Topic-Enhanced Capsule Network for Multi-Label Emotion Classification
IEEE-ACM TRANSACTIONS ON AUDIO SPEECH AND LANGUAGE PROCESSING(2020)
摘要
Identifying multiple emotions in a piece of text is an important research topic in the NLP community.Existing methods usually model the task as a multi-label classification problem, while these work has two issues. First, these methods fail to leverage the topic information of the text, which has been shown to be effective for sentiment analysis task. Second, different parts of the text can contribute differently to predicting different emotion labels, so the proposed model needs to capture effective features for each corresponding emotion, which is not considered by existing models. To tackle these problems, we propose a topic-enhanced capsule network, which contains two main parts: a variational autoencoder and a capsule module, for multi-label emotion detection task. Specifically, the variational autoencoder can learn the latent topic information of the text, and the capsule module can capture rich features for corresponding emotion. Experimental results on two benchmark datasets show that the proposed model achieves the current best performance, outperforming previous methods and strong baselines by a large margin.
更多查看译文
关键词
Task analysis,Predictive models,Speech processing,Routing,Feature extraction,Motion pictures,Decoding,Information extraction,emotion detection,neural networks,topic model,sentiment analysis
AI 理解论文
溯源树
样例
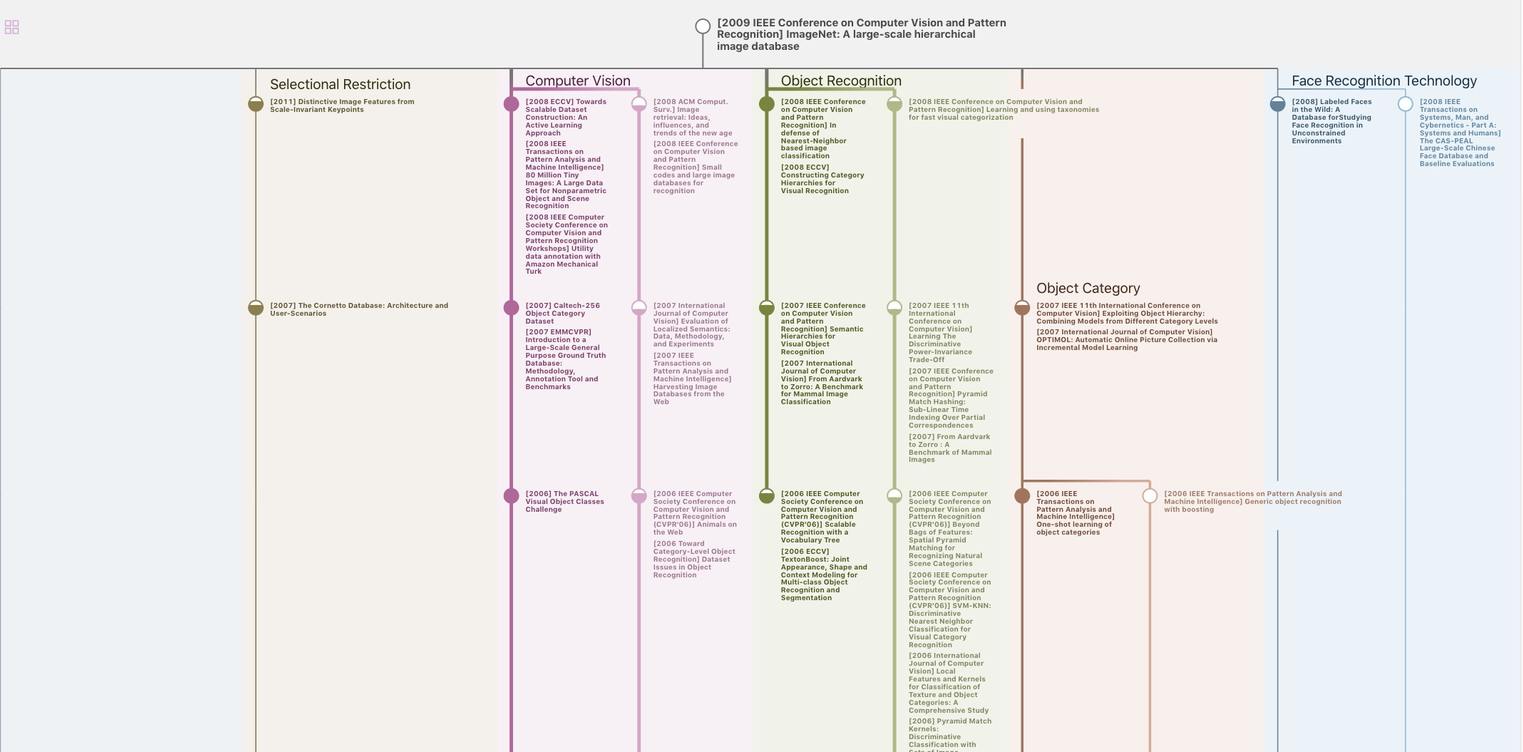
生成溯源树,研究论文发展脉络
Chat Paper
正在生成论文摘要