Learning Feature Representation And Partial Correlation For Multimodal Multi-Label Data
IEEE TRANSACTIONS ON MULTIMEDIA(2021)
摘要
User-provided annotations in existing multimodal datasets sometimes are inappropriate for model learning and can hinder the task of cross-modal retrieval. To handle this issue, we propose a discriminative and noise-robust cross-modal retrieval method, called FLPCL, which consists of deep feature learning and partial correlation learning. Deep feature learning is implemented by utilizing label supervised information to guide the training of deep neural network for each modality, which aims to find modality-specific deep feature representations that preserve the similarity and discrimination information among multimodal data. Based on deep feature learning, partial correlation learning is proposed to infer direct association between different modalities by removing the effect of common underlying semantics from each modality. It is achieved by maximizing the canonical correlation of the feature representations of different modalities conditioned on the label modality. Different from existing works that build indirect association between modalities via incorporating semantic labels, our FLPCL method can learn more effective and robust multimodal latent representations by explicitly preserving both intra-modal and inter-modal relationship among multimodal data. Extensive experiments on three cross-modal datasets show that our method outperforms state-of-the-art methods on cross-modal retrieval tasks.
更多查看译文
关键词
Semantics, Correlation, Task analysis, Data models, Learning systems, Kernel, Deep learning, Cross-modal retrieval, correlation learning, feature learning, partial correlation
AI 理解论文
溯源树
样例
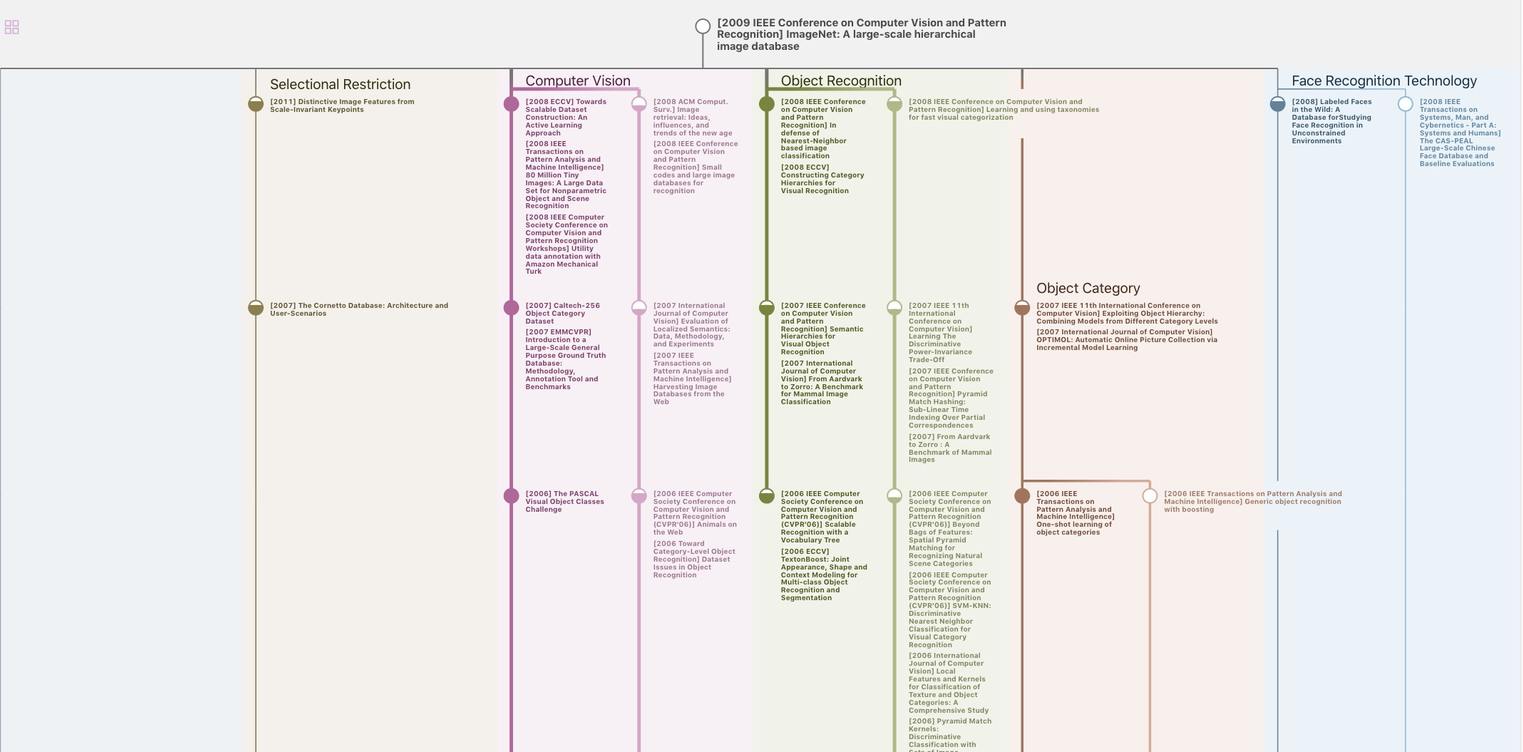
生成溯源树,研究论文发展脉络
Chat Paper
正在生成论文摘要