ABP: Adaptive Body Partition Model For Visible Infrared Person Re-Identification
2020 IEEE International Conference on Multimedia and Expo (ICME)(2020)
摘要
Person re-identification (Re-ID) aims to match pedestrian images cross multiple cameras. Most Re-ID studies focus on visible pedestrian images, without considering the images obtained by infrared cameras in the dark. To solve the cross-modality person Re-ID problem, current methods usually exploit global feature descriptors to obtain discriminative representations. However, they ignore the fine-grained information of heterogeneous images. In this paper, we propose an adaptive body partition (ABP) model to automatically detect and distinguish effective part representations. Instead of utilizing a two-stream convolutional neural network (CNN) to extract modality-specific information, we directly design an end-to-end one-stream CNN to simultaneously learn multi-modality sharable features and map them on a common space. Global loss, part losses and threefold triplet loss are integrated to enhance the feature discriminability and minimize the cross-modality gap. Extensive experimental results on two cross-modality Re-ID datasets exhibit the superiority of the proposed method compared with the state-of-the-art solutions.
更多查看译文
关键词
Adaptive body partition,cross-modality,person re-identification
AI 理解论文
溯源树
样例
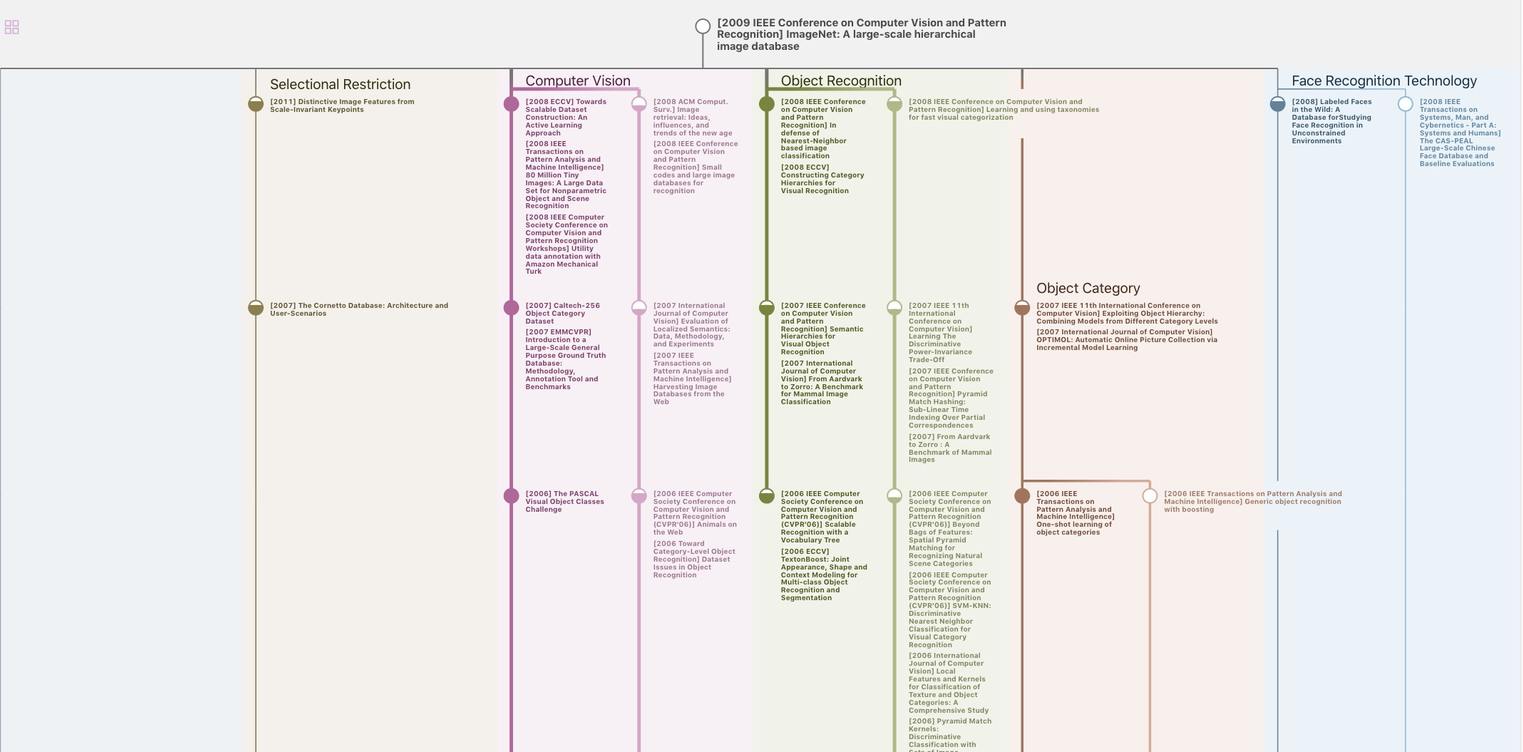
生成溯源树,研究论文发展脉络
Chat Paper
正在生成论文摘要