Automatic Encoding and Repair of Reactive High Level Tasks with Learned Abstract Representations
Accepted, Robotics Research: the 18th Annual Symposium(2019)
摘要
We present a framework that, given a set of skills a robot can perform, abstracts sensor data into symbols that are used to automatically encode the robot’s capabilities in Linear Temporal Logic (LTL). We specify reactive high-level tasks based on these capabilities, for which a strategy is automatically synthesized and executed on the robot, if the task is feasible. If a task is not feasible given the robot’s capabilities, our framework automatically suggests additional skills for the robot that would make the task feasible. We demonstrate our framework on a Baxter robot manipulating blocks on a table.
更多查看译文
关键词
representations,learned
AI 理解论文
溯源树
样例
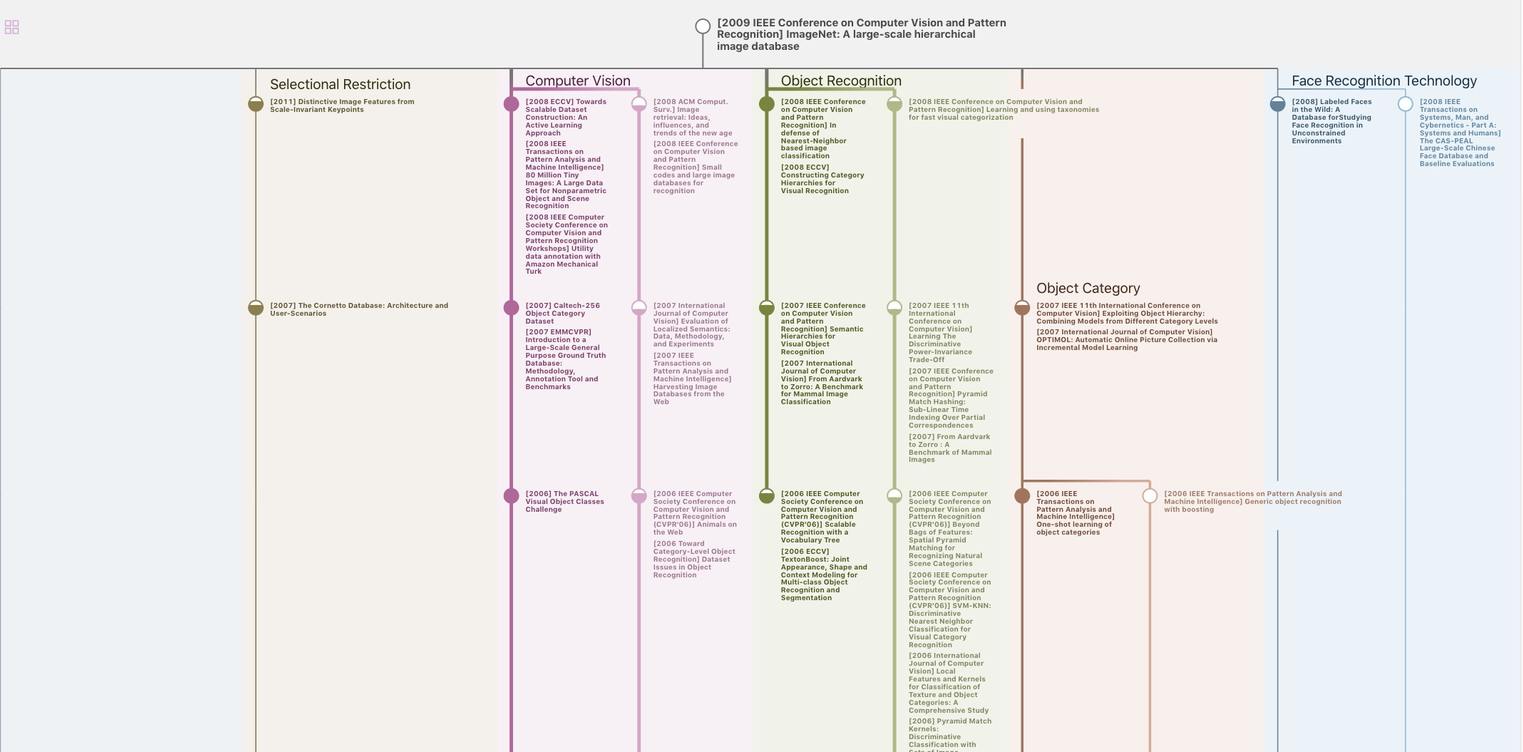
生成溯源树,研究论文发展脉络
Chat Paper
正在生成论文摘要