Modelling desert locust presences using 32-year soil moisture data on a large-scale
ECOLOGICAL INDICATORS(2020)
摘要
The desert locust is the world's most dangerous migratory pest according to the Food and Agriculture Organization of the United Nations (FAO), and its recession area extends over more than 30 countries. While promising assertions have been made to relate desert locust presences with remotely sensed soil moisture (SM), they have not yet been tested robustly in large-scale studies. The aim of this work was to evaluate the potential of soil moisture data to detect desert locust presences (solitarious nymphs) using the European Space Agency Climate Change Initiative Soil Moisture (ESA CCI SM) product from 1985 to 2017. Firstly, 5 machine learning algorithms were fitted using various pre-processing options and variable creation scenarios. Subsequently, the best performing models were fine-tuned using the k-fold cross validation technique and validate the results with an independent dataset taking random dates and locations. The best results were obtained by the weighted k-nearest neighbours (kknn) and random forest (rf) models. The kknn performance was ROC-AUC = 0.79, KAPPA = 0.61 and accuracy = 0.83; and the rf obtained a ROC-AUC = 0.91, KAPPA = 0.56 and accu-racy = 0.81. In general, both models agreed that SM values above 0.11 m(3)/m(3) led to increase the possibility to observe nymph of desert locust with a time delay between 35 and 79 days depending on the model. Furthermore, it was found that model performances increased when the time interval of the variables was smaller, so that we suggest the use of mean SM values over 4 days period to link presences and SM values. These results prove the validity of our methodology to identify favourable breeding areas by means of ESA CCI SM dataset using ma-chine learning approaches over the entire recession area of desert locust, and it could be used in any of the affected countries for this pest. Future improvements in ESA CCI SM product (e.g. higher spatial resolution) may lead to improve model accuracies with monitoring and preventive purposes.
更多查看译文
关键词
ESA CCI SM,Desert locust,Machine learning,Monitoring and preventive,Soil moisture
AI 理解论文
溯源树
样例
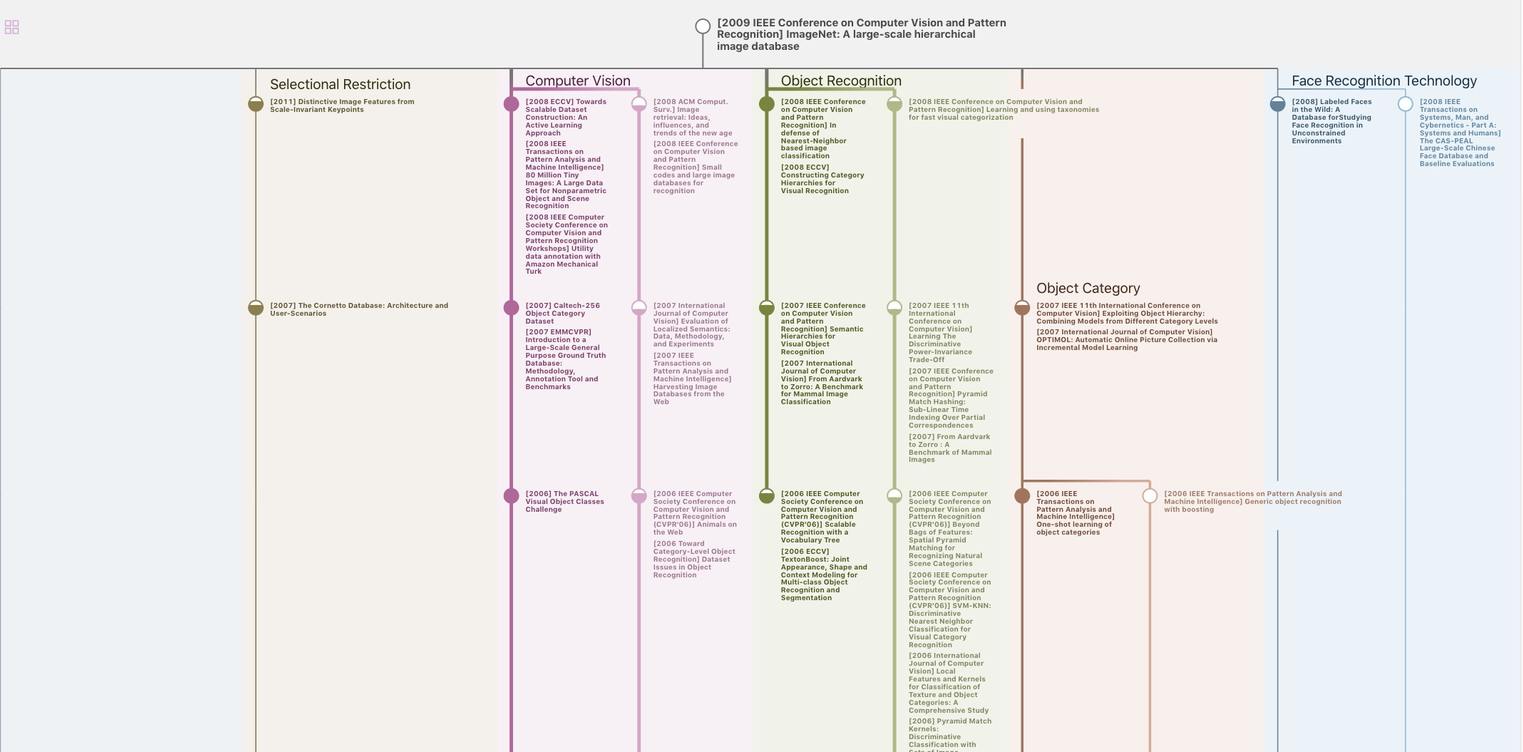
生成溯源树,研究论文发展脉络
Chat Paper
正在生成论文摘要