Dynamic Visualization of Uncertainties in Medical Feature of Interest
IEEE ACCESS(2020)
摘要
In a medical context, uncertainty visualization of a Feature of Interest (FOI) is important, which could provide medical experts with informative feedback to make better decisions for diagnosis or preoperative planning. Traditional uncertainty visualization techniques allow the exploration of the uncertainty visualization of the FOI. However, when the intensity of the FOI and other materials is similar or overlapping, and/or the FOI is occluded by other materials, it would be difficult or impossible for them to reveal the FOI and its uncertainties. To address this problem, we propose an uncertainty visualization technique that includes two main components: the Multiattribute-rule-based FOI Segmentation (M-rule Seg. for short) and the FOI Dynamic Uncertainty Visualization. To demonstrate its effectiveness, we performed an experiment comparing it with two traditional uncertainty visualization techniques. The experimental results show that our proposed uncertainty visualization technique allows to better reveal the FOI and all its uncertainties that are difficult or impossible to be visualized by traditional uncertainty visualization techniques. In addition, we performed another experiment to compare our proposed M-rule Seg. technique with three traditional segmentation techniques. The experimental results show that our M-rule Seg. technique can generate more accurate segmentation results at the cost of a longer computing time.
更多查看译文
关键词
Medical visualization,multiattribute-rule,uncertainty visualization,volume segmentation
AI 理解论文
溯源树
样例
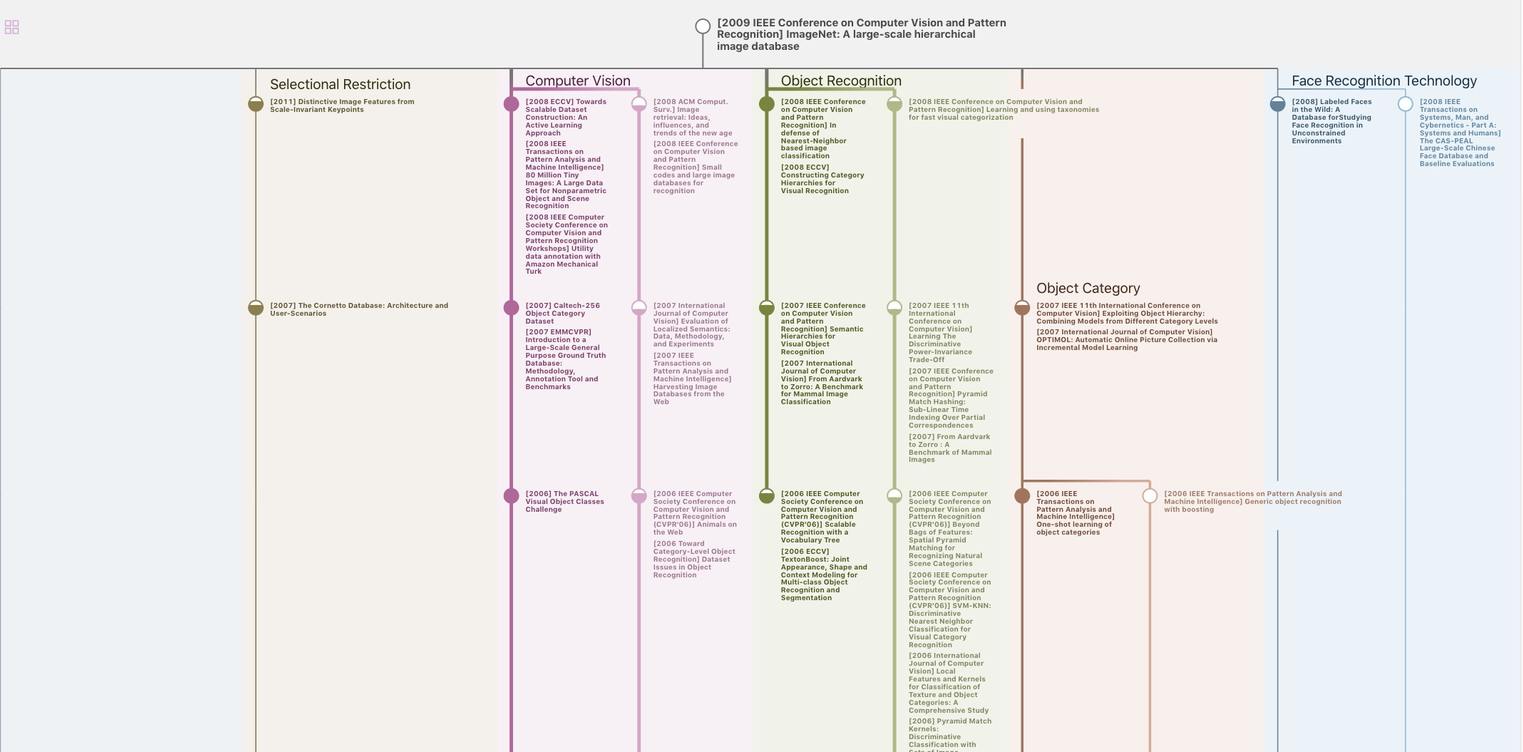
生成溯源树,研究论文发展脉络
Chat Paper
正在生成论文摘要