Recovering and Simulating Pedestrians in the Wild.
CoRL(2020)
摘要
Sensor simulation is a key component for testing the performance of self-driving vehicles and for data augmentation to better train perception systems. Typical approaches rely on artists to create both 3D assets and their animations to generate a new scenario. This, however, does not scale. In contrast, we propose to recover the shape and motion of pedestrians from sensor readings captured in the wild by a self-driving car driving around. Towards this goal, we formulate the problem as energy minimization in a deep structured model that exploits human shape priors, reprojection consistency with 2D poses extracted from images, and a ray-caster that encourages the reconstructed mesh to agree with the LiDAR readings. Importantly, we do not require any ground-truth 3D scans or 3D pose annotations. We then incorporate the reconstructed pedestrian assets bank in a realistic LiDAR simulation system by performing motion retargeting, and show that the simulated LiDAR data can be used to significantly reduce the amount of annotated real-world data required for visual perception tasks.
更多查看译文
关键词
simulating pedestrians
AI 理解论文
溯源树
样例
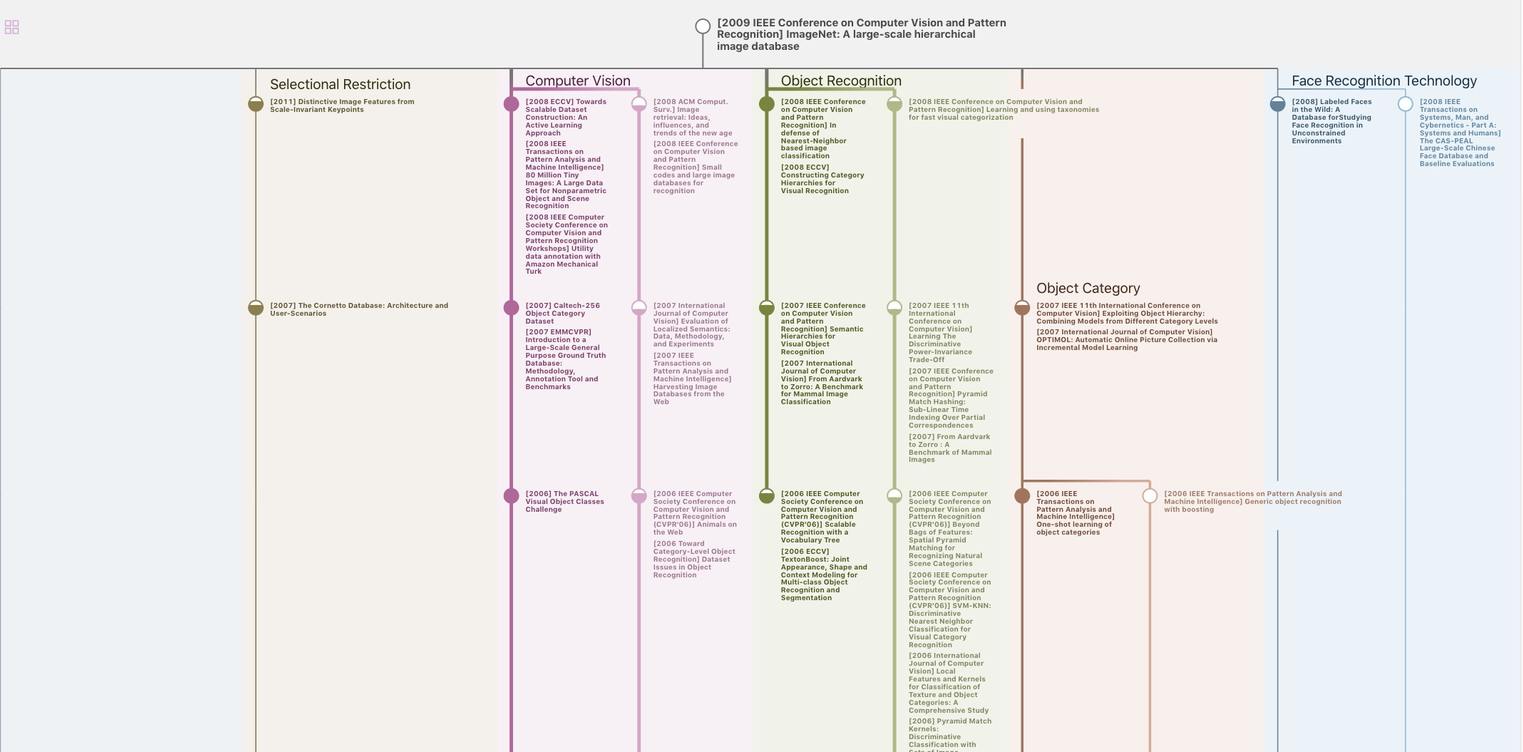
生成溯源树,研究论文发展脉络
Chat Paper
正在生成论文摘要