Revisit Retinex Theory: Towards a Lightness-Aware Restorer for Underexposed Images
MATHEMATICAL PROBLEMS IN ENGINEERING(2020)
摘要
We investigate how to correct exposure of underexposed images. The bottleneck of previous methods mainly lies in their naturalness and robustness when dealing with images with various exposure levels. When facing well-exposed or extremely underexposed images, they may produce over- or underenhanced outputs. In this paper, we propose a novel retinex-based approach, namely, LiAR (short for lightness-aware restorer). The word "lightness-aware" refers to that the estimated illumination not only is a component to be adjusted but also serves as a measure that reflects the brightness of the scene, determining the degree of adjustment. In this way, underexposed images can be restored adaptively according to their own brightness. Given an image, LiAR first estimates its illumination map using a specially designed loss function which can ensure the result's color consistency and texture richness. Then adaptive correction is performed to get properly exposed output. LiAR is based on internal optimization of the single test image and does not need any prior training, implying that it can adapt itself to different settings per image. Additionally, LiAR can be easily extended to the video case due to its simplicity and stability. Experiments demonstrate that facing images/videos with various exposure levels, LiAR can achieve robust and real-time correction with high contrast and naturalness.
更多查看译文
AI 理解论文
溯源树
样例
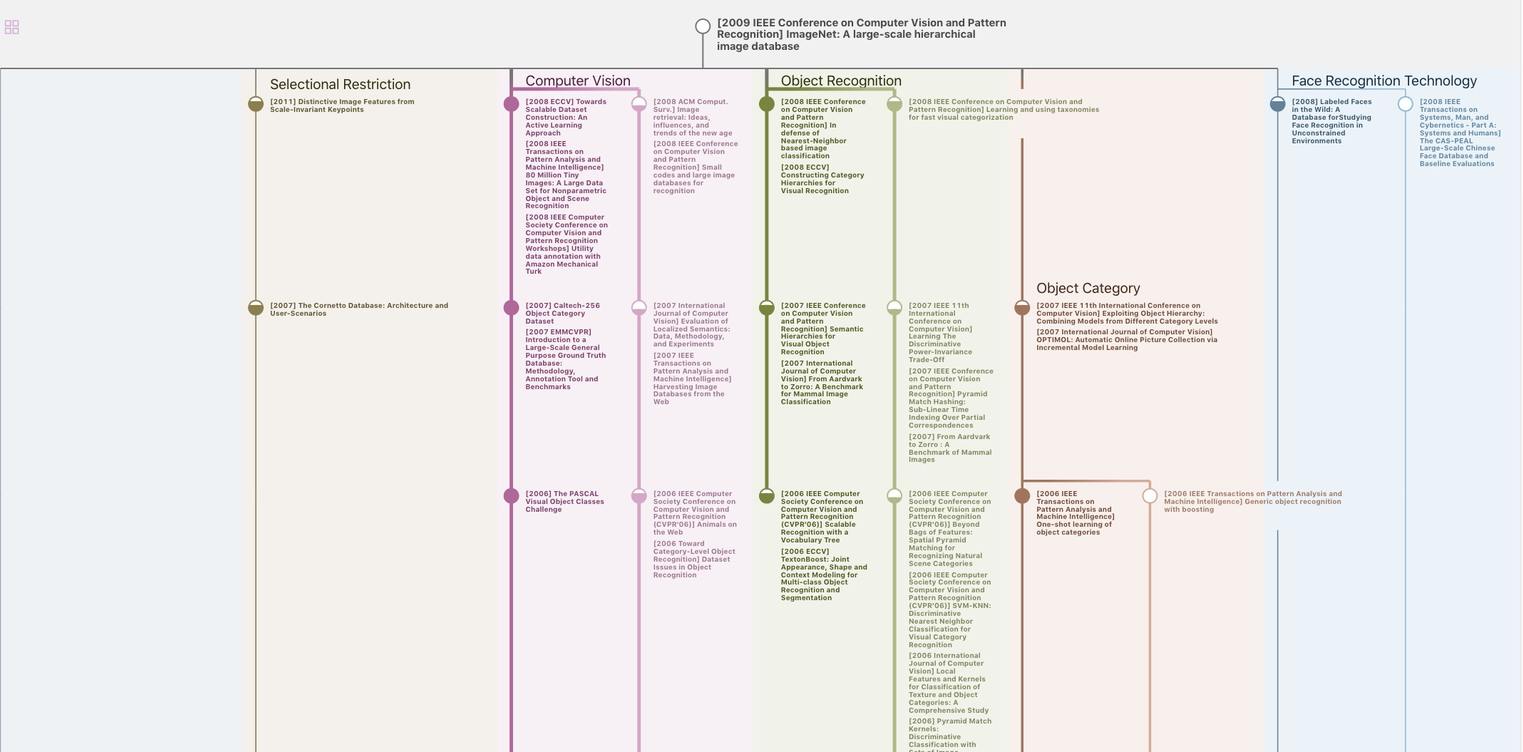
生成溯源树,研究论文发展脉络
Chat Paper
正在生成论文摘要