AUTOMATIC SELECTION OF RELEVANT DATA FOR PAPER MACHINE DIAGNOSTICS
J-FOR-JOURNAL OF SCIENCE & TECHNOLOGY FOR FOREST PRODUCTS AND PROCESSES(2015)
摘要
This article presents a method to focus the attention of process operators and experts on the most relevant data in a large data base when searching for the root cause of a detected process disturbance. The method is generic in that it analyzes control loops individually based on their signal triplets of set-point, measurement, and control output. The model of normal operation is a Gaussian mixture model (GMM) for a two-dimensional feature vector identified at regular intervals, e.g., once per day for a two-week period. When a process disturbance occurs, the relevant control loops are chosen based on their poor correspondence to the normal operating model. Data for hundreds of control loops can be reduced to data for 10-30 relevant loops, thus focussing the analysis significantly. The efficiency of GMM modeling methods is discussed. The method is demonstrated with real-life paper production data involving hundreds of control loops.
更多查看译文
AI 理解论文
溯源树
样例
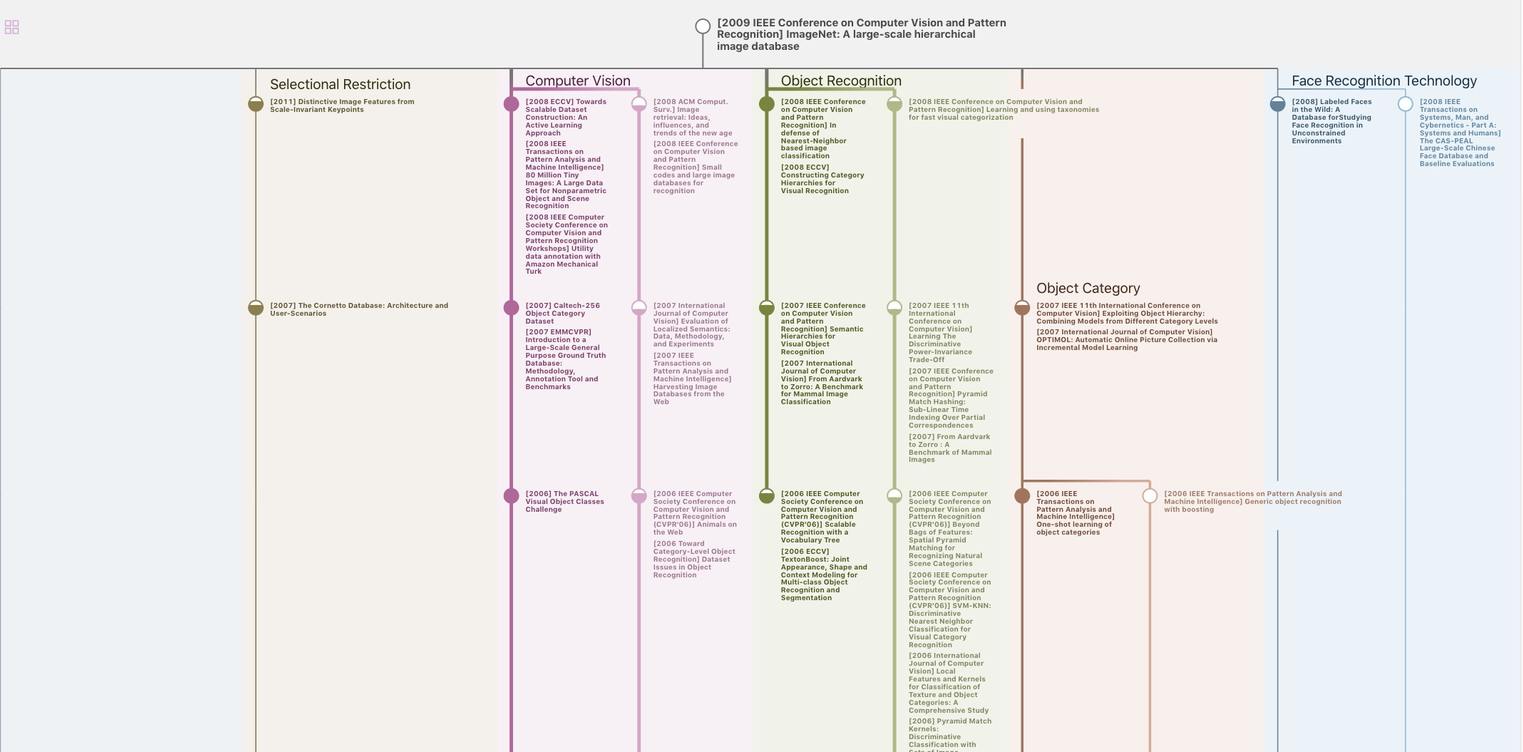
生成溯源树,研究论文发展脉络
Chat Paper
正在生成论文摘要