Distilling the Evidence to Augment Fact Verification Models
FACT EXTRACTION AND VERIFICATION (FEVER)(2020)
摘要
The alarming spread of fake news in social media, together with the impossibility of scaling manual fact verification, motivated the development of natural language processing techniques to automatically verify the veracity of claims. Most approaches perform a claim-evidence classification without providing any insights about why the claim is trustworthy or not. We propose, instead, a model-agnostic framework that consists of two modules: (1) a span extractor, which identifies the crucial information connecting claim and evidence; and (2) a classifier that combines claim, evidence, and the extracted spans to predict the veracity of the claim We show that the spans are informative for the classifier, improving performance and robustness. Tested on several state-of-the-art models over the FEVER dataset, the enhanced classifiers consistently achieve higher accuracy while also showing reduced sensitivity to artifacts in the claims.
更多查看译文
关键词
evidence,fact,models
AI 理解论文
溯源树
样例
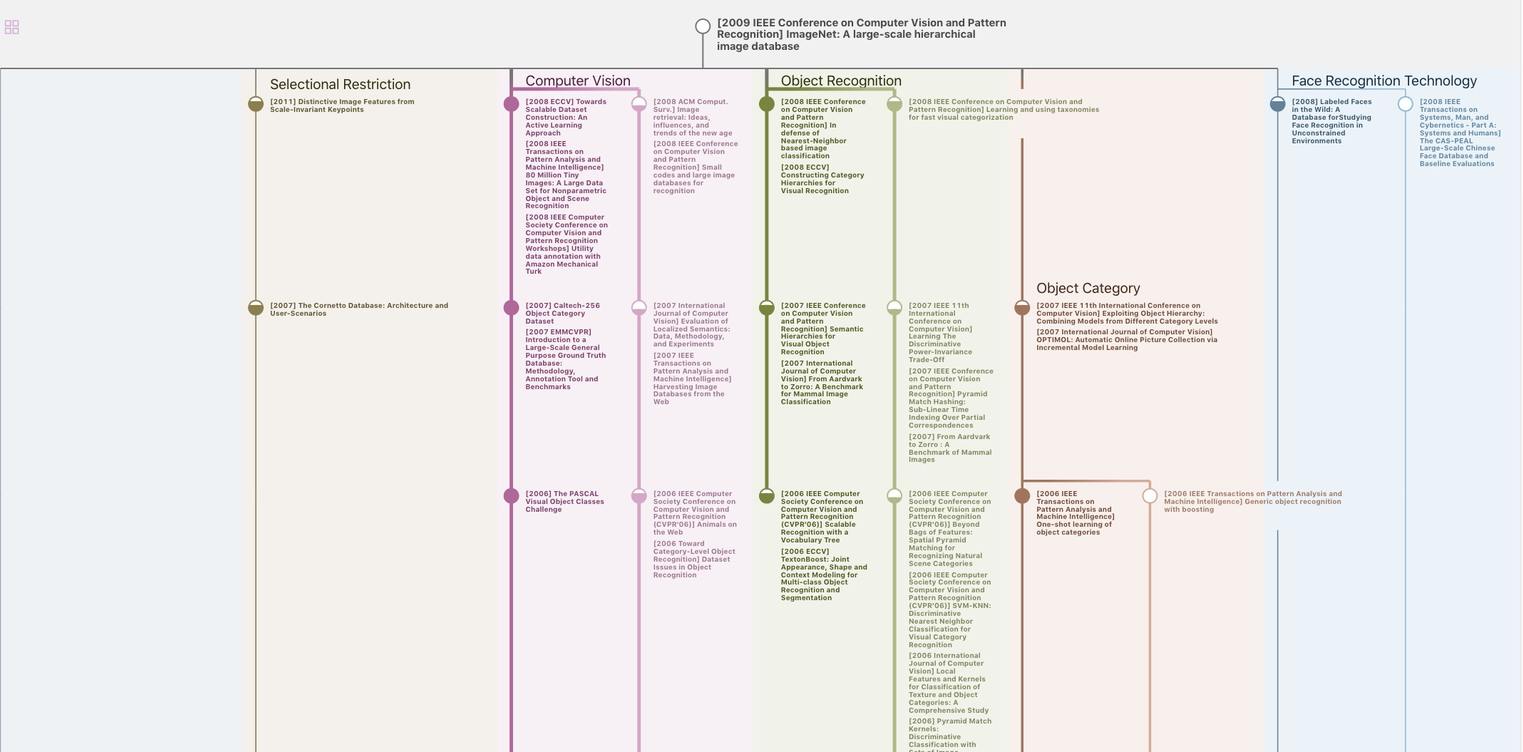
生成溯源树,研究论文发展脉络
Chat Paper
正在生成论文摘要