Adversarial Multi-Label Variational Hashing
Periodicals(2021)
摘要
AbstractIn this paper, we propose an adversarial multi-label variational hashing (AMVH) method to learn compact binary codes for efficient image retrieval. Unlike most existing deep hashing methods which only learn binary codes from specific real samples, our AMVH learns hash functions from both synthetic and real data which make our model effective for unseen data. Specifically, we design an end-to-end deep hashing framework which consists of a generator network and a discriminator-hashing network by enforcing simultaneous adversarial learning and discriminative binary codes learning to learn compact binary codes. The discriminator-hashing network learns binary codes by optimizing a multi-label discriminative criterion and minimizing the quantization loss between binary codes and real-value codes. The generator network is learned so that latent representations can be sampled in a probabilistic manner and used to generate new synthetic training sample for the discriminator-hashing network. Experimental results on several benchmark datasets show the efficacy of the proposed approach.
更多查看译文
关键词
Binary codes, Training, Semantics, Generators, Image retrieval, Hash functions, Visualization, Scalable image search, fast similarity search, hashing, deep learning, multi-label learning
AI 理解论文
溯源树
样例
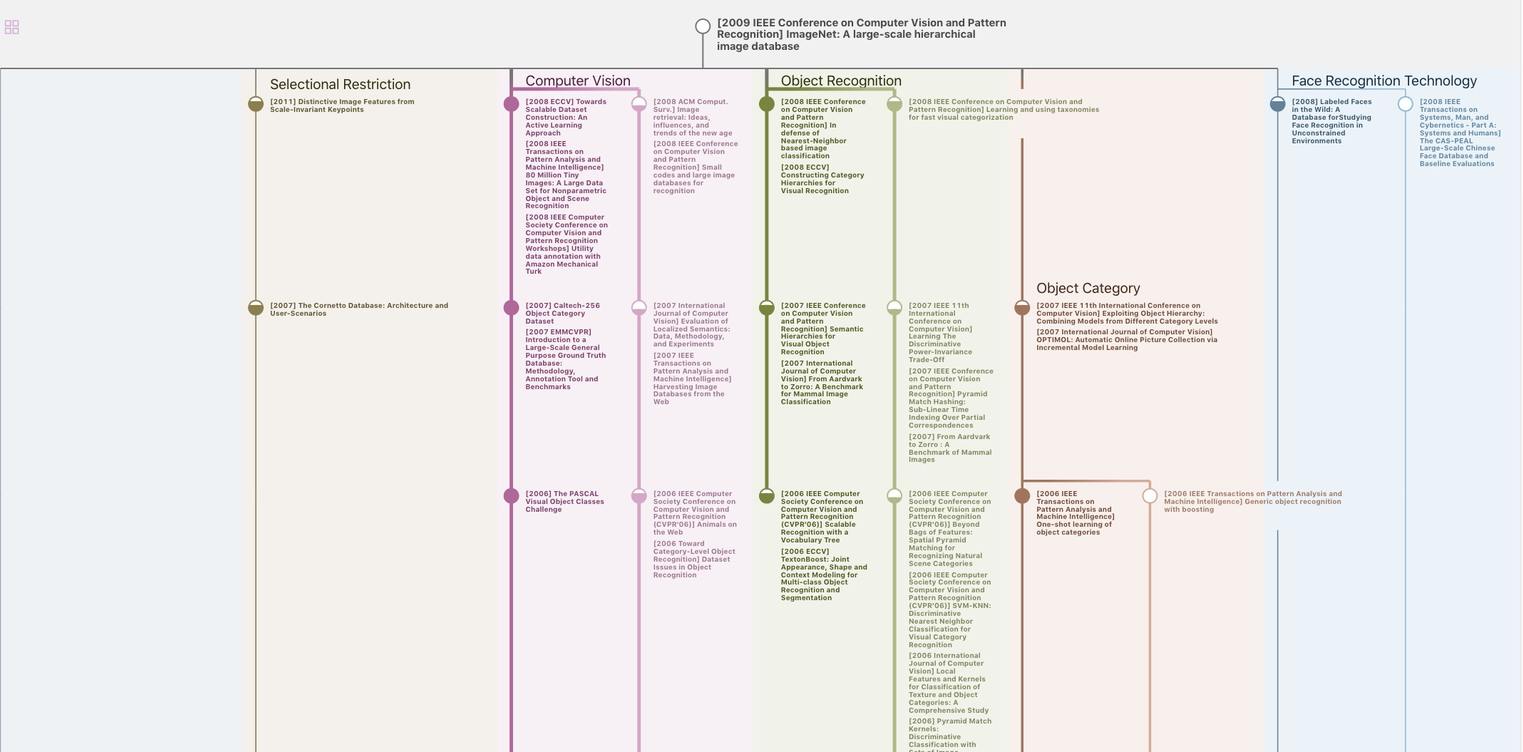
生成溯源树,研究论文发展脉络
Chat Paper
正在生成论文摘要