Machine Learning to Identify Variables in Thermodynamically Small Systems
Computers & Chemical Engineering(2020)
摘要
Thermodynamically small systems, with a number N of interacting particles in the range of 1–1000, are increasingly of interest in science and engineering. While the thermodynamic formalism for bulk systems, where N approaches infinity, was established long ago, the thermodynamics of small systems is currently approached by adding new variables in a somewhat ad hoc fashion. We propose a more rigorous approach based on machine learning (ML), which we demonstrate by applying both unsupervised (diffusion maps, autoencoders) and supervised (classical neural networks) ML methods to large data sets from Monte Carlo simulations of systems comprising N=3 Lennard-Jones particles at fixed temperature. The ML methods clearly identify structural and energetic changes that occur in this model system and suggest that the data may be collapsed from the original nine dimensions to two. Using intuition and screening, we identified two simple geometric properties of the system as a useful variable set.
更多查看译文
关键词
Artificial neural networks,Diffusion maps,Molecular simulation,Clusters
AI 理解论文
溯源树
样例
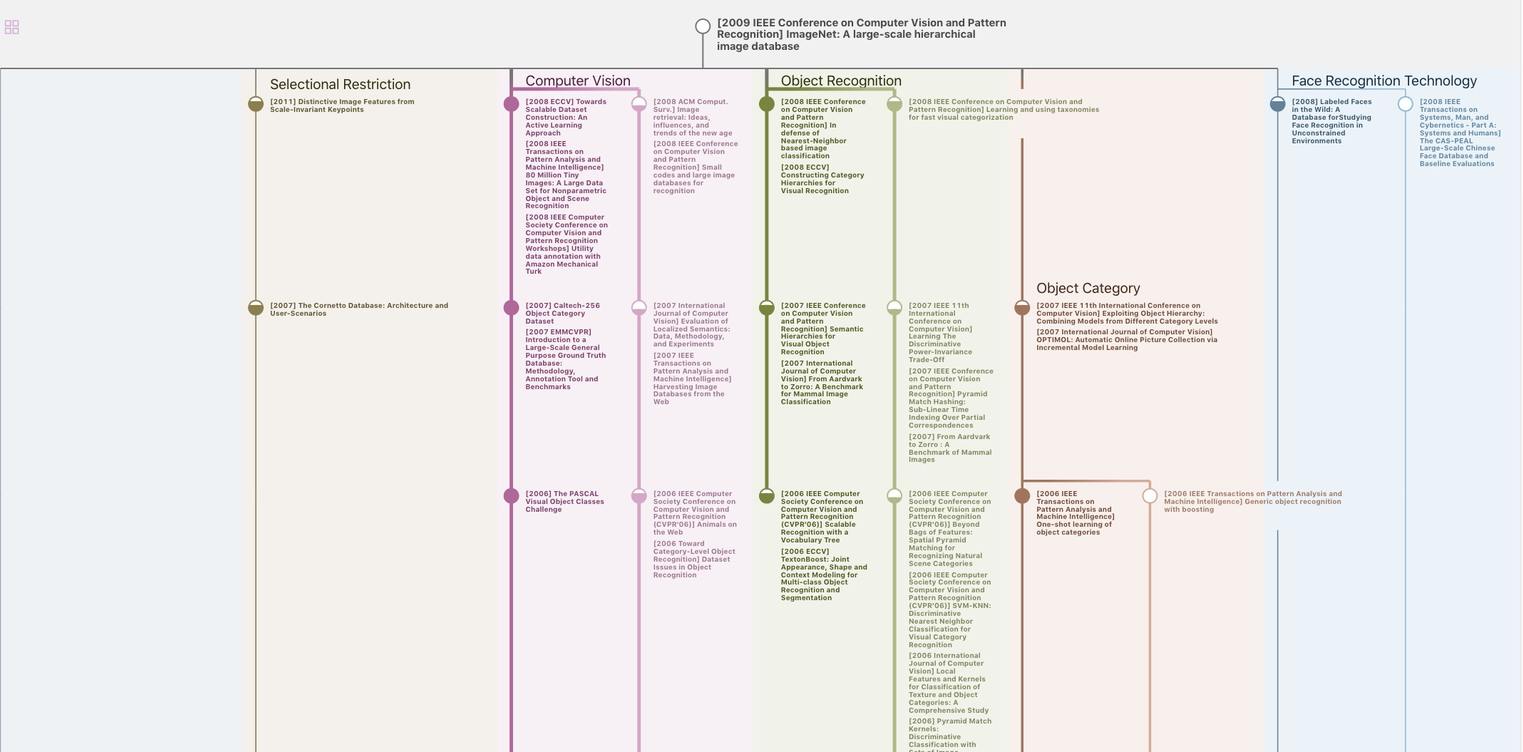
生成溯源树,研究论文发展脉络
Chat Paper
正在生成论文摘要