Benchmark for Generic Product Detection: A Low Data Baseline for Dense Object Detection.
international conference on image analysis and recognition(2020)
摘要
Object detection in densely packed scenes is a new area where standard object detectors fail to train well [6]. Dense object detectors like RetinaNet [7] trained on large and dense datasets show great performance. We train a standard object detector on a small, normally packed dataset with data augmentation techniques. This dataset is 265 times smaller than the standard dataset, in terms of number of annotations. This low data baseline achieves satisfactory results (mAP = 0.56) at standard IoU of 0.5. We also create a varied benchmark for generic SKU product detection by providing full annotations for multiple public datasets. It can be accessed at this URL. We hope that this benchmark helps in building robust detectors that perform reliably across different settings in the wild.
更多查看译文
关键词
Dense object detection, Grocery products, Retail products, Benchmark, Generic SKU detection
AI 理解论文
溯源树
样例
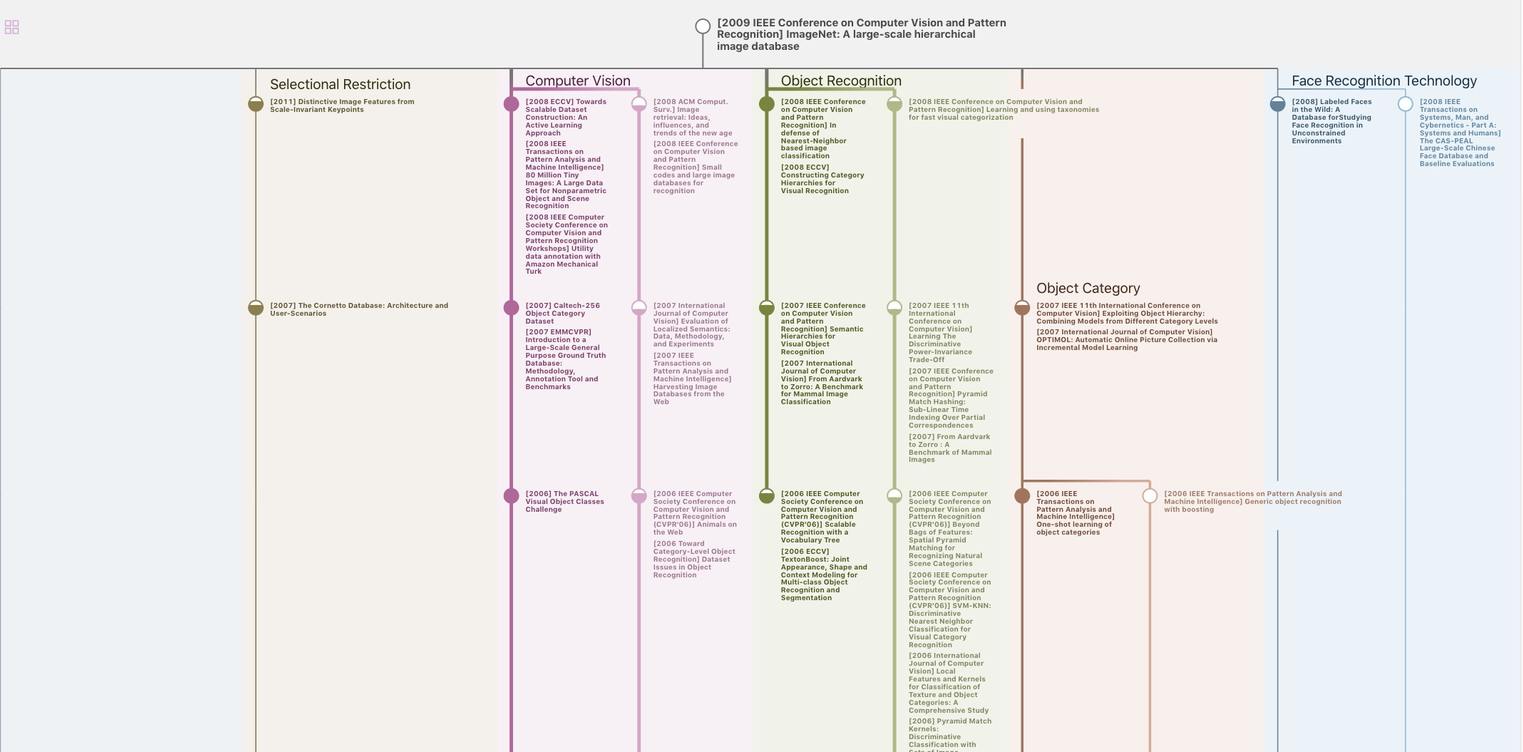
生成溯源树,研究论文发展脉络
Chat Paper
正在生成论文摘要