DesnowGAN: An Efficient Single Image Snow Removal Framework Using Cross-Resolution Lateral Connection and GANs
IEEE Transactions on Circuits and Systems for Video Technology(2021)
摘要
In this paper, we present a simple, efficient, and highly modularized network architecture for single-image snow-removal. To address the challenging snow-removal problem in terms of network interpretability and computational complexity, we employ a pyramidal hierarchical design with lateral connections across different resolutions. This design enables us to incorporate high-level semantic features with other feature maps at different scales to enrich location information and reduce computational time. In addition, a refinement stage based on recently introduced generative adversarial networks (GANs) is proposed to further improve the visual quality of the resulting snow-removed images and make a refined image and a clean image indistinguishable by a computer vision algorithm to avoid the potential perturbations of machine interpretation. Finally, atrous spatial pyramid pooling (ASPP) is adopted to probe features at multiple scales and further boost the performance. The proposed DesnowGAN (DS-GAN) performs significantly better than state-of-the-art methods quantitatively and qualitatively on the Snow100K dataset.
更多查看译文
关键词
Snow,Feature extraction,Network architecture,Generative adversarial networks,Atmospheric modeling,Radio frequency,Computational complexity
AI 理解论文
溯源树
样例
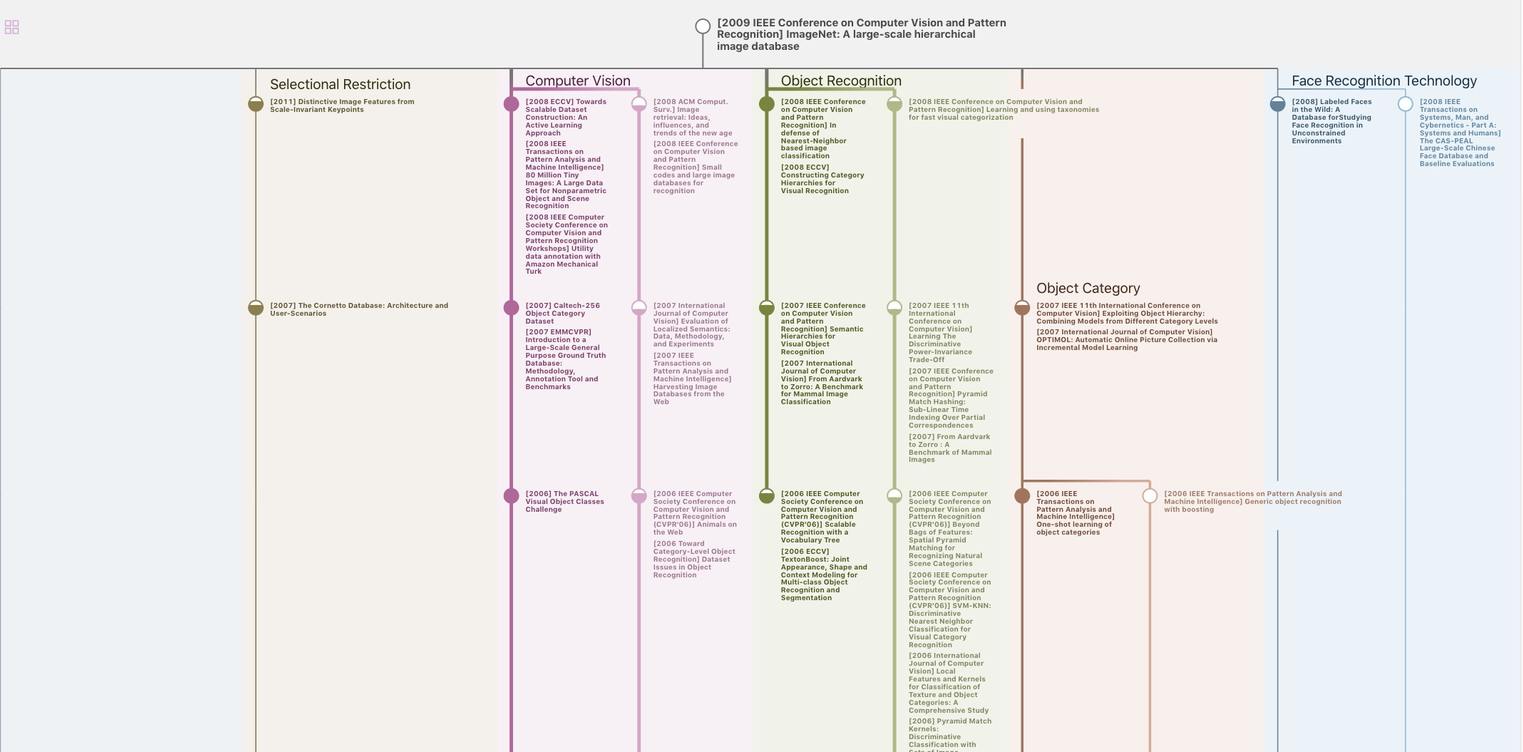
生成溯源树,研究论文发展脉络
Chat Paper
正在生成论文摘要