Broken adaptive ridge regression for right-censored survival data
Annals of the Institute of Statistical Mathematics(2021)
摘要
Broken adaptive ridge (BAR) is a computationally scalable surrogate to L_0 -penalized regression, which involves iteratively performing reweighted L_2 penalized regressions and enjoys some appealing properties of both L_0 and L_2 penalized regressions while avoiding some of their limitations. In this paper, we extend the BAR method to the semi-parametric accelerated failure time (AFT) model for right-censored survival data. Specifically, we propose a censored BAR (CBAR) estimator by applying the BAR algorithm to the Leurgan’s synthetic data and show that the resulting CBAR estimator is consistent for variable selection, possesses an oracle property for parameter estimation and enjoys a grouping property for highly correlation covariates. Both low- and high-dimensional covariates are considered. The effectiveness of our method is demonstrated and compared with some popular penalization methods using simulations. Real data illustrations are provided on a diffuse large-B-cell lymphoma data and a glioblastoma multiforme data.
更多查看译文
关键词
Accelerated failure time model, Grouping effect,
penalization, Right censoring, Variable selection
AI 理解论文
溯源树
样例
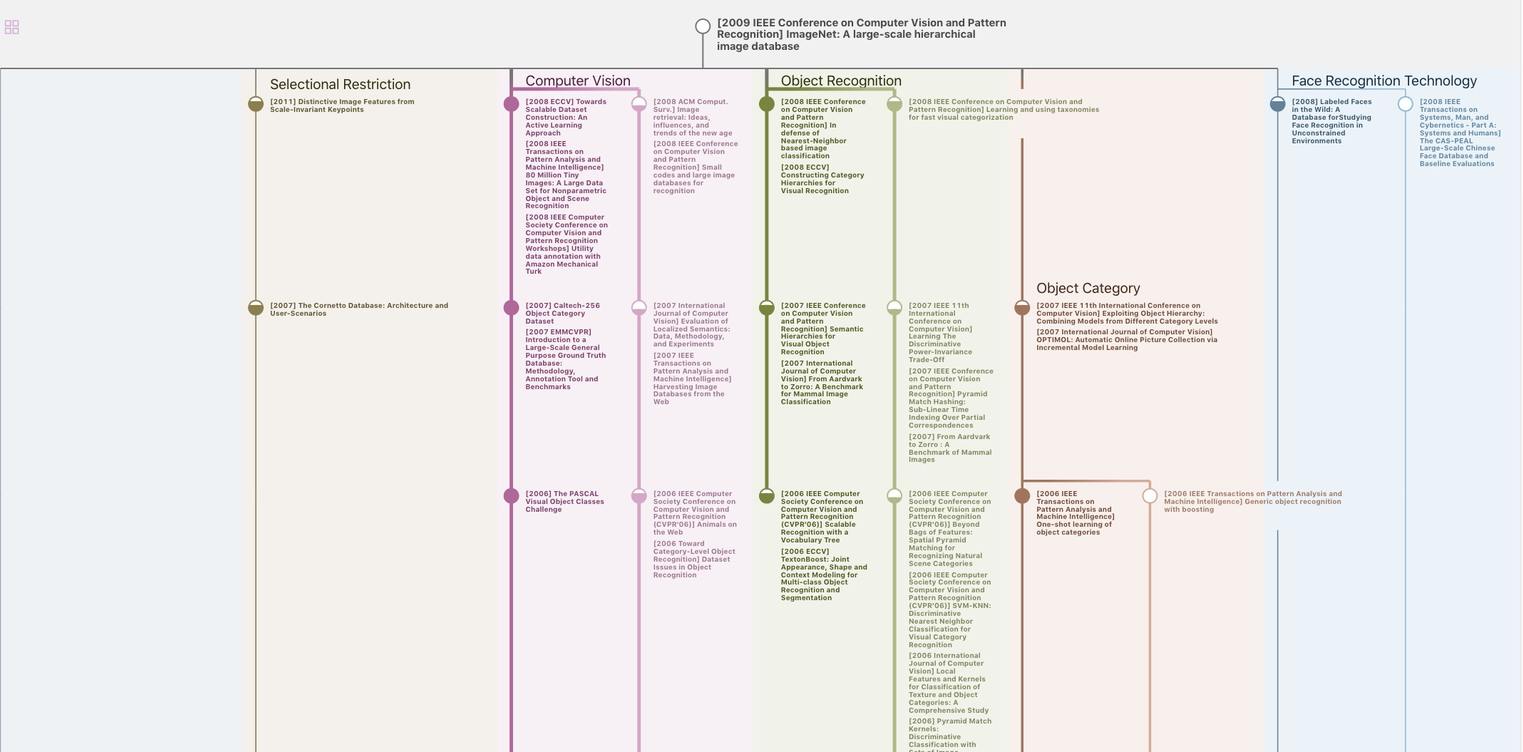
生成溯源树,研究论文发展脉络
Chat Paper
正在生成论文摘要