Graph Attention Tracking
2021 IEEE/CVF CONFERENCE ON COMPUTER VISION AND PATTERN RECOGNITION, CVPR 2021(2021)
摘要
Siamese network based trackers formulate the visual tracking task as a similarity matching problem. Almost all popular Siamese trackers realize the similarity learning via convolutional feature cross-correlation between a target branch and a search branch. However, since the size of target feature region needs to be pre-fixed, these cross-correlation base methods suffer from either reserving much adverse background information or missing a great deal of foreground information. Moreover, the global matching between the target and search region also largely neglects the target structure and part-level information. In this paper, to solve the above issues, we propose a simple target-aware Siamese graph attention network for general object tracking. We propose to establish part-to-part correspondence between the target and the search region with a complete bipartite graph, and apply the graph attention mechanism to propagate target information from the template feature to the search feature. Further, instead of using the pre-fixed region cropping for template-feature-area selection, we investigate a target-aware area selection mechanism to fit the size and aspect ratio variations of different objects. Experiments on challenging benchmarks including GOT-10k, UAV123, OTB-100 and LaSOT demonstrate that the proposed SiamGAT outperforms many stateof-the-art trackers and achieves leading performance.
更多查看译文
关键词
graph attention tracking,Siamese network,visual tracking task,similarity matching problem,popular Siamese trackers,convolutional feature cross-correlation,target branch,search branch,target feature region,cross-correlation base methods,reserving much adverse background information,foreground information,global matching,search region,target structure,part-level information,simple target-aware Siamese graph attention network,general object tracking,part-to-part correspondence,complete bipartite graph,graph attention mechanism,target information,template feature,search feature,pre-fixed region cropping,template-feature-area selection,target-aware area selection mechanism,temperature 10.0 K
AI 理解论文
溯源树
样例
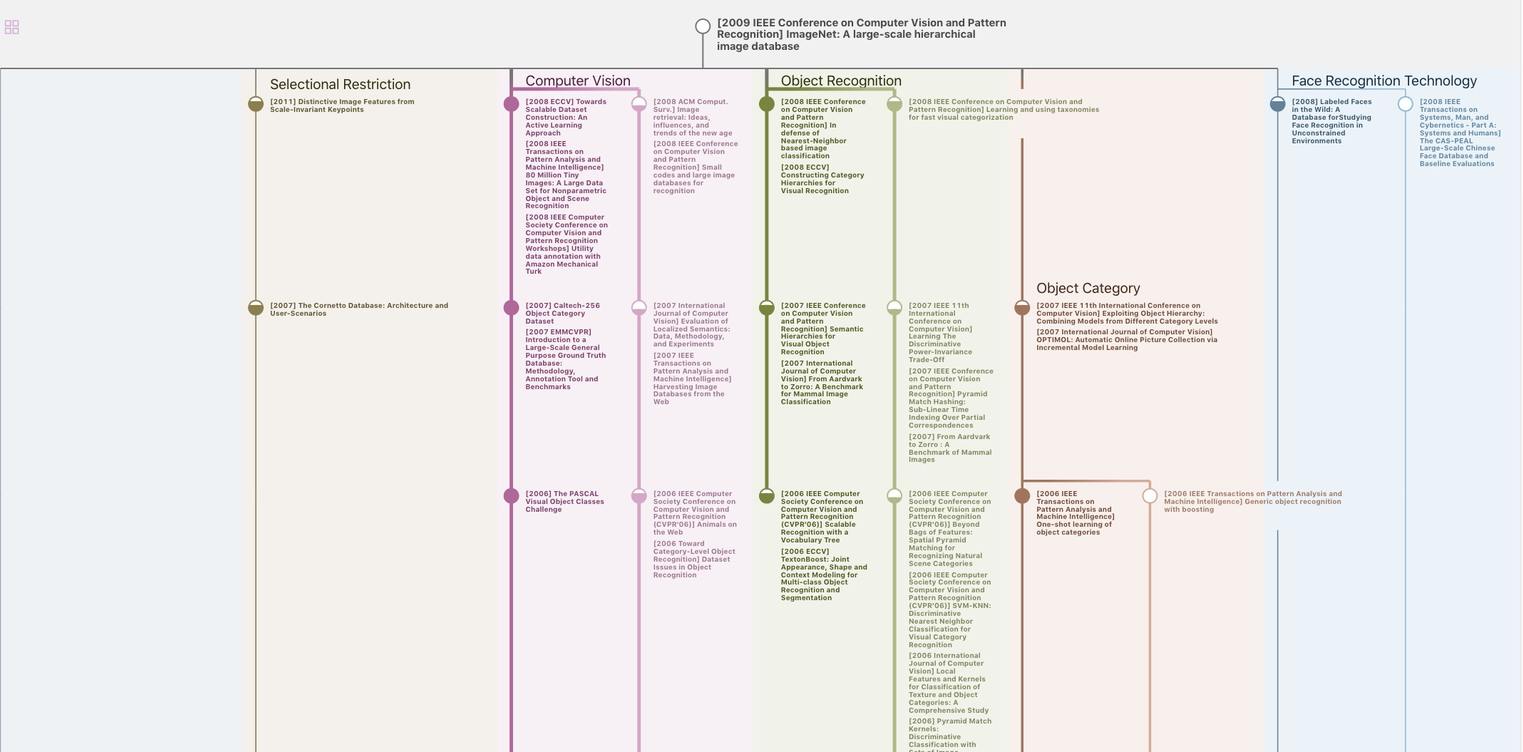
生成溯源树,研究论文发展脉络
Chat Paper
正在生成论文摘要