A Selective Survey on Versatile Knowledge Distillation Paradigm for Neural Network Models
arxiv(2020)
摘要
This paper aims to provide a selective survey about knowledge distillation(KD) framework for researchers and practitioners to take advantage of it for developing new optimized models in the deep neural network field. To this end, we give a brief overview of knowledge distillation and some related works including learning using privileged information(LUPI) and generalized distillation(GD). Even though knowledge distillation based on the teacher-student architecture was initially devised as a model compression technique, it has found versatile applications over various frameworks. In this paper, we review the characteristics of knowledge distillation from the hypothesis that the three important ingredients of knowledge distillation are distilled knowledge and loss,teacher-student paradigm, and the distillation process. In addition, we survey the versatility of the knowledge distillation by studying its direct applications and its usage in combination with other deep learning paradigms. Finally we present some future works in knowledge distillation including explainable knowledge distillation where the analytical analysis of the performance gain is studied and the self-supervised learning which is a hot research topic in deep learning community.
更多查看译文
关键词
versatile knowledge distillation paradigm,neural network models,neural network
AI 理解论文
溯源树
样例
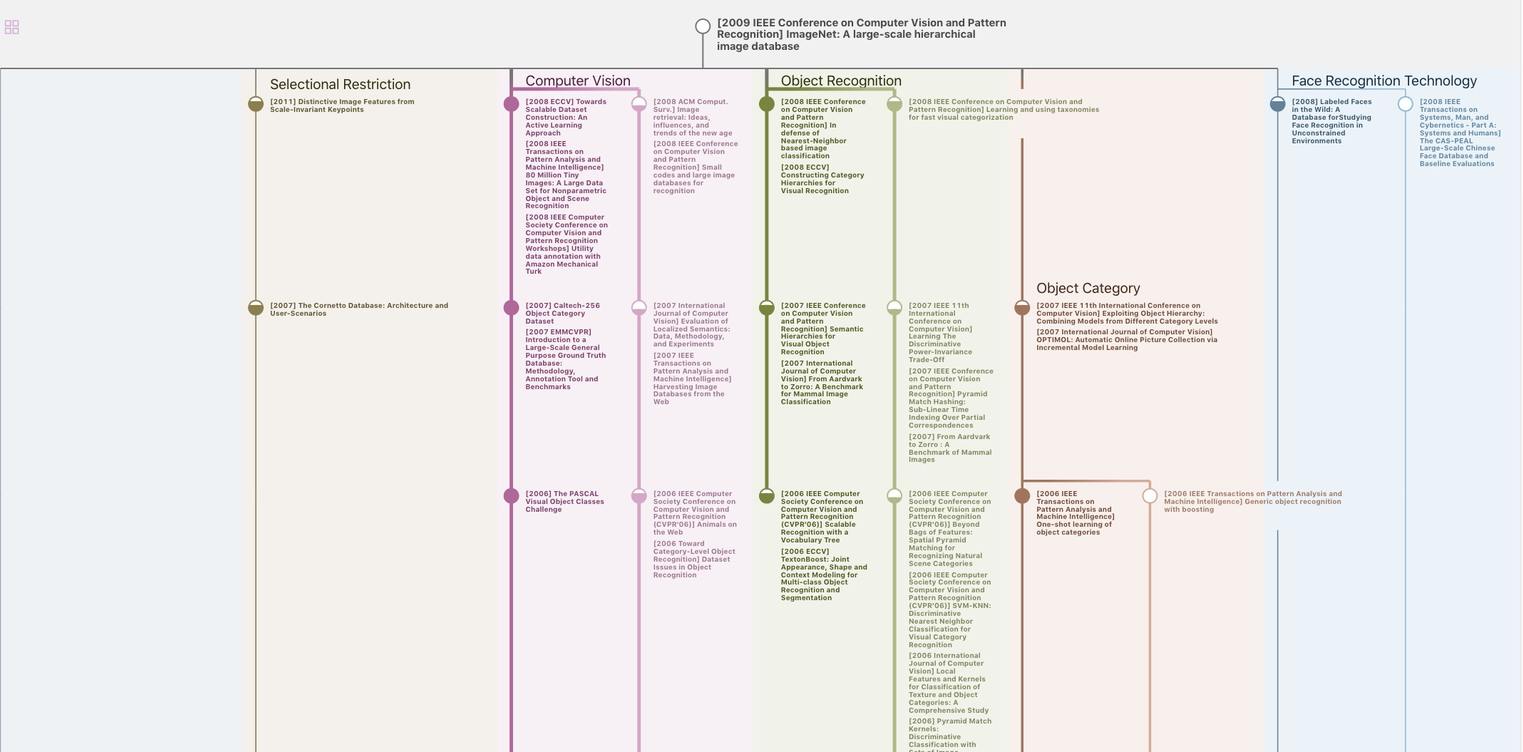
生成溯源树,研究论文发展脉络
Chat Paper
正在生成论文摘要