Neural Text Classification by Jointly Learning to Cluster and Align.
arxiv(2023)
摘要
Distributional text clustering delivers semantically informative representations and captures the relevance between each word and semantic clustering centroids. We extend the neural text clustering approach to text classification tasks by inducing cluster centers via a variational autoencoder and interacting with distributional word embeddings, to enrich the text representation and measure the relatedness between tokens and each learnable cluster centroid. The proposed method jointly learns word clustering centroids and cluster-token alignments, achieving competitive results on multiple benchmark datasets and proving that the proposed cluster-token alignment mechanism is favorable to text classification. Notably, the learned text representations are well-clustered, which matches the ground-truth categories. Experimental results show that our model can also improve the classification performance on top of BERT representations. To the best of our knowledge, we are the first adopting the variational autoencoder to update clustering centroids for text classification.
更多查看译文
关键词
neural text classification, neural clustering
AI 理解论文
溯源树
样例
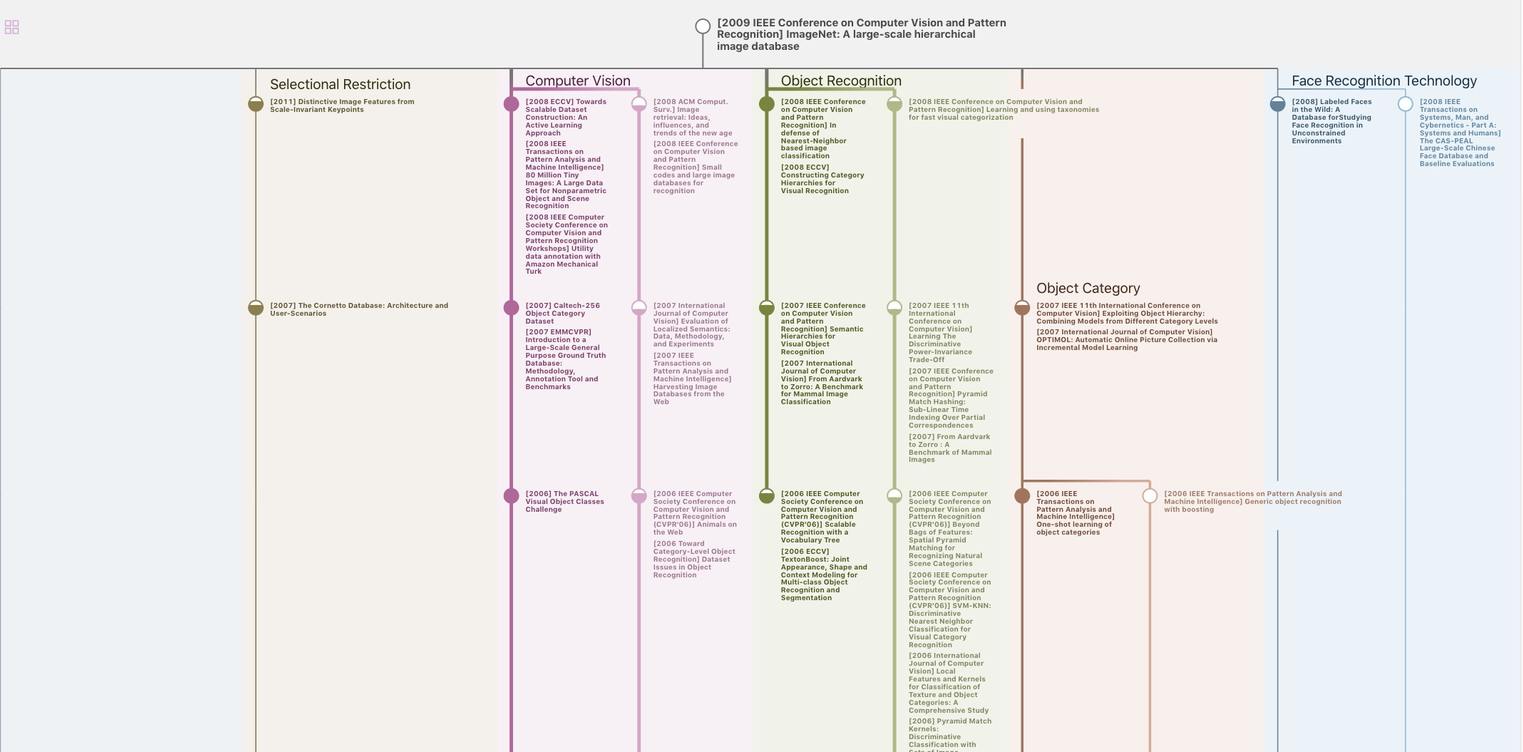
生成溯源树,研究论文发展脉络
Chat Paper
正在生成论文摘要