Identification High Influential Articles By Considering The Topic Characteristics Of Articles
IEEE ACCESS(2020)
摘要
The topic of one article reflects its main semantic content, which is also the main guidance for researchers to choose reference literature. In order to explore whether the topic of an article will affect its citation trend in future, this paper establishes a machine learning framework to study the role of topic characteristics in the prediction of future high influential articles. Articles from four different disciplines are collected as experimental samples to verify whether the framework proposed in this paper can be applied to the prediction task in different disciplines. The Latent Dirichlet Allocation (LDA) is used to determine the topic characteristics of sample articles. LDA can map sample articles to current hot topics and generate the mapping probability of sample articles under different hot topics. The maximum mapping probability of the sample article under the hot topics is extracted as the topic feature of the article. Then the feature space for the prediction task is constructed by combining the topic feature and some bibliometrics indices of articles. Three feature selection algorithms, Fisher Score, Relief-F and Spectral Feature Selection (SPEC), are taken to select the important features in the feature space. The prediction performance of these features is finally tested by three classifiers, SVM, KNN and Bagging. The experimental results show that the topic characteristics of article, the early citation characteristics of article, and the reputation of the author are the key factors that determine whether an article can grow into a highly influential one. The important value of topic characteristics in articles' citation activities shows that the content of the article is an important factor in attracting more citations.
更多查看译文
关键词
Feature extraction, Bibliometrics, Task analysis, Semantics, Physics, Oncology, Neurology, High influential articles, topic characteristics, LDA, feature extraction
AI 理解论文
溯源树
样例
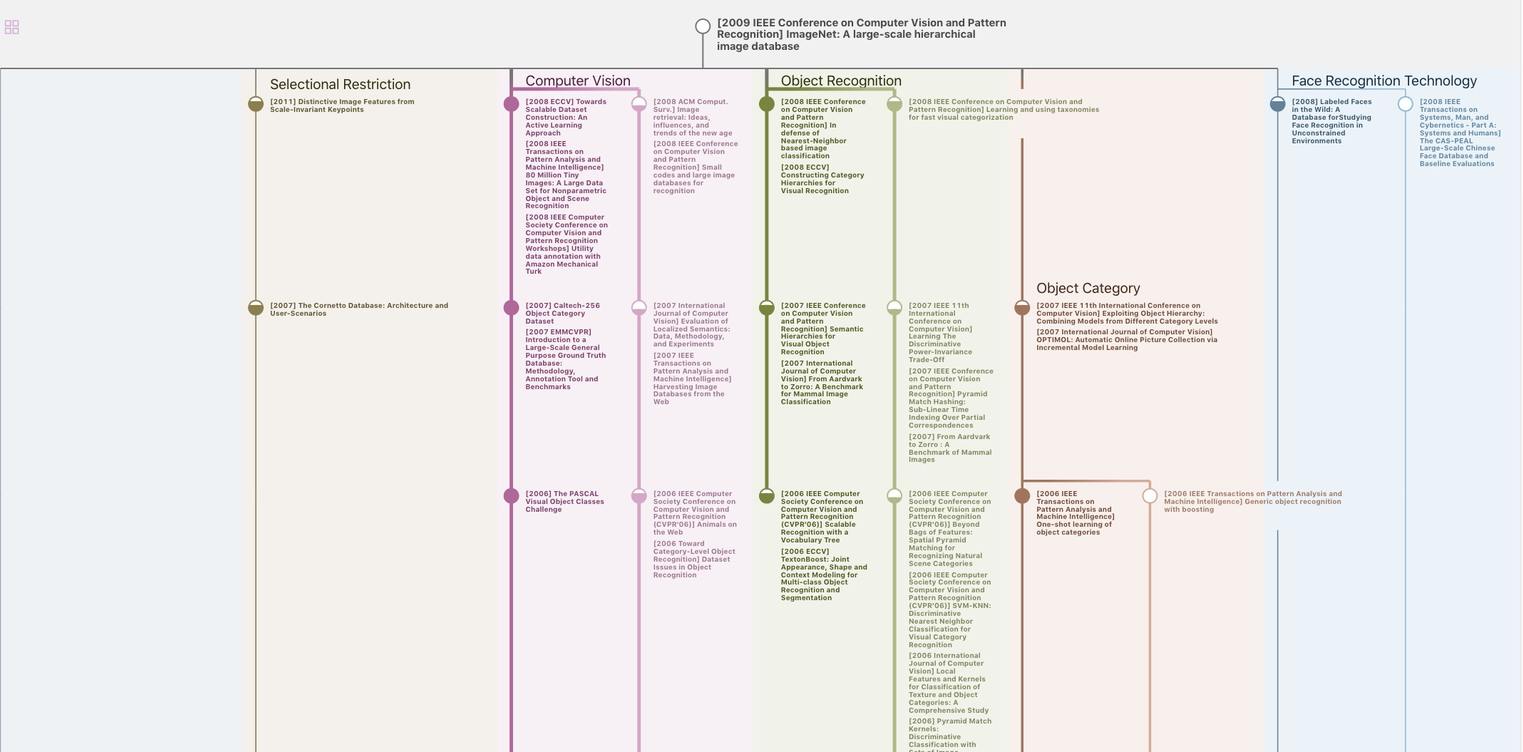
生成溯源树,研究论文发展脉络
Chat Paper
正在生成论文摘要