NeuralFusion: Online Depth Fusion in Latent Space
2021 IEEE/CVF CONFERENCE ON COMPUTER VISION AND PATTERN RECOGNITION, CVPR 2021(2021)
摘要
We present a novel online depth map fusion approach that learns depth map aggregation in a latent feature space. While previous fusion methods use an explicit scene representation like signed distance functions (SDFs), we propose a learned feature representation for the fusion. The key idea is a separation between the scene representation used for the fusion and the output scene representation, via an additional translator network. Our neural network architecture consists of two main parts: a depth and feature fusion sub-network, which is followed by a translator sub-network to produce the final surface representation (e.g. TSDF) for visualization or other tasks. Our approach is an online process, handles high noise levels, and is particularly able to deal with gross outliers common for photometric stereo-based depth maps. Experiments on real and synthetic data demonstrate improved results compared to the state of the art, especially in challenging scenarios with large amounts of noise and outliers. The source code will be made available at https://github.com/weders/NeuralFusion.
更多查看译文
关键词
online depth neuralfusion,latent space
AI 理解论文
溯源树
样例
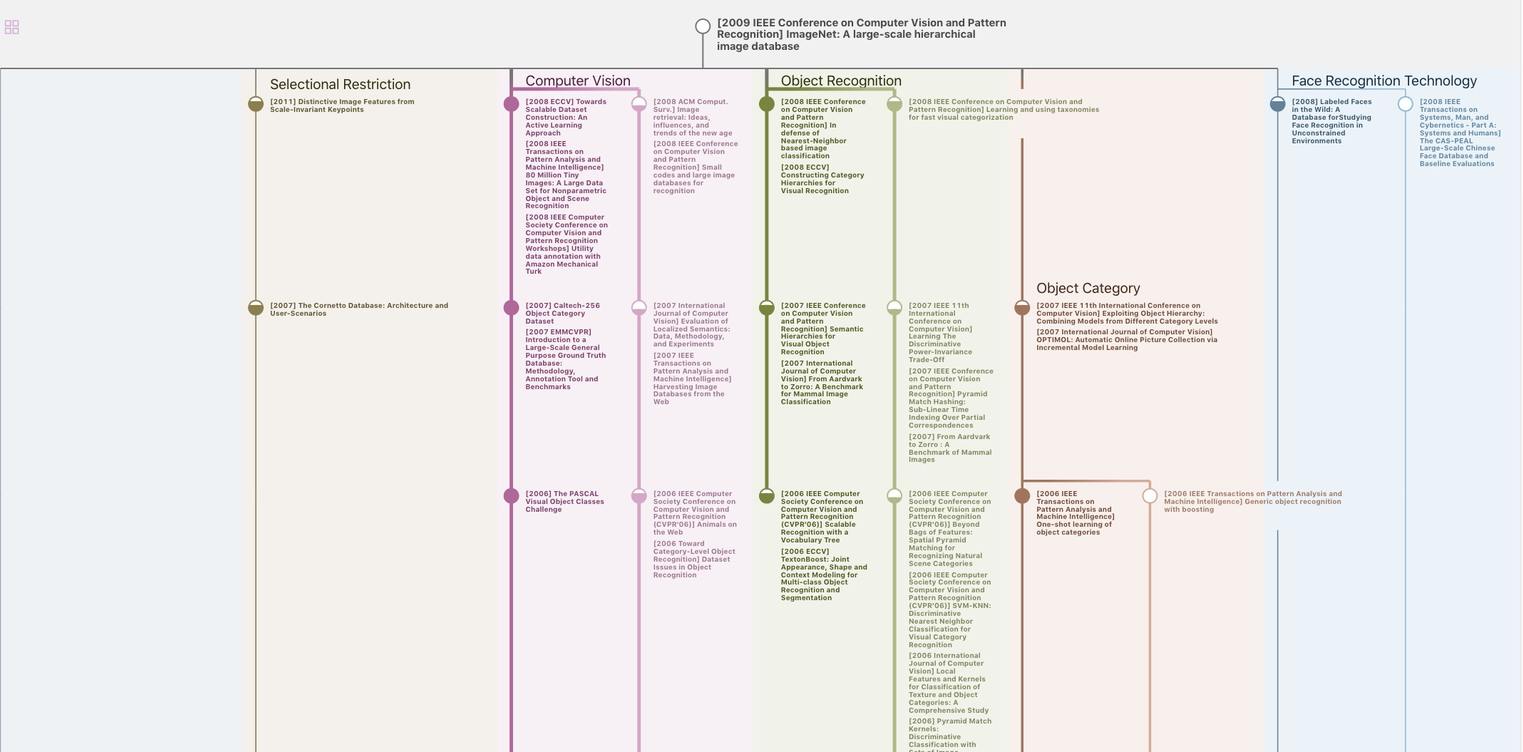
生成溯源树,研究论文发展脉络
Chat Paper
正在生成论文摘要