General Transportability - Synthesizing Observations And Experiments From Heterogeneous Domains
national conference on artificial intelligence(2020)
摘要
The process of transporting and synthesizing experimental findings from heterogeneous data collections to construct causal explanations is arguably one of the most central and challenging problems in modern data science. This problem has been studied in the causal inference literature under the rubric of causal effect identifiability and transportability (Bareinboim and Pearl 2016). In this paper, we investigate a general version of this challenge where the goal is to learn conditional causal effects from an arbitrary combination of datasets collected under different conditions, observational or experimental, and from heterogeneous populations. Specifically, we introduce a unified graphical criterion that characterizes the conditions under which conditional causal effects can be uniquely determined from the disparate data collections. We further develop an efficient, sound, and complete algorithm that outputs an expression for the conditional effect whenever it exists, which synthesizes the available causal knowledge and empirical evidence; if the algorithm is unable to find a formula, then such synthesis is provably impossible, unless further parametric assumptions are made. Finally, we prove that do-calculus (Pearl 1995) is complete for this task, i.e., the inexistence of a do-calculus derivation implies the impossibility of constructing the targeted causal explanation.
更多查看译文
AI 理解论文
溯源树
样例
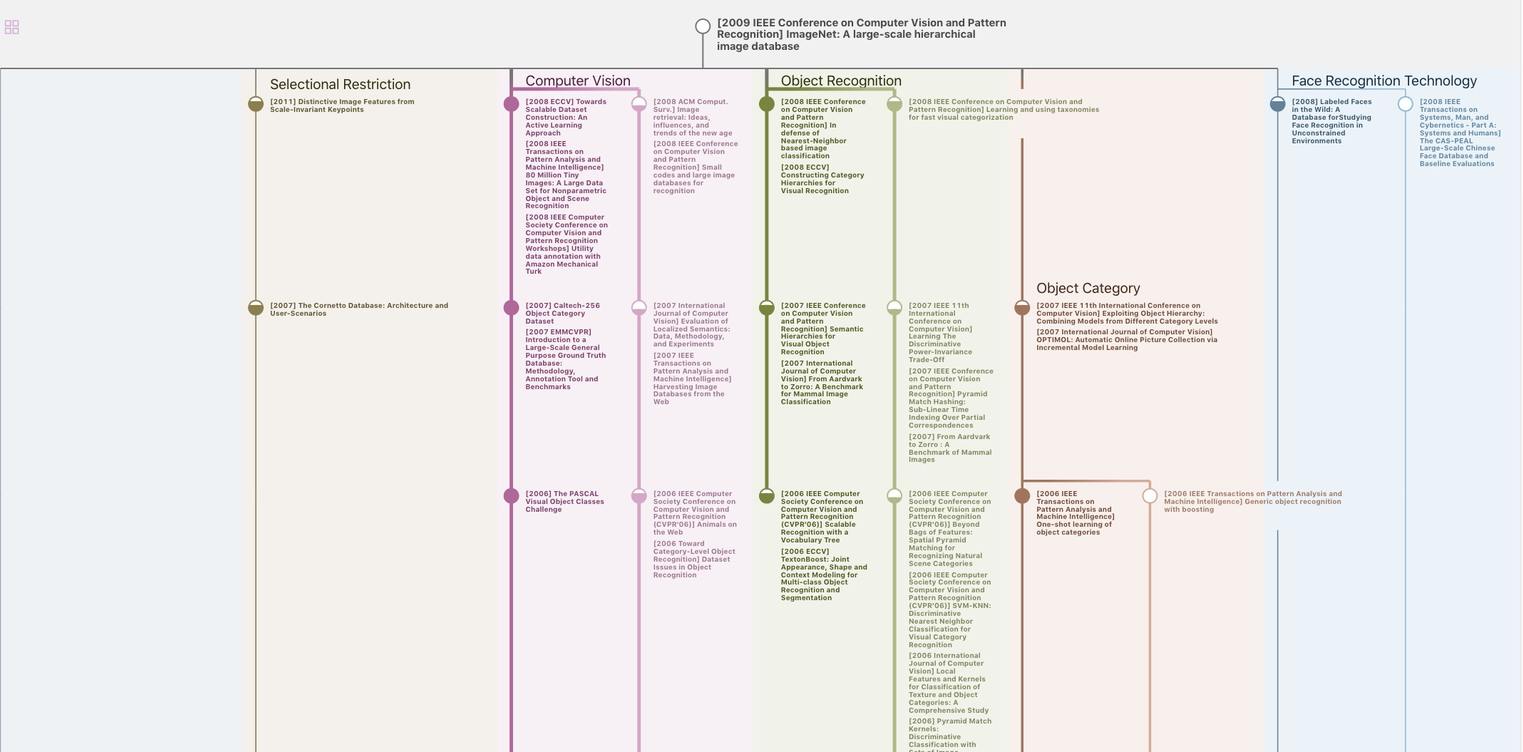
生成溯源树,研究论文发展脉络
Chat Paper
正在生成论文摘要