A Model-Based, Bayesian Characterization of Subsurface Corrosion Parameters in Composite Multi-Layered Structures
AIP Conference Proceedings(2016)
摘要
Thermographic NDE approaches to detect subsurface corrosion defects of multi-layered structures with composite top layers have proven difficult due to the fact that the thermal conductivity of composite materials is larger in lateral directions (the plane parallel to the surface) than in the through-thickness directions. This causes heat to dissipate faster laterally than through the thickness when a heat source is applied to the surface of the structure, making it difficult for subsurface damage effects to manifest on the surface, where the heat source and inspection typically occur. To address this, a heat induction approach is presented that excites the damaged, metallic bottom layer directly by Joule heating, resulting in more observable damage effects on the surface than what could be expected for traditional thermographic methods on this type of structure. To characterize the subsurface damage parameters (defect location, diameter, and depth), Bayesian inversion of numerically-simulated noisy data, using a high-fidelity, coupled electromagnetic-heat transfer model is employed. Stochastic estimation methods such as Markov chain Monte Carlo (MCMC) allow for quantification of uncertainty surrounding the damage parameters, which is important as this directly translates into uncertainty surrounding the component reliability. However, because thousands of high-fidelity finite element models are computationally costly to evaluate, as is typical in most MCMC methods, the use of Bayesian inversion is rarely feasible in real-time. To address this, a projection-based reduced order modeling (ROM) tracking and interpolation scheme is formulated within the MCMC sampling method for the multi-physics problem, resulting in significant speedup of solution time with little loss of accuracy, enabling near-real time stochastic estimation of damage.
更多查看译文
AI 理解论文
溯源树
样例
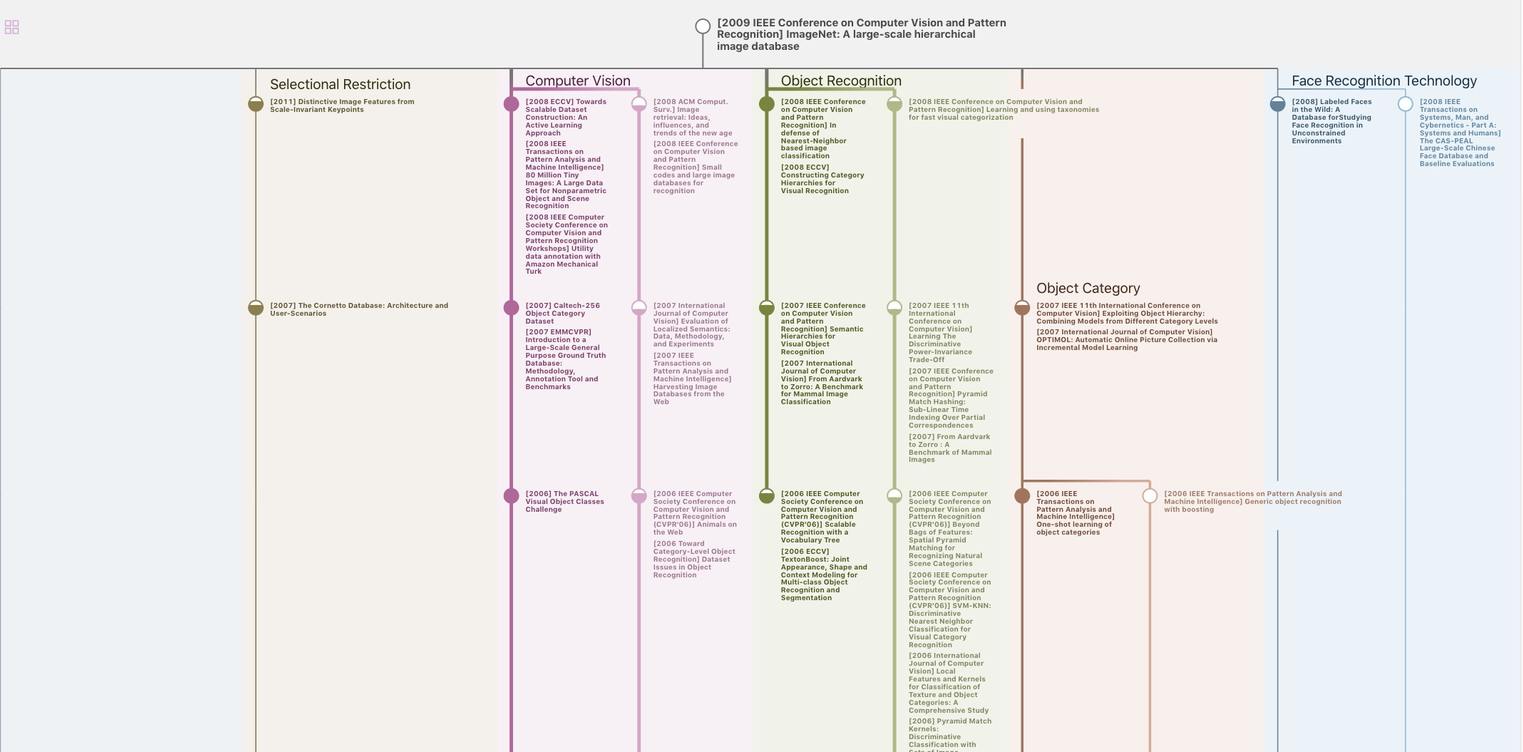
生成溯源树,研究论文发展脉络
Chat Paper
正在生成论文摘要