A comparative study of semi- and self-supervised semantic segmentation of biomedical microscopy data
arXiv (Cornell University)(2020)
摘要
In recent years, Convolutional Neural Networks (CNNs) have become the state-of-the-art method for biomedical image analysis. However, these networks are usually trained in a supervised manner, requiring large amounts of labelled training data. These labelled data sets are often difficult to acquire in the biomedical domain. In this work, we validate alternative ways to train CNNs with fewer labels for biomedical image segmentation using. We adapt two semi- and self-supervised image classification methods and analyse their performance for semantic segmentation of biomedical microscopy images.
更多查看译文
关键词
semantic segmentation,self-supervised
AI 理解论文
溯源树
样例
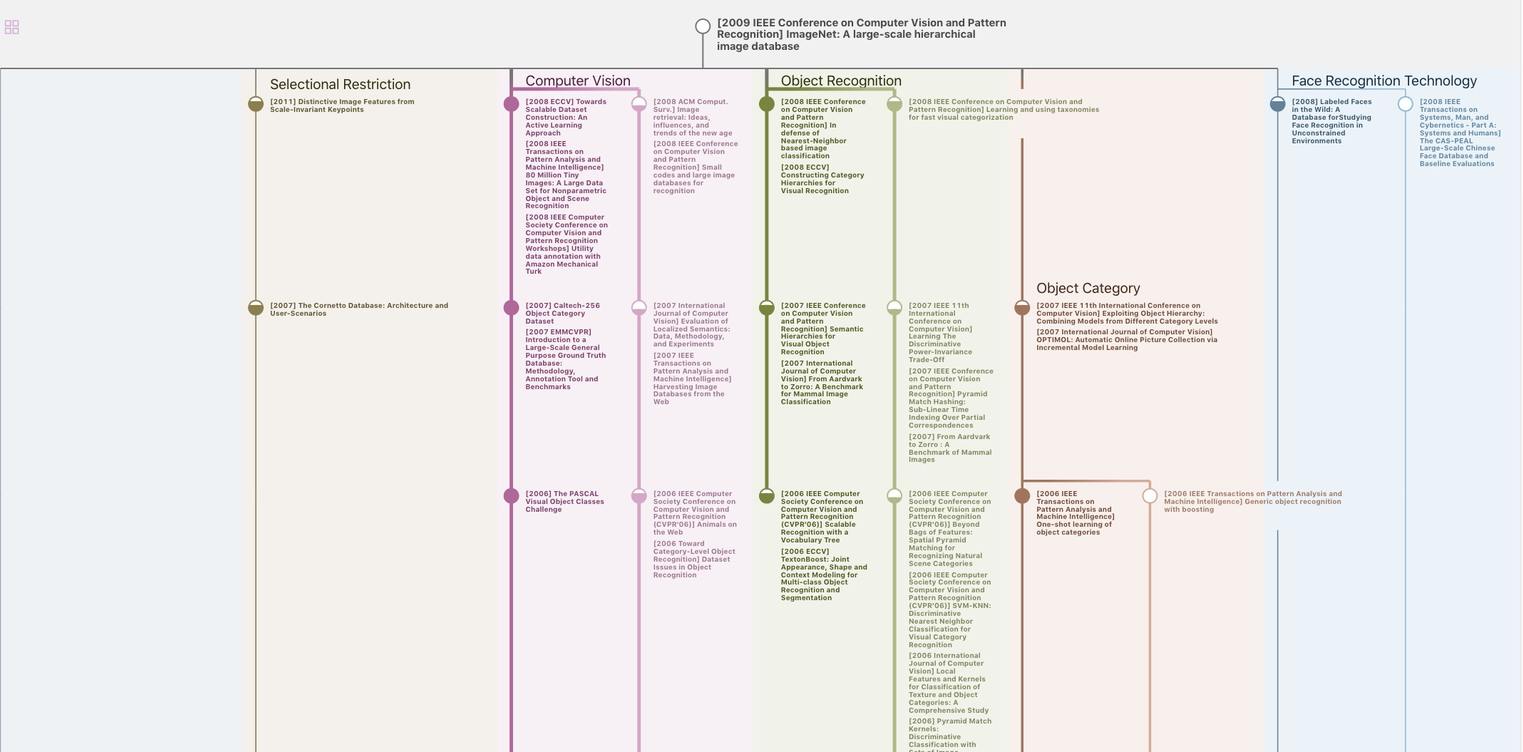
生成溯源树,研究论文发展脉络
Chat Paper
正在生成论文摘要