Clutter Identification based on Sparse Recovery with Dynamically Changing Dictionary Sizes for Cognitive Radar
Proceedings of SPIE(2019)
摘要
Existing radar algorithms assume stationary statistical characteristics for environment/clutter. In practical scenarios, the statistical characteristics of the clutter can dynamically change depending on where the radar is operating. Nonstationarity in the statistical characteristics of the clutter may negatively affect the radar performance. Cognitive radar that can sense the changes in the clutter statistics, learn the new statistical characteristics, and adapt to these changes has been proposed to overcome these shortcomings. We have recently developed techniques for detection of statistical changes and learning the new clutter distribution for cognitive radar. In this work, we will extend the learning component. More specifically, in our previous work, we have developed a sparse recovery based clutter distribution identification to learn the distribution of the new clutter characteristics after the detected change in the statistics of the clutter. In our method, we have built a dictionary of clutter distributions and used this dictionary in orthogonal matching pursuit to perform sparse recovery of the clutter distribution assuming that the dictionary includes the new distribution. In this work, we propose a hypothesis testing based approach to detect whether the new distribution of the clutter is included in the dictionary or not, and suggest a method to dynamically update the dictionary. We envision that the successful outcomes of this work will be of high relevance to the adaptive learning and cognitive augmentation of the radar systems that are used in remotely piloted vehicles for surveillance and reconnaissance operations.
更多查看译文
关键词
Cognitive Radar,Sparse Recovery,Hypothesis Testing,Distance/Similarity measure
AI 理解论文
溯源树
样例
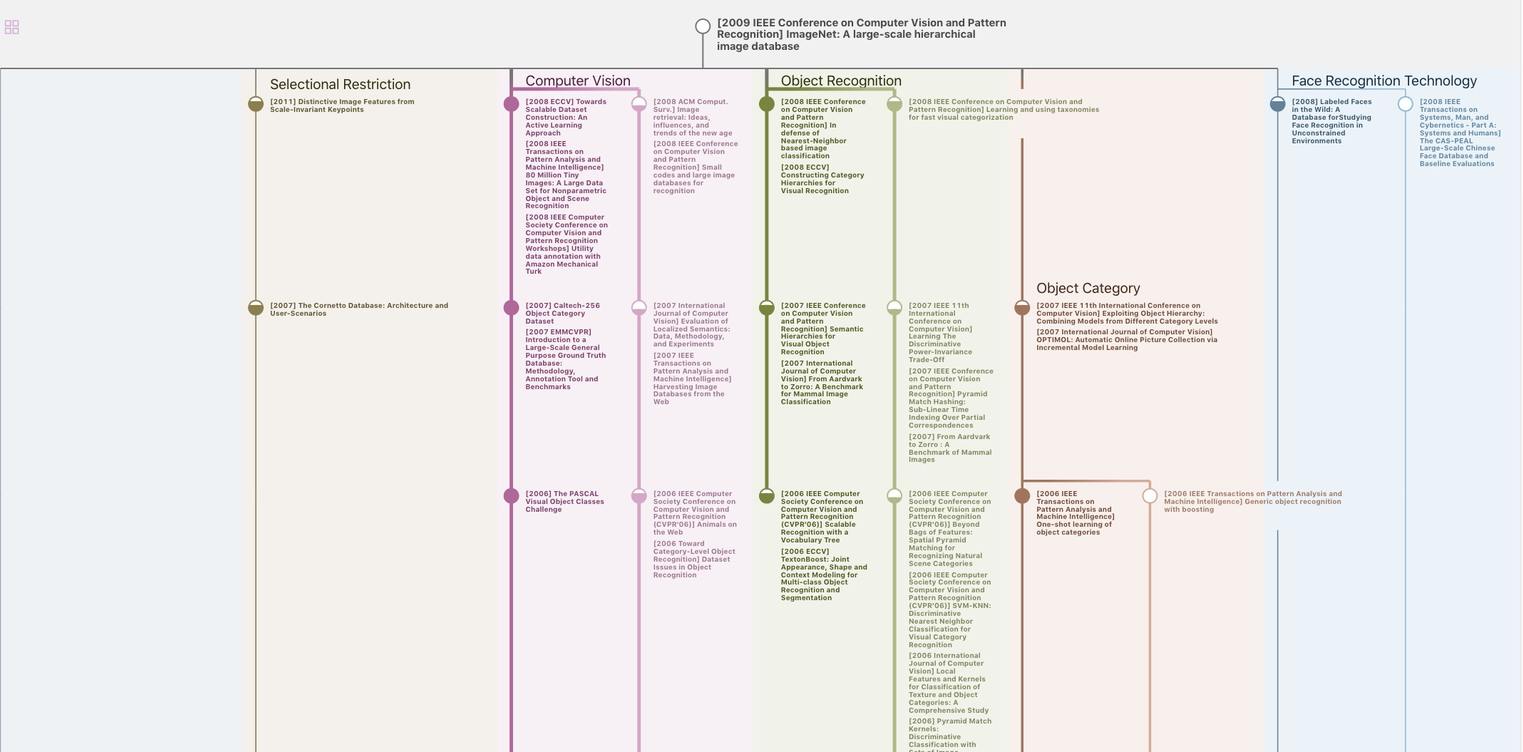
生成溯源树,研究论文发展脉络
Chat Paper
正在生成论文摘要