Decoding and Diversity in Machine Translation
arxiv(2020)
摘要
Neural Machine Translation (NMT) systems are typically evaluated using automated metrics that assess the agreement between generated translations and ground truth candidates. To improve systems with respect to these metrics, NLP researchers employ a variety of heuristic techniques, including searching for the conditional mode (vs. sampling) and incorporating various training heuristics (e.g., label smoothing). While search strategies significantly improve BLEU score, they yield deterministic outputs that lack the diversity of human translations. Moreover, search tends to bias the distribution of translated gender pronouns. This makes human-level BLEU a misleading benchmark in that modern MT systems cannot approach human-level BLEU while simultaneously maintaining human-level translation diversity. In this paper, we characterize distributional differences between generated and real translations, examining the cost in diversity paid for the BLEU scores enjoyed by NMT. Moreover, our study implicates search as a salient source of known bias when translating gender pronouns.
更多查看译文
关键词
machine translation,diversity
AI 理解论文
溯源树
样例
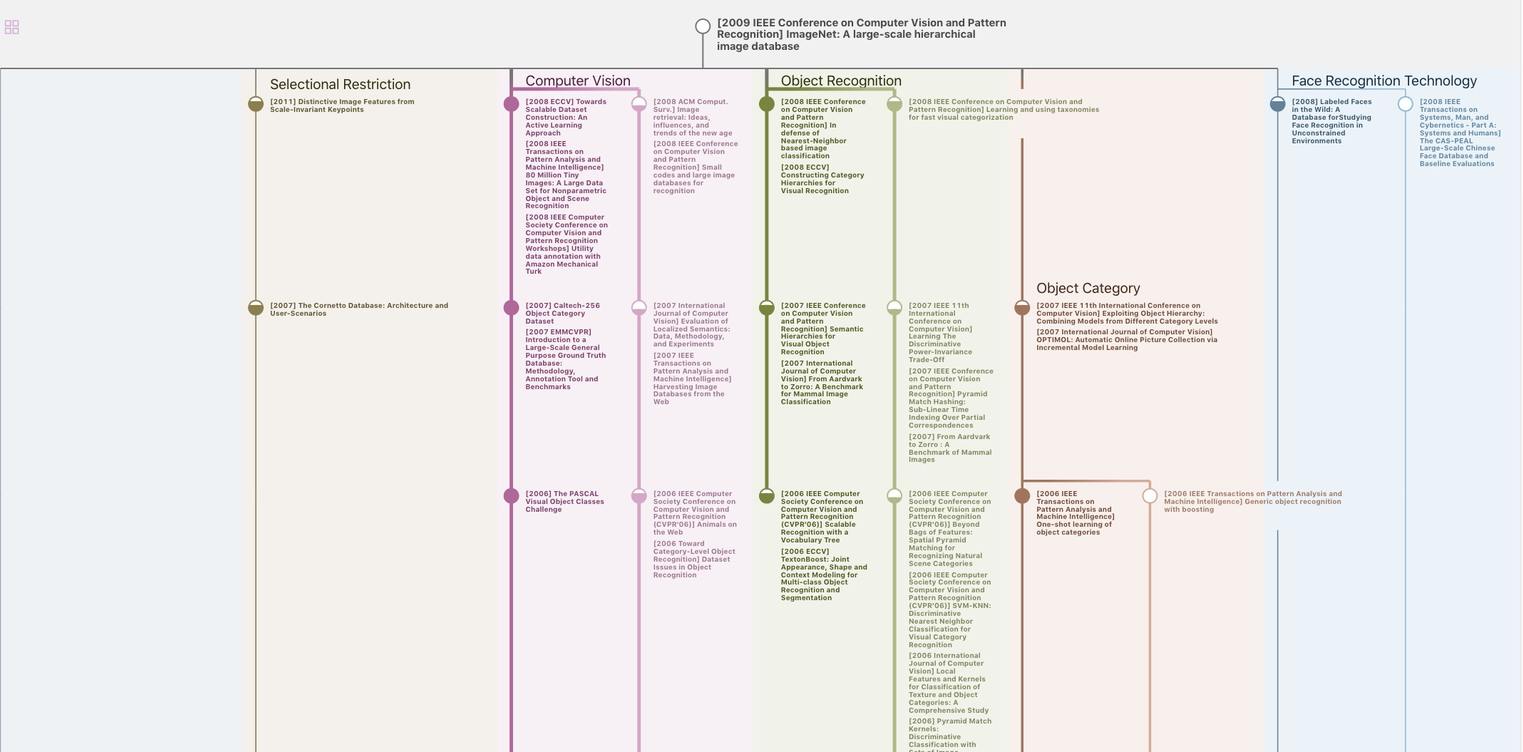
生成溯源树,研究论文发展脉络
Chat Paper
正在生成论文摘要