On CIFAR-10 dataset, our model reduces 60.5% FLOPs and 93.3% parameters on VGG-16 without hurting the network accuracy, and yields a significantly compressed architecture for ResNet-110 by reductions of 71.92% FLOPs and 79.62% parameters with the cost of only 0.11% accuracy loss.
更多查看译文
关键词
Network compression,reinforcement learning,policy gradient
AI 理解论文
溯源树
样例
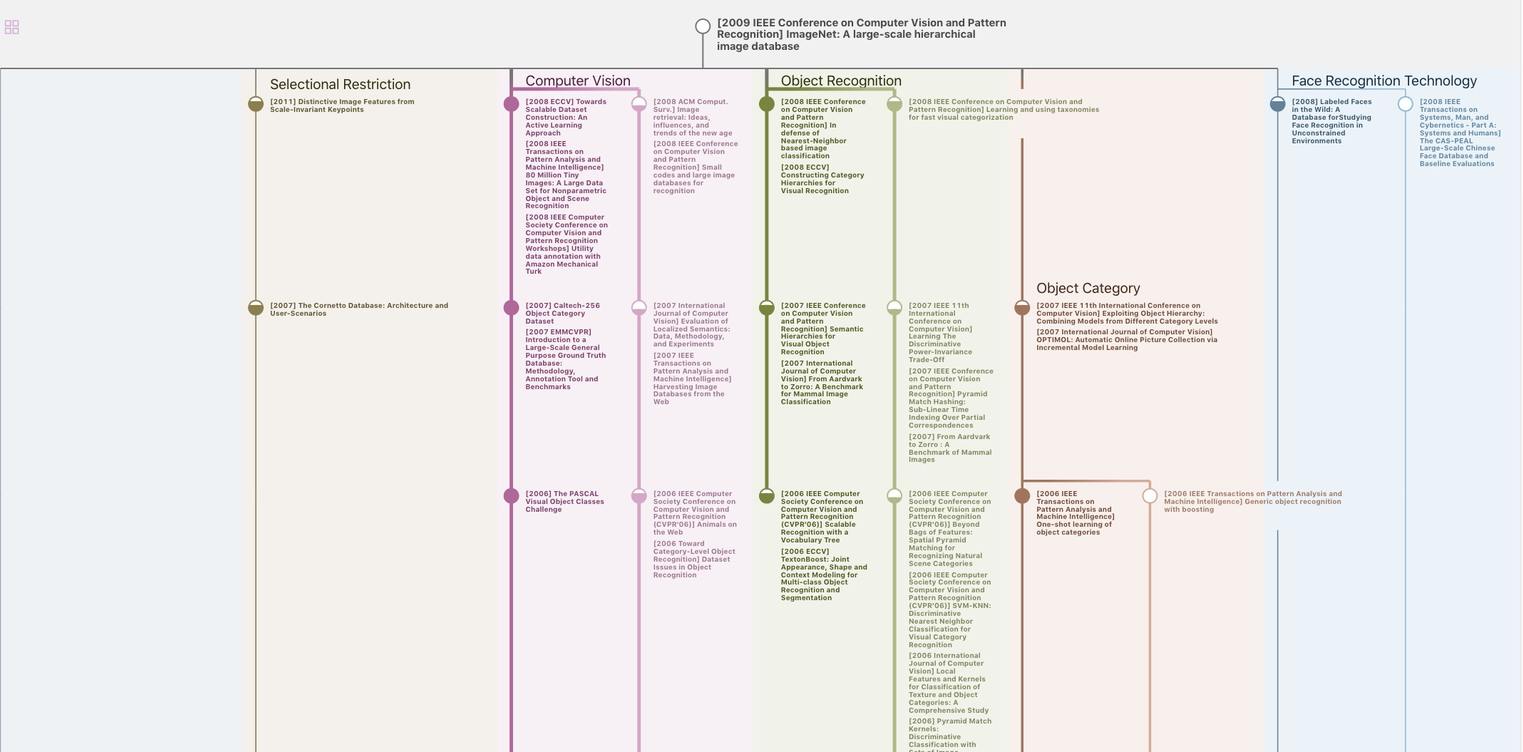
生成溯源树,研究论文发展脉络
Chat Paper
正在生成论文摘要