The Improved Fastmremma And Gcim Algorithms For Genome-Wide Association And Linkage Studies In Large Mapping Populations
CROP JOURNAL(2020)
摘要
Owing to high power and accuracy and low false positive rate in our multi-locus approaches for genome-wide association studies and linkage analyses, these approaches have attracted considerable attention in plant and animal genetics. In large mapping population, however, fast multi-locus random-SNP-effect efficient mixed model association (FASTmrEMMA) and genome-wide composite interval mapping (GCIM) run a relatively long time. To address this issue, we proposed the improved FASTmrEMMA and GCIM algorithms in this study. In the new algorithms, some matrix identities, such as the Woodbury matrix identity, were used. In scanning each marker on the entire genome, in other words, the improved algorithms effectively replace the expensive eigenvector solutions in (restricted) maximum likelihood estimations in original algorithms with two (one) updated inner products and one updated vector-matrix-vector multiplication. Simulated and real data analyses showed that their computational efficiencies are increased sharply in large mapping population, although there are no mapping result differences between original and improved algorithms. In addition, the related software packages (mrMLM.GUI and QTL.gCIMapping.GUI) can be downloaded from the R and BioCode websites. (C) 2020 Crop Science Society of China and Institute of Crop Science, CAAS. Publishing services by Elsevier B.V. on behalf of KeAi Communications Co. Ltd.
更多查看译文
关键词
Genome-wide association study,Genome-wide composite interval mapping,Mixed model,Multi-locus genetic model,Woodbury matrix identity
AI 理解论文
溯源树
样例
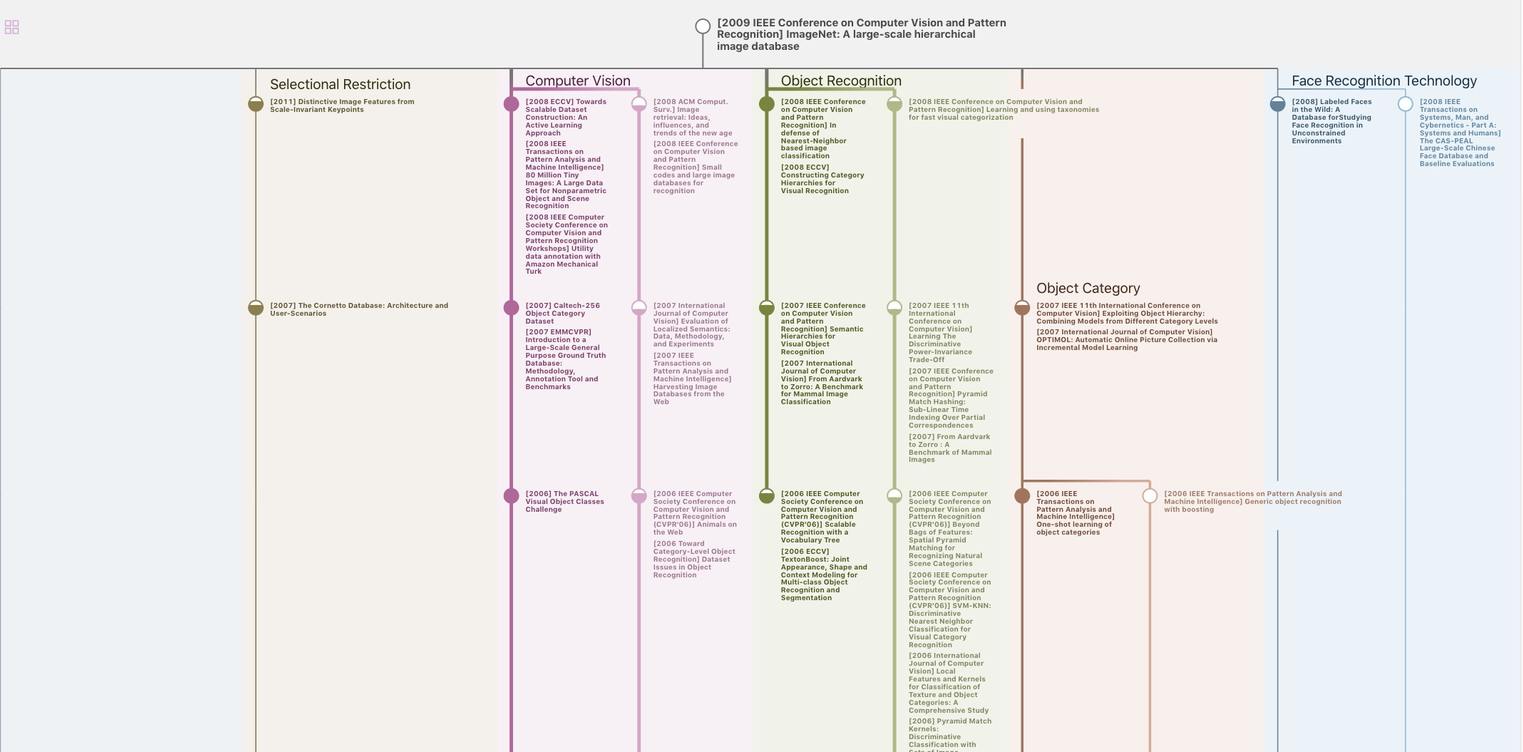
生成溯源树,研究论文发展脉络
Chat Paper
正在生成论文摘要