Fusing imperfect experimental data for risk assessment of musculoskeletal disorders in construction using canonical polyadic decomposition
Automation in Construction(2020)
摘要
Field or laboratory data collected for work-related musculoskeletal disorder (WMSD) risk assessment in construction often becomes unreliable as a large amount of data go missing due to technology-induced errors, instrument failures or sometimes at random. Missing data can adversely affect the assessment conclusions. This study proposes a method that applies Canonical Polyadic Decomposition (CPD) tensor decomposition to fuse multiple sparse risk-related datasets and fill in missing data by leveraging the correlation among multiple risk indicators within those datasets. Two knee WMSD risk-related datasets—3D knee rotation (kinematics) and electromyography (EMG) of five knee postural muscles—collected from previous studies were used for the validation and demonstration of the proposed method. The analysis results revealed that for a large portion of missing values (40%), the proposed method can generate a fused dataset that provides reliable risk assessment results highly consistent (70%–87%) with those obtained from the original experimental datasets. This signified the usefulness of the proposed method for use in WMSD risk assessment studies when data collection is affected by a significant amount of missing data, which will facilitate reliable assessment of WMSD risks among construction workers. In the future, findings of this study will be implemented to explore whether, and to what extent, the fused dataset outperforms the datasets with missing values by comparing consistencies of the risk assessment results obtained from these datasets for further investigation of the fusion performance.
更多查看译文
关键词
Tensor decomposition,Risk assessment,Data fusion,Construction safety
AI 理解论文
溯源树
样例
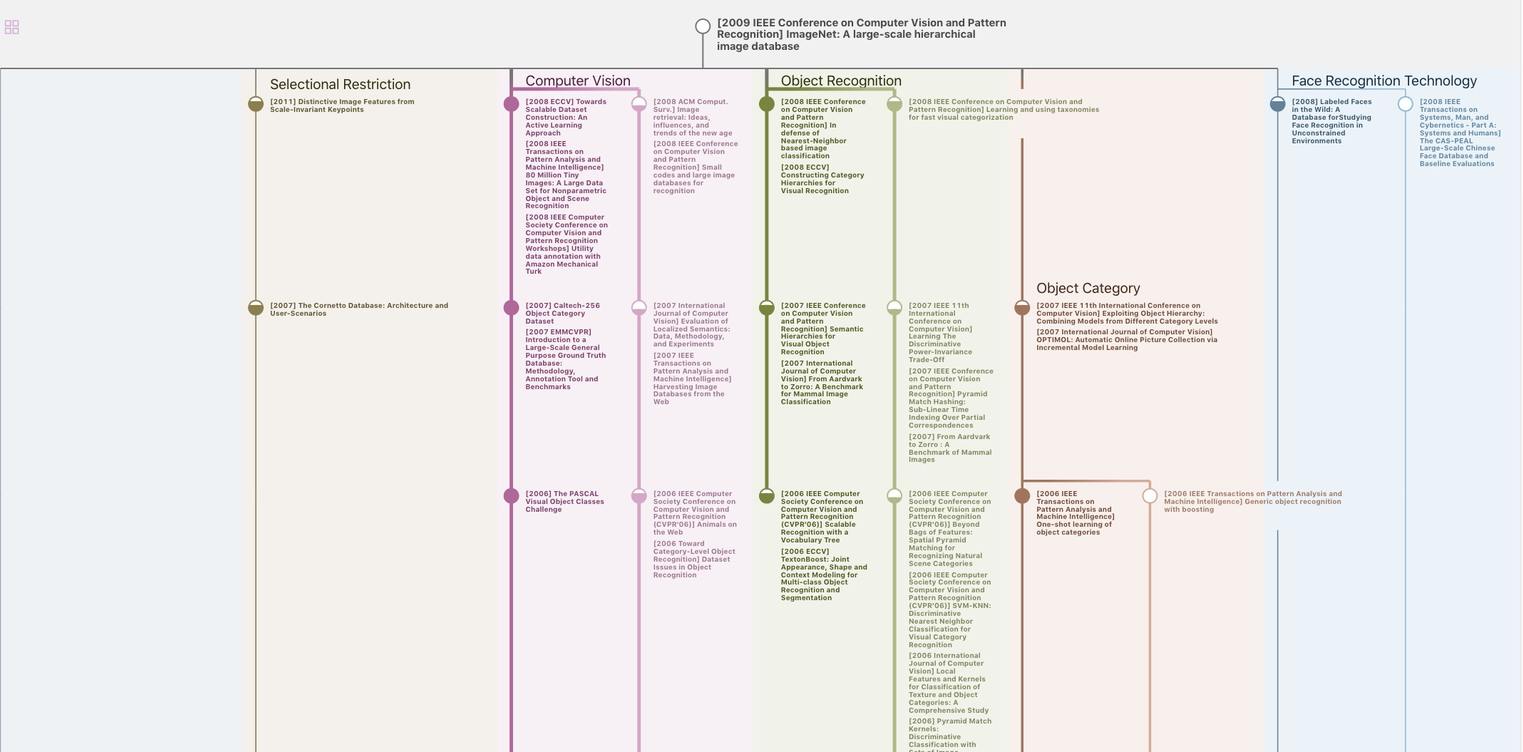
生成溯源树,研究论文发展脉络
Chat Paper
正在生成论文摘要