Dense Label Encoding for Boundary Discontinuity Free Rotation Detection
2021 IEEE/CVF CONFERENCE ON COMPUTER VISION AND PATTERN RECOGNITION, CVPR 2021(2021)
摘要
Rotation detection serves as a fundamental building block in many visual applications involving aerial image, scene text, and face etc. Differing from the dominant regression-based approaches for orientation estimation, this paper explores a relatively less-studied methodology based on classification. The hope is to inherently dismiss the boundary discontinuity issue as encountered by the regression-based detectors. We propose new techniques to push its frontier in two aspects: i) new encoding mechanism: the design of two Densely Coded Labels (DCL) for angle classification, to replace the Sparsely Coded Label (SCL) in existing classification-based detectors, leading to three times training speed increase as empirically observed across benchmarks, further with notable improvement in detection accuracy; ii) loss re-weighting: we propose Angle Distance and Aspect Ratio Sensitive Weighting (ADARSW), which improves the detection accuracy especially for square-like objects, by making DCL-based detectors sensitive to angular distance and object's aspect ratio. Extensive experiments and visual analysis on large-scale public datasets for aerial images i.e. DOTA, UCAS-AOD, HRSC2016, as well as scene text dataset ICDAR2015 and MLT, show the effectiveness of our approach. The source code is available at DCL and is also integrated in our open source rotation detection benchmark: RotationDetection.
更多查看译文
关键词
label,detection
AI 理解论文
溯源树
样例
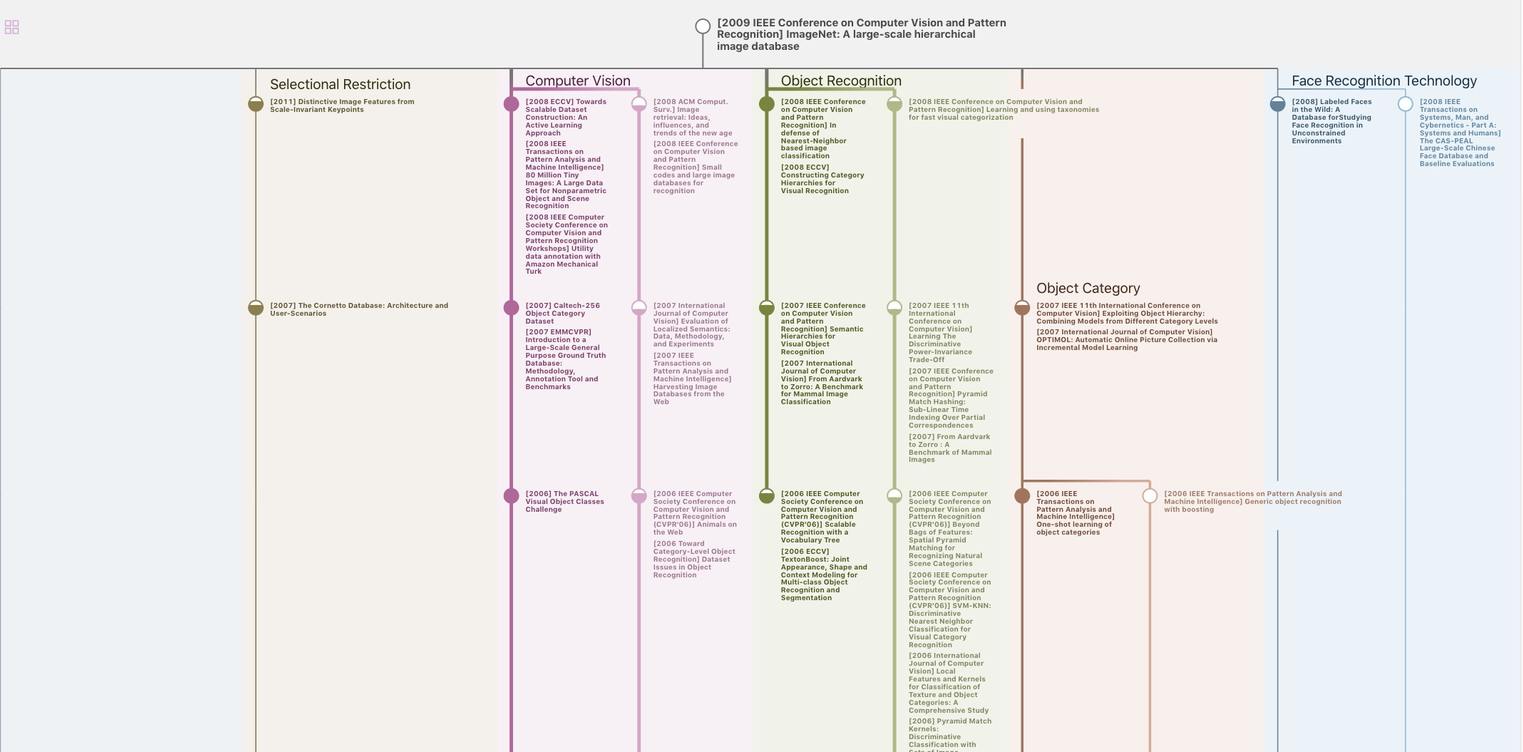
生成溯源树,研究论文发展脉络
Chat Paper
正在生成论文摘要