Gradient descent for deep matrix factorization: Dynamics and implicit bias towards low rank
APPLIED AND COMPUTATIONAL HARMONIC ANALYSIS(2024)
摘要
In deep learning, it is common to use more network parameters than training points. In such scenario of over-parameterization, there are usually multiple networks that achieve zero training error so that the training algorithm induces an implicit bias on the computed solution. In practice, (stochastic) gradient descent tends to prefer solutions which generalize well, which provides a possible explanation of the success of deep learning. In this paper we analyze the dynamics of gradient descent in the simplified setting of linear networks and of an estimation problem. Although we are not in an overparameterized scenario, our analysis nevertheless provides insights into the phenomenon of implicit bias. In fact, we derive a rigorous analysis of the dynamics of vanilla gradient descent, and characterize the dynamical convergence of the spectrum. We are able to accurately locate time intervals where the effective rank of the iterates is close to the effective rank of a low-rank projection of the ground -truth matrix. In practice, those intervals can be used as criteria for early stopping if a certain regularity is desired. We also provide empirical evidence for implicit bias in more general scenarios, such as matrix sensing and random initialization. This suggests that deep learning prefers trajectories whose complexity (measured in terms of effective rank) is monotonically increasing, which we believe is a fundamental concept for the theoretical understanding of deep learning.(c) 2023 Elsevier Inc. All rights reserved.
更多查看译文
关键词
Gradient descent,Implicit bias/regularization,Matrix factorization,Neural networks
AI 理解论文
溯源树
样例
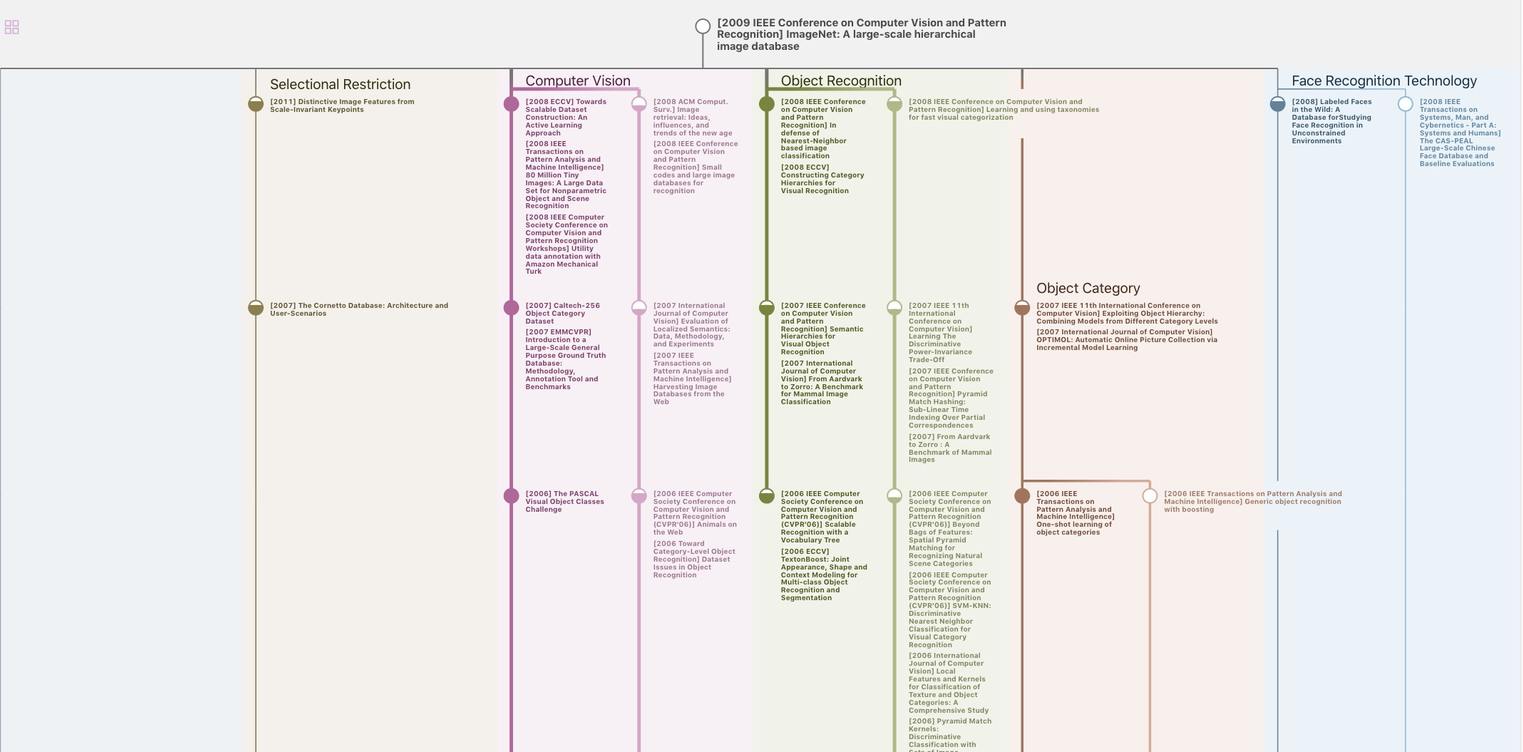
生成溯源树,研究论文发展脉络
Chat Paper
正在生成论文摘要