Msb-Fcn: Multi-Scale Bidirectional Fcn For Object Skeleton Extraction
IEEE TRANSACTIONS ON IMAGE PROCESSING(2021)
摘要
The performance of state-of-the-art object skeleton detection (OSD) methods have been greatly boosted by Convolutional Neural Networks (CNNs). However, the most existing CNN-based OSD methods rely on a 'skip-layer' structure where low-level and high-level features are combined to gather multi-level contextual information. Unfortunately, as shallow features tend to be noisy and lack semantic knowledge, they will cause errors and inaccuracy. Therefore, in order to improve the accuracy of object skeleton detection, we propose a novel network architecture, the Multi-Scale Bidirectional Fully Convolutional Network (MSB-FCN), to better gather and enhance multi-scale high-level contextual information. The advantage is that only deep features are used to construct multi-scale feature representations along with a bidirectional structure for better capturing contextual knowledge. This enables the proposed MSB-FCN to learn semantic-level information from different sub-regions. Moreover, we introduce dense connections into the bidirectional structure to ensure that the learning process at each scale can directly encode information from all other scales. An attention pyramid is also integrated into our MSB-FCN to dynamically control information propagation and reduce unreliable features. Extensive experiments on various benchmarks demonstrate that the proposed MSB-FCN achieves significant improvements over the state-of-the-art algorithms.
更多查看译文
关键词
Skeleton, Feature extraction, Image edge detection, Semantics, Task analysis, Network architecture, Benchmark testing, Salient skeleton detection, deep learning, multi-scale features
AI 理解论文
溯源树
样例
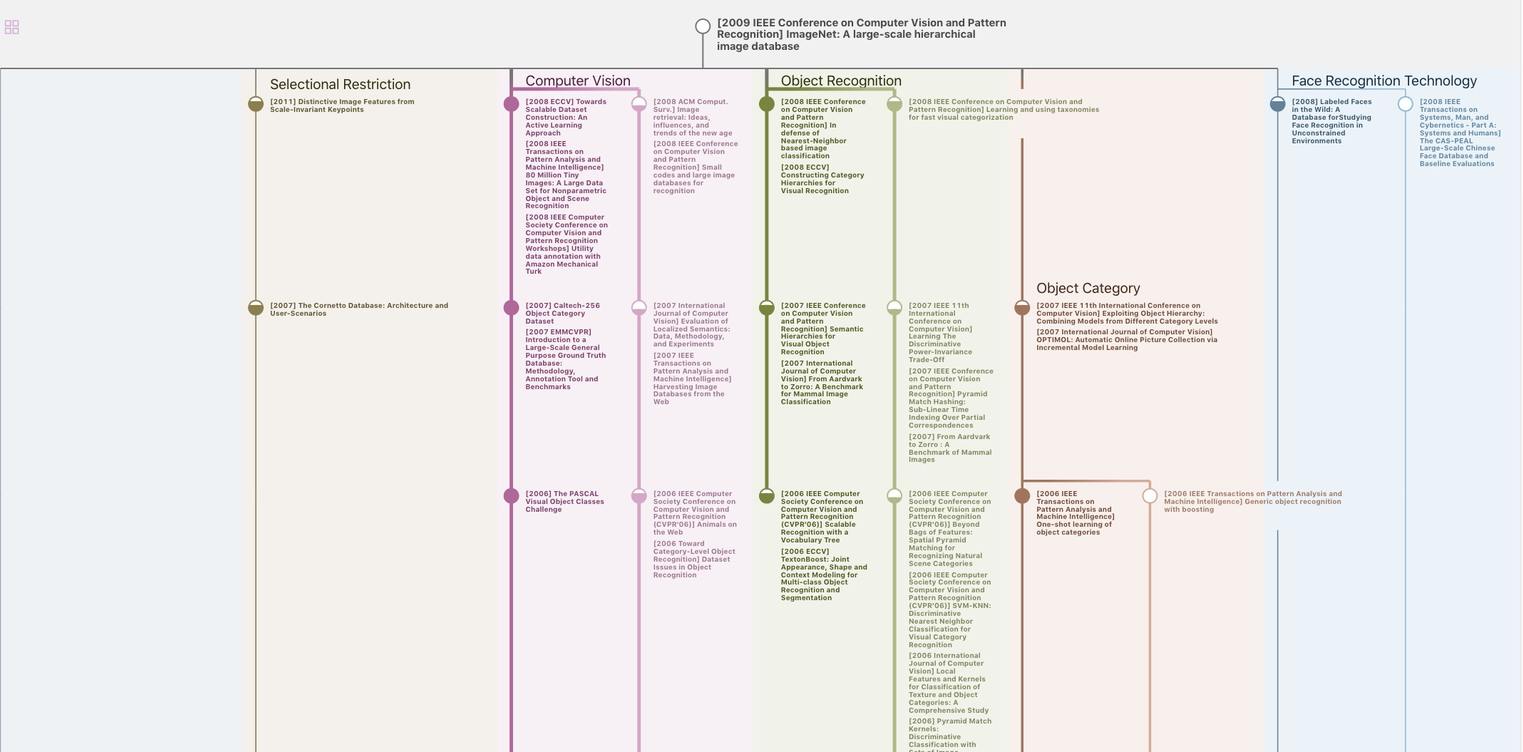
生成溯源树,研究论文发展脉络
Chat Paper
正在生成论文摘要