Similarity-based Particle Filter for Remaining Useful Life prediction with enhanced performance
Applied Soft Computing(2020)
摘要
This paper proposes a similarity-based Particle Filter (PF) method for Remaining Useful Life (RUL) prediction with improved performance. In the proposed methodology, Maximum Mean Discrepancy (MMD) and Kernel Two Sample Test are firstly adopted to query similar Run-To-Failure (R2F) profiles from historical data library. The states and parameters of degradation are initialized based on the similar R2F profiles. Next, Rao–Blackwellized Particle Filter (RBPF) is employed to update the degradation states based on the initialization. The RUL prediction results are obtained by extrapolating the degradation states updated by RBPF. The proposed RUL prediction method holds several advantages: (1) compared with other PF methods, the proposed model includes historical knowledge from similar R2F profiles; (2) compared with similarity-based methods, the proposed model presents good probabilistic interpretation of prediction uncertainties based on RUL distribution. The effectiveness and superiority over other peer algorithms are justified based on a public aero-engine dataset for prognostics.
更多查看译文
关键词
Remaining Useful Life,Rao–Blackwellized Particle Filter,Maximum Mean Discrepancy,Kernel Two Sample Test,NASA C-MAPSS Dataset
AI 理解论文
溯源树
样例
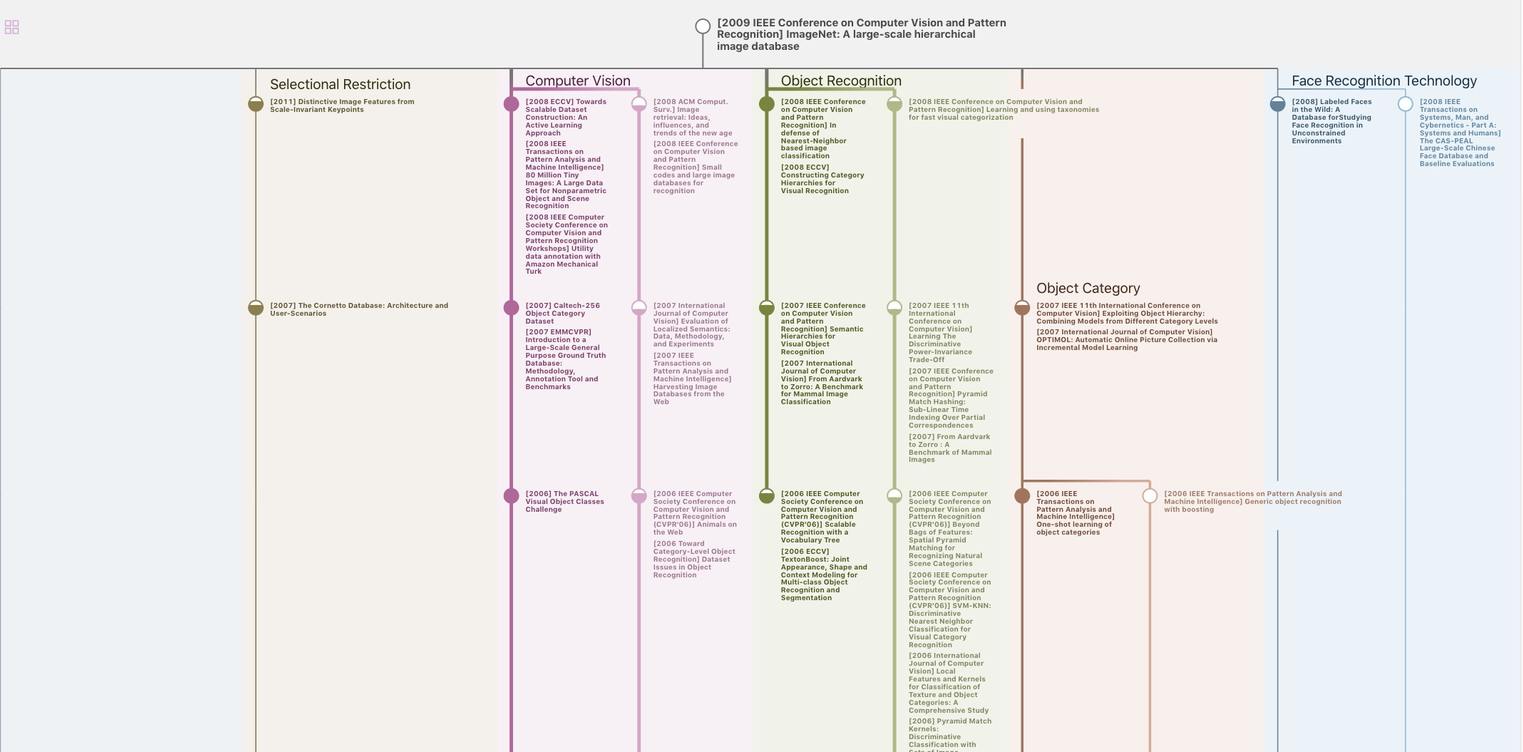
生成溯源树,研究论文发展脉络
Chat Paper
正在生成论文摘要