A graph density-based strategy for features fusion from different peak extract software to achieve more metabolites in metabolic profiling from high-resolution mass spectrometry
Analytica Chimica Acta(2020)
摘要
In metabolomics study, it is not easy to extract the metabolites from data of ultra high-performance liquid chromatography-high-resolution mass spectrometry, especially for those with low abundance. Different software for peak recognition and matching use different algorithms, leading to different extract results. Therefore, integration of results from different software can obtain richer metabolome information, but the redundant features should be removed. In this study, an integrated strategy of fusing features and removing redundancy based on graph density (FRRGD) was proposed. A graph is used to cover the ion features generated by two open access software (XCMS, MZmine 2) and a software (SIEVE) from an instrument vendor, and redundant features were removed by searching the maximal complete sub-graphs. A standard mixture containing 41 metabolites and a spontaneous urine were utilized to develop the method and demonstrate its usefulness. For the standard mixture, 19, 19 and 27 metabolites were extracted by XCMS, MZmine 2 and SIEVE, respectively. After fusion by FRRGD, 37 metabolites were obtained. For the diluted spontaneous urine sample, 1103, 1500 and 387 metabolites were extracted by XCMS, MZmine 2 and SIEVE, respectively, FRRGD produced 1619 metabolites which were much more than individual software, significantly increasing metabolome coverage. The proposed FRRGD shows a great prospect as a new data processing strategy for metabolomics study.
更多查看译文
关键词
Untargeted metabolomics,Low abundant metabolites,Graph density,Removal of redundancy,Mass spectrometry,Metabolic profiling
AI 理解论文
溯源树
样例
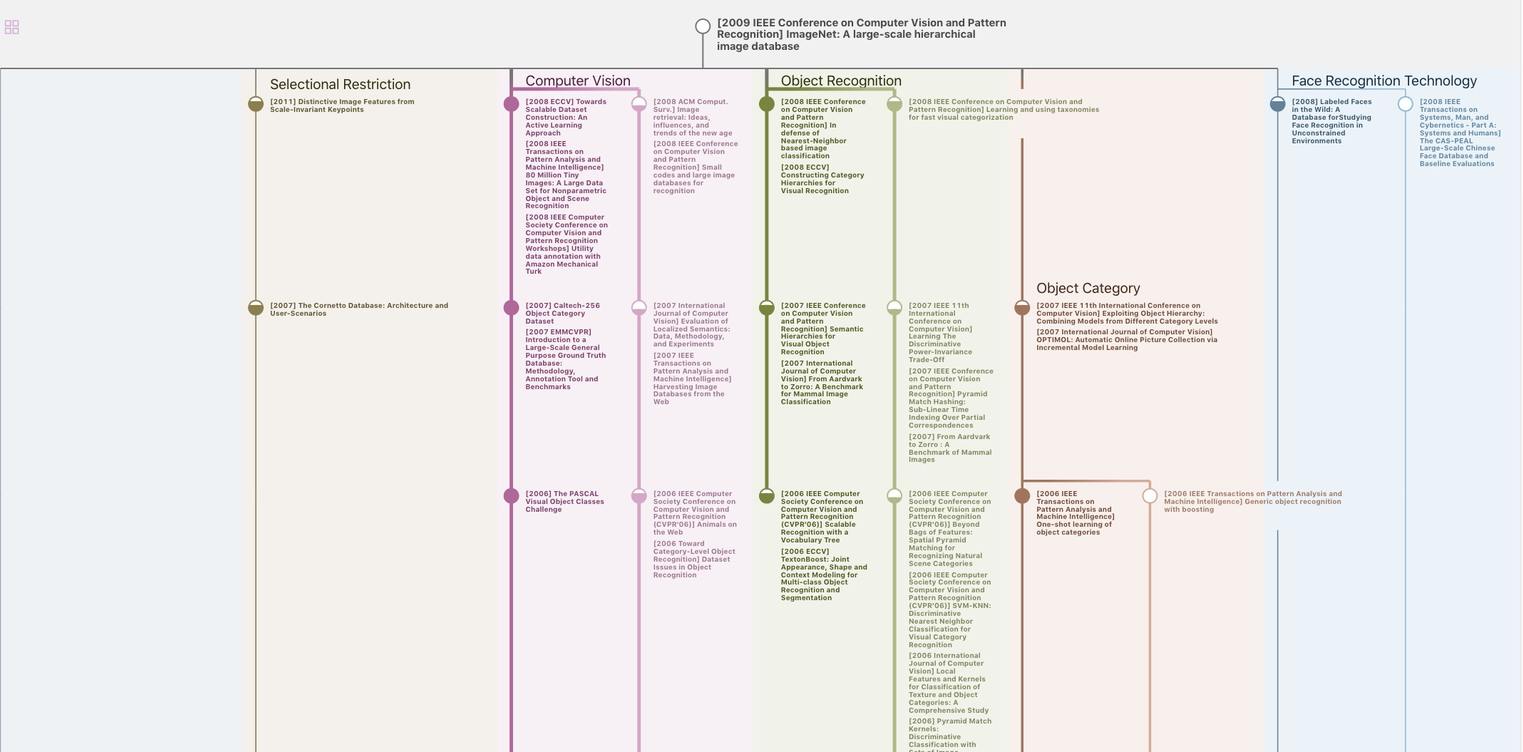
生成溯源树,研究论文发展脉络
Chat Paper
正在生成论文摘要