Topology Discriminative Pooling via Graph Kernel Derived Nodes and Edges Co-Embedding
2020 IEEE International Conference on Multimedia and Expo (ICME)(2020)
摘要
As a vital component of graph neural networks, graph pooling remains largely an open problem. Most existing methods are induced by empirical insights, while ignore the effect of topology information for graph coarsening guidance. In this paper, we propose a Topology Discriminative Pooling (TD-Pool) approach, which derives graph pooling architectures from graph kernels for semantic and structural feature co-embedding. Concretely, TD-Pool contains two graph modules, namely recurrent edge module and convolutional node module, for respectively learning topological/semantic embeddings on edges/nodes. The edge module combines Weisfeiler-Lehman graph kernel with neural networks, which captures topology features in a data-driven manner. It is deployed on the line graphs, whose nodes correspond to the original graph edges for semantic-topology decoupling. The node module learns semantic features with graph convolution in the spectral domain, which acts on the original graphs nodes. Extensive experiments demonstrate the effectiveness of our TD-Pool in graph-level tasks.
更多查看译文
关键词
Graph pooling,edge feature learning
AI 理解论文
溯源树
样例
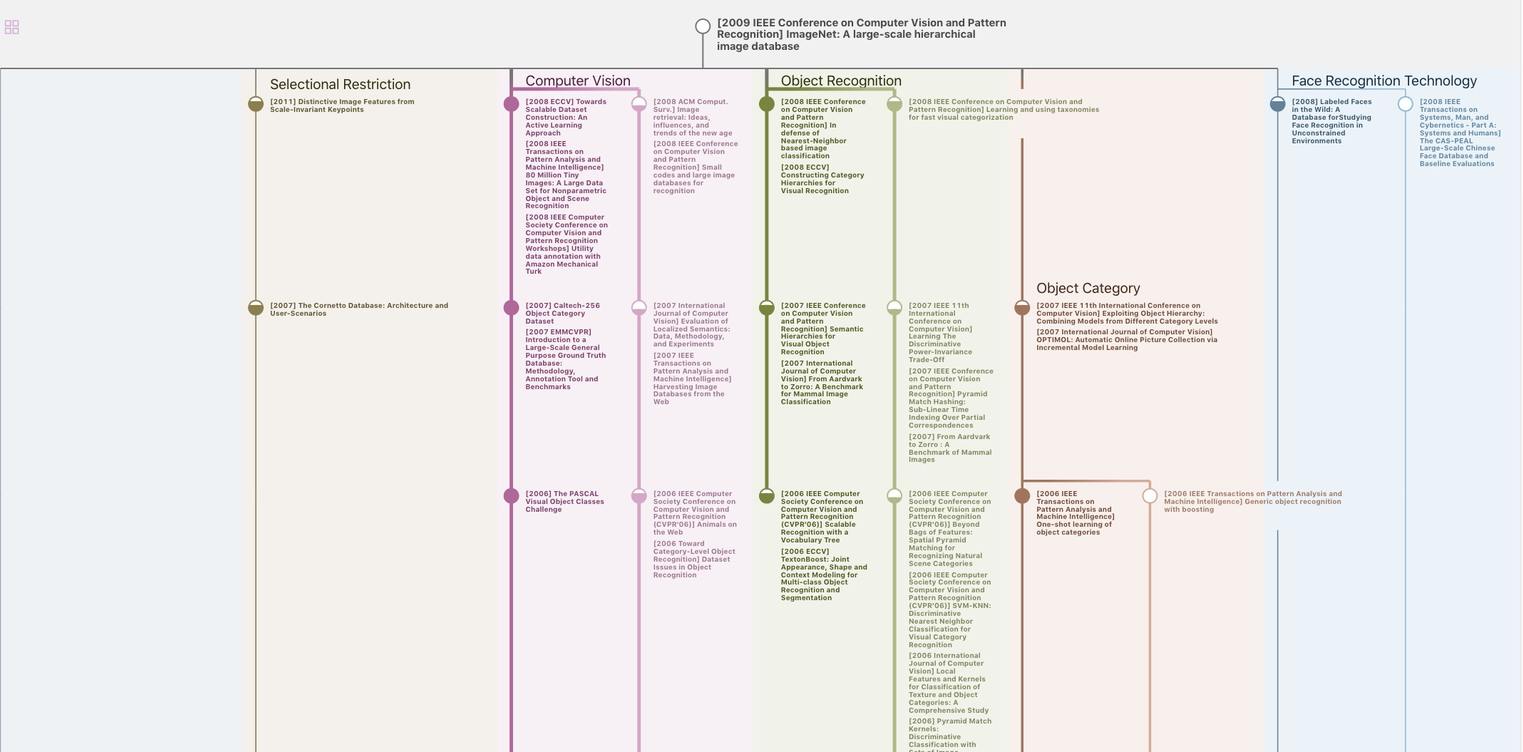
生成溯源树,研究论文发展脉络
Chat Paper
正在生成论文摘要