Multi-layered tensor networks for image classification
arxiv(2020)
摘要
The recently introduced locally orderless tensor network (LoTeNet) for supervised image classification uses matrix product state (MPS) operations on grids of transformed image patches. The resulting patch representations are combined back together into the image space and aggregated hierarchically using multiple MPS blocks per layer to obtain the final decision rules. In this work, we propose a non-patch based modification to LoTeNet that performs one MPS operation per layer, instead of several patch-level operations. The spatial information in the input images to MPS blocks at each layer is squeezed into the feature dimension, similar to LoTeNet, to maximise retained spatial correlation between pixels when images are flattened into 1D vectors. The proposed multi-layered tensor network (MLTN) is capable of learning linear decision boundaries in high dimensional spaces in a multi-layered setting, which results in a reduction in the computation cost compared to LoTeNet without any degradation in performance.
更多查看译文
关键词
tensor,image classification,networks,multi-layered
AI 理解论文
溯源树
样例
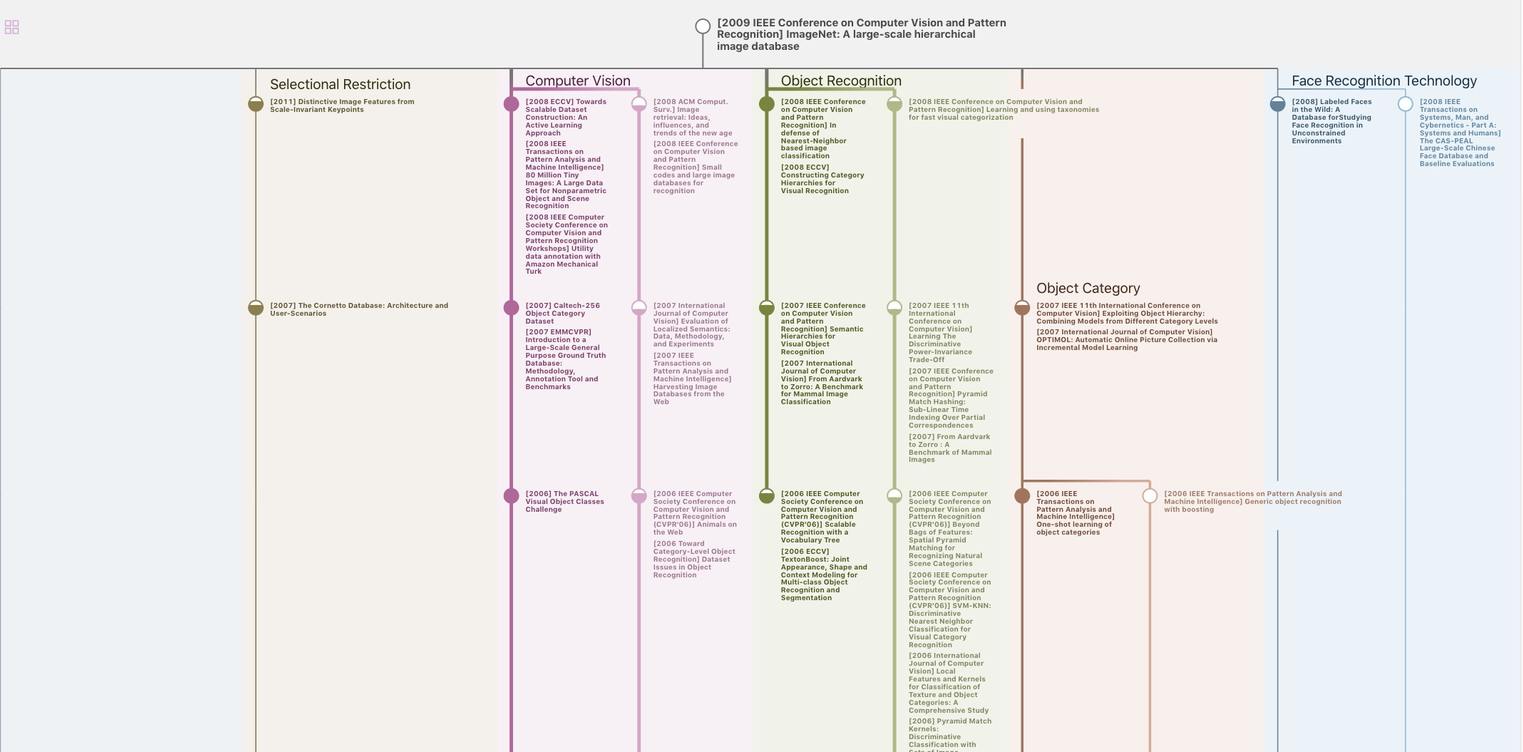
生成溯源树,研究论文发展脉络
Chat Paper
正在生成论文摘要