Algorithmic Improvement of Crowdsourced Data: Intrinsic Quality Measures, Local Optima, and Consensus
SIGSPATIAL '20: 28th International Conference on Advances in Geographic Information Systems Seattle WA USA November, 2020(2020)
摘要
Raw crowdsourced data is often of questionable quality. The typical solution to this is redundancy: ask multiple independent participants the same question and take some form of majority answer. However, this can be wasteful in terms of human effort. In this paper we show that algorithmic analysis of the data is able to get higher quality results out of a given amount of crowd effort (or alternatively, that less crowd effort would have sufficed for the same level of quality). Our case study is based on a publicly available crowdsourced data set by the New York Public Library, featuring building footprints in historical insurance atlases. Besides evaluating the quality improvement achieved by our methods, we provide both a command line interface for batch-mode processing and an interactive web interface; both work with standard data formats and are available as open source software.
更多查看译文
关键词
crowdsourced data,improvement
AI 理解论文
溯源树
样例
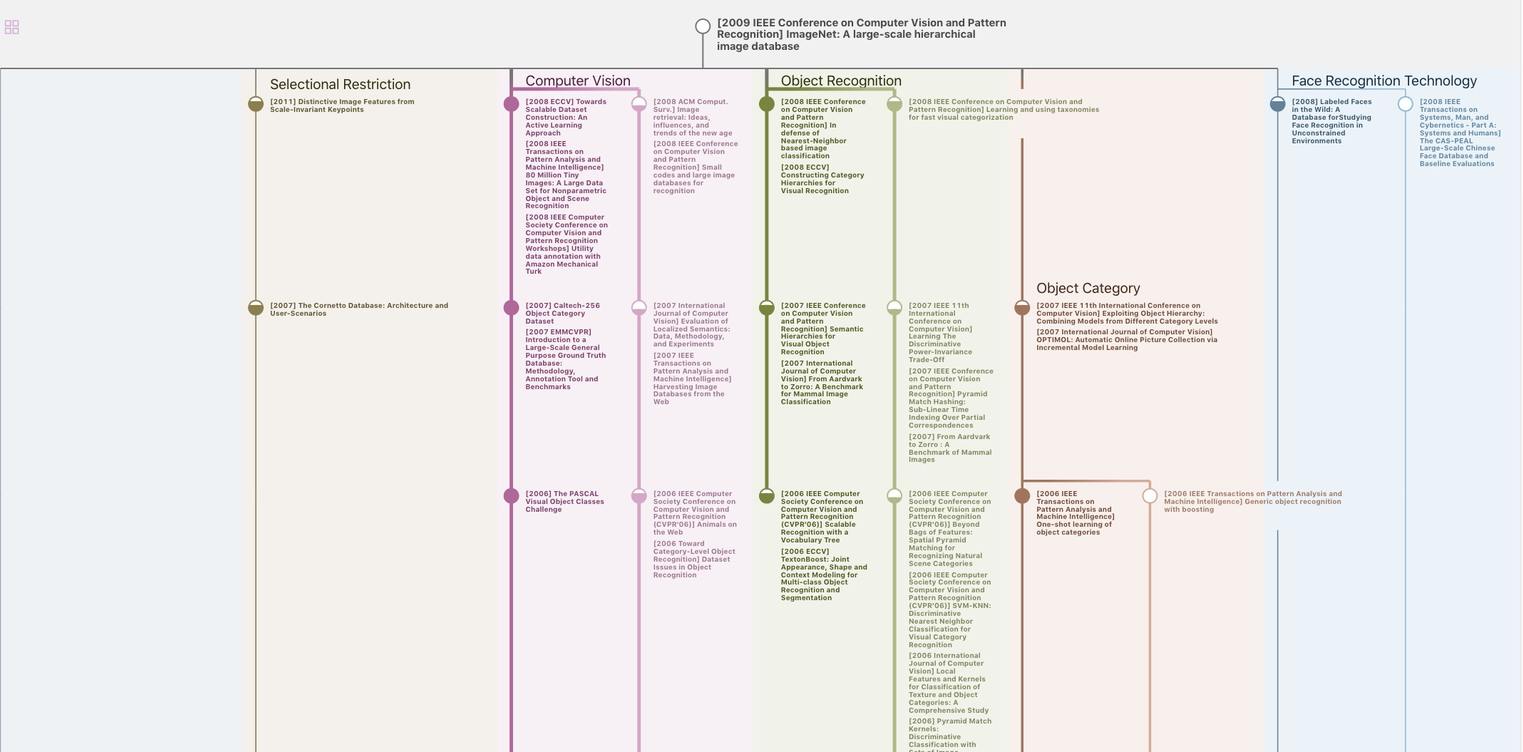
生成溯源树,研究论文发展脉络
Chat Paper
正在生成论文摘要