Robust Deep Reservoir Computing Through Reliable Memristor With Improved Heat Dissipation Capability
IEEE Transactions on Computer-Aided Design of Integrated Circuits and Systems(2021)
摘要
Deep neural networks (DNNs), a brain-inspired learning methodology, requires tremendous data for training before performing inference tasks. The recent studies demonstrate a strong positive correlation between the inference accuracy and the size of the DNNs and datasets, which leads to an inevitable demand for large DNNs. However, conventional memory techniques are not adequate to deal with the drastic growth of dataset and neural network size. Recently, a resistive memristor has been widely considered as the next generation memory device owing to its high density and low power consumption. Nevertheless, its high switching resistance variations (cycle-to-cycle) restrict its feasibility in deep learning. In this work, a novel memristor configuration with the enhanced heat dissipation feature is fabricated and evaluated to address this challenge. Our experimental results demonstrate our memristor reduces the resistance variation by ~ 30% and the inference accuracy increases correspondingly in a similar range. The accuracy increment is evaluated by our deep delay-feed-back reservoir computing (Deep-DFR) model. The design area, power consumption, and latency are reduced by ~48%, ~42%, and ~67%, respectively, compared to the conventional static random-access memory technique (6T). The performance of our memristor is improved at various degrees (~13%-73%) compared to the state-of-the-art memristors.
更多查看译文
关键词
Artificial neural networks,deep delay-feed-back reservoir computing (Deep-DFR),memristor,reservoir computing
AI 理解论文
溯源树
样例
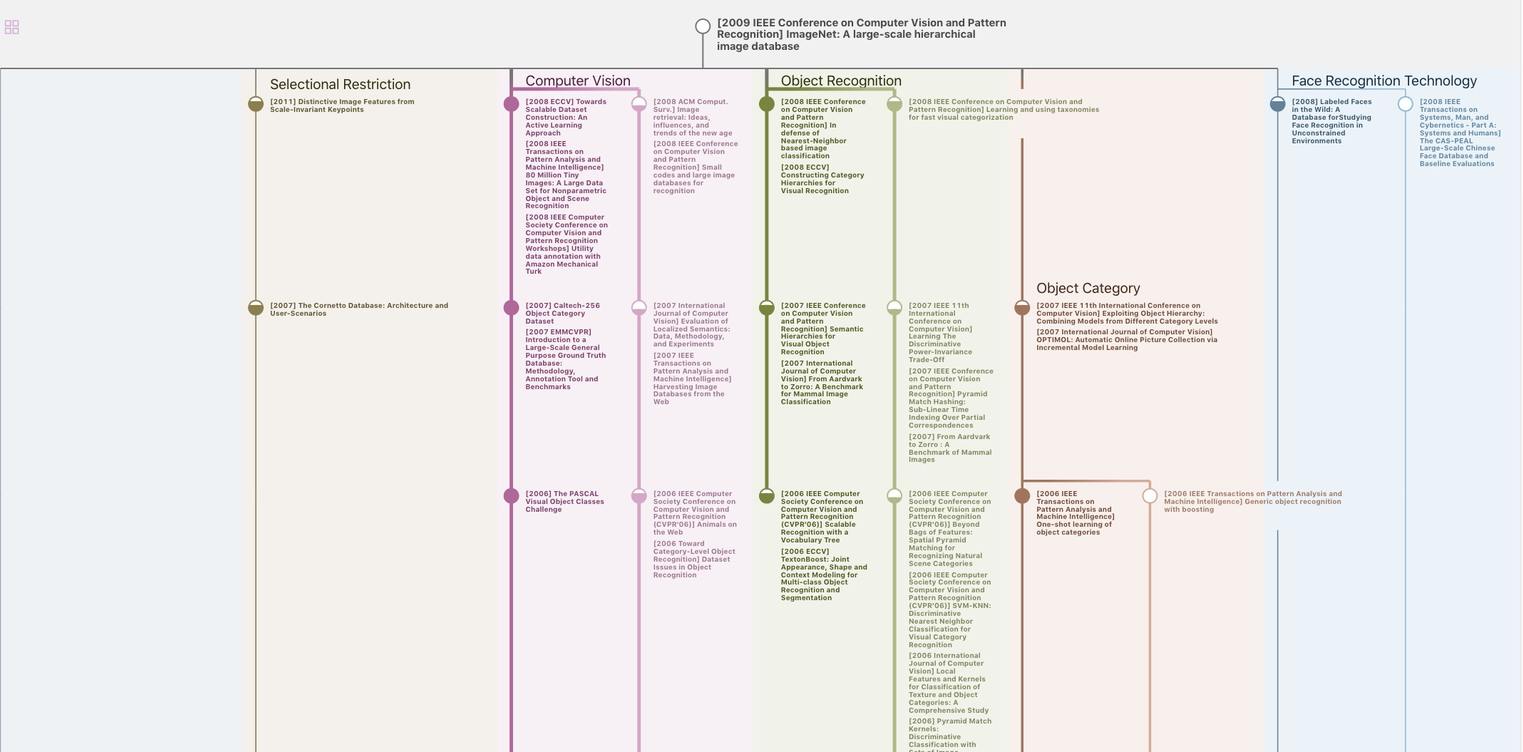
生成溯源树,研究论文发展脉络
Chat Paper
正在生成论文摘要