Particle filtering for chaotic dynamical systems using future right-singular vectors
NONLINEAR DYNAMICS(2020)
摘要
In this paper, we combine tools from the study of chaotic dynamical systems with nonlinear non-Gaussian data assimilation algorithms to produce novel particle filtering algorithms, where the use of dynamical information improves both the accuracy of estimation of the unknown state as well as improves the diversity of the particles that collectively represent the posterior distribution. Unlike past efforts of assimilation in the unstable subspace, we focus not on the deterministic signal and low observation noise case, but instead on the case of moderate signal and observation noise. Filtering algorithms using finite-time Lyapunov vectors, left-singular vectors, and a novel concept of future right-singular vectors, to project observations onto reduced subspaces are developed and tested against two regimes of the single scale Lorenz 1996 model—a weakly chaotic, non-Gaussian regime and a strongly chaotic, near-Gaussian regime. As modeling in the geosciences continue to improve resolution and fidelity of finer physical processes, the models are requiring data assimilation techniques that can handle the fully nonlinear, non-Gaussian case and represent multimodal distributions in high-dimensional spaces. This paper contributes to pushing the boundary of nonlinear data assimilation by aiming to improve particle filtering algorithms for high-dimensional chaotic systems.
更多查看译文
关键词
Data assimilation, Assimilation in the unstable subspace, Particle filters, Optimal proposal, Chaotic dynamics, Right-singular vectors, Lorenz '96 model
AI 理解论文
溯源树
样例
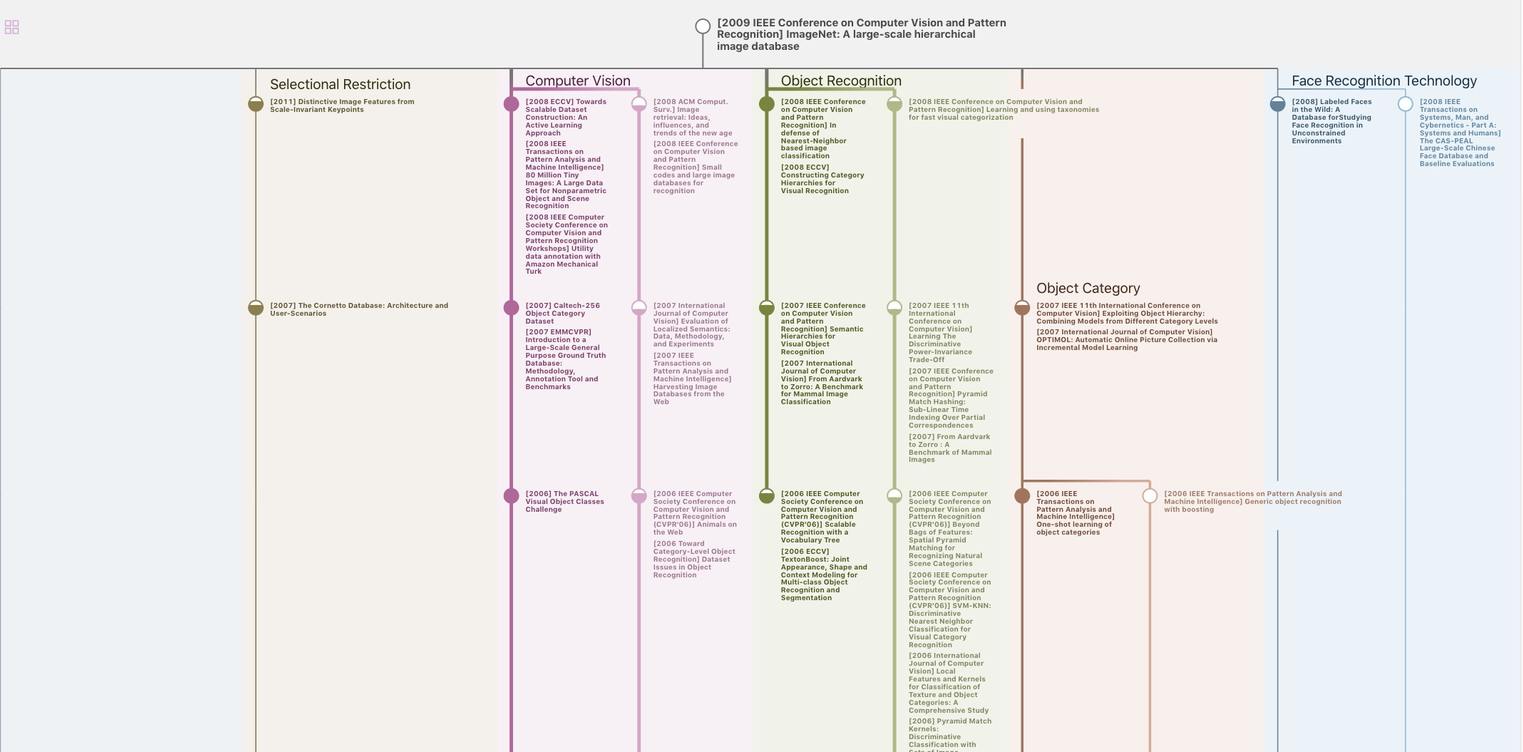
生成溯源树,研究论文发展脉络
Chat Paper
正在生成论文摘要