Incremental Learning of Multi-Domain Image-to-Image Translations
IEEE Transactions on Circuits and Systems for Video Technology(2021)
摘要
Current multi-domain image-to-image translation models assume a fixed set of domains and that all the data are always available during training. However, over time, we may want to include additional domains to our model. Existing methods either require re-training the whole model with data from all domains or require training several additional modules to accommodate new domains. To address these limitations, we present IncrementalGAN, a multi-domain image-to-image translation model that can incrementally learn new domains using only a single generator. Our approach first decouples the domain label representation from the generator to allow it to be re-used for new domains without any architectural modification. Next, we introduce a distillation loss that prevents the model from forgetting previously learned domains. Our model compares favorably against several state-of-the-art baselines.
更多查看译文
关键词
Generators,Data models,Generative adversarial networks,Training,Training data,Gallium nitride,Task analysis
AI 理解论文
溯源树
样例
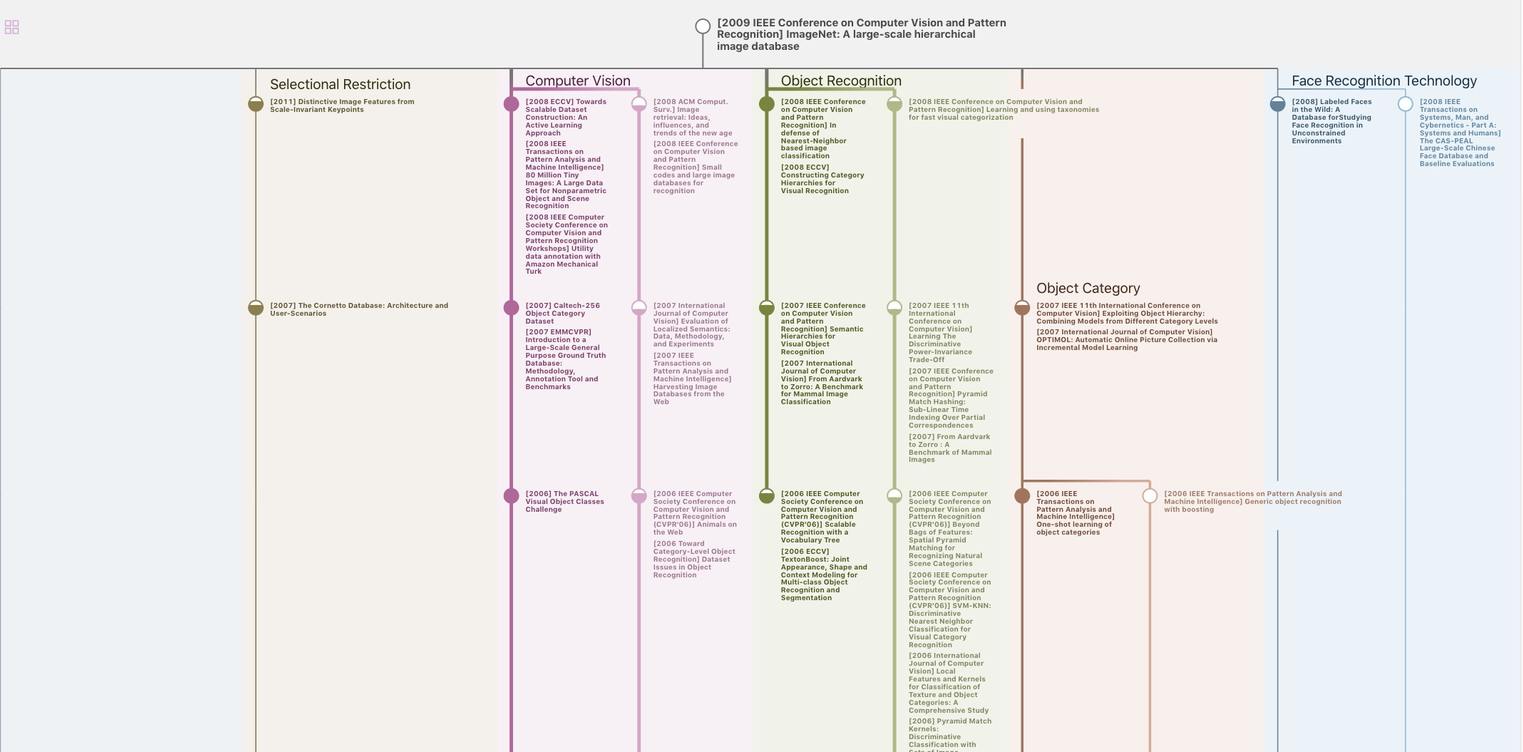
生成溯源树,研究论文发展脉络
Chat Paper
正在生成论文摘要