Integrative analysis of structural variations using short-reads and linked-reads yields highly specific and sensitive predictions.
PLOS COMPUTATIONAL BIOLOGY(2020)
摘要
Author summary Cancer and many other diseases are often driven by structural rearrangements in the patients. Their precise identification is necessary to understand evolution and cure for the disease. In this study, we have compared two sequencing technologies for the identification of structural variations i.e. Illumina's short-reads and 10X Genomics linked-reads sequencing. Short-reads sequencing is already known to have high false discovery rate for structural variations, while, an unbiased performance evaluation of linked-reads sequencing is missing. Hence, we evaluate the performance of these two technologies using computational and PCR based methodologies. Moreover, we also present a statistical approach to increase their performance, supporting better detection of structural variations and thus further research into disease biology. Genetic diseases are driven by aberrations of the human genome. Identification of such aberrations including structural variations (SVs) is key to our understanding. Conventional short-reads whole genome sequencing (cWGS) can identify SVs to base-pair resolution, but utilizes only short-range information and suffers from high false discovery rate (FDR). Linked-reads sequencing (10XWGS) utilizes long-range information by linkage of short-reads originating from the same large DNA molecule. This can mitigate alignment-based artefacts especially in repetitive regions and should enable better prediction of SVs. However, an unbiased evaluation of this technology is not available. In this study, we performed a comprehensive analysis of different types and sizes of SVs predicted by both the technologies and validated with an independent PCR based approach. The SVs commonly identified by both the technologies were highly specific, while validation rate dropped for uncommon events. A particularly high FDR was observed for SVs only found by 10XWGS. To improve FDR and sensitivity, statistical models for both the technologies were trained. Using our approach, we characterized SVs from the MCF7 cell line and a primary breast cancer tumor with high precision. This approach improves SV prediction and can therefore help in understanding the underlying genetics in various diseases.
更多查看译文
AI 理解论文
溯源树
样例
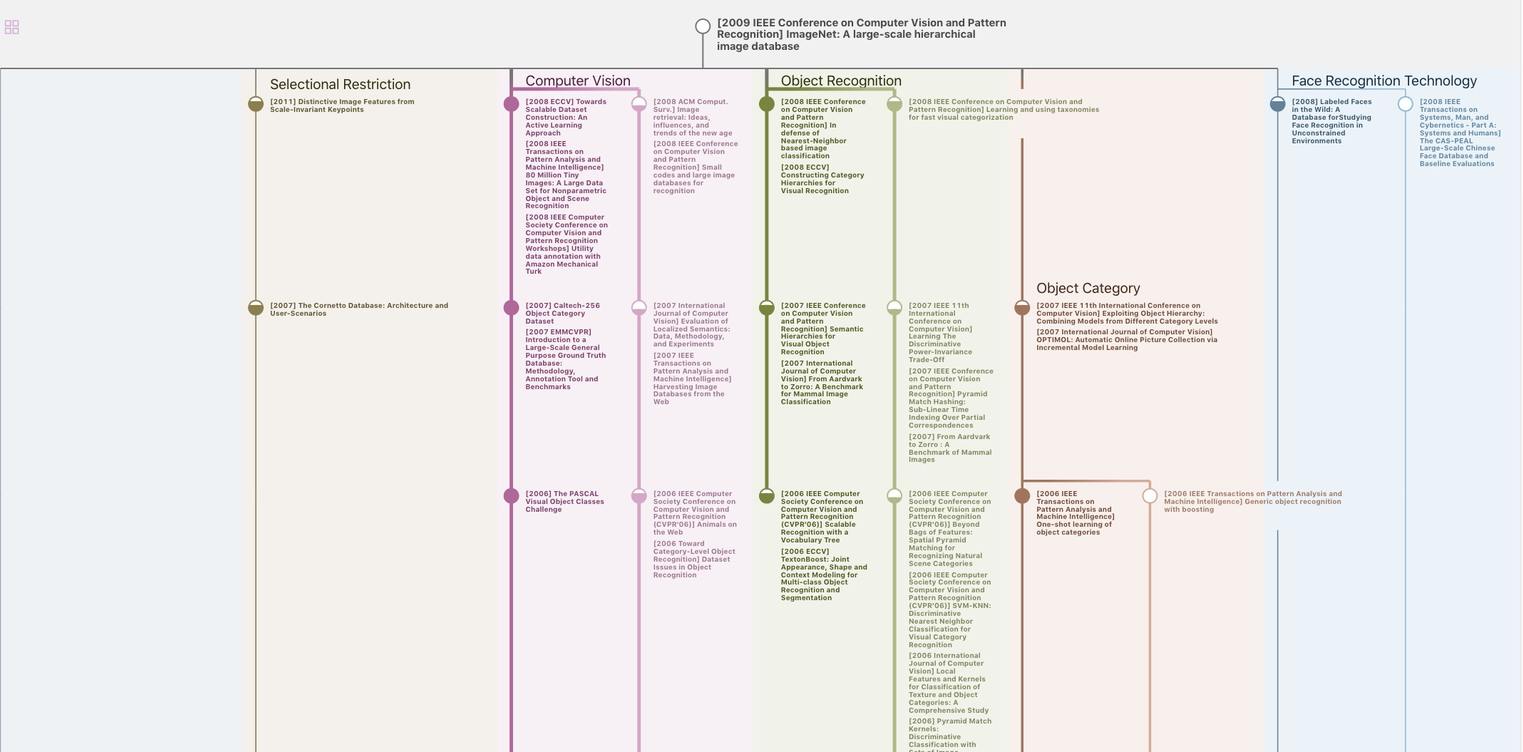
生成溯源树,研究论文发展脉络
Chat Paper
正在生成论文摘要