CONVOLUTIVE TRANSFER FUNCTION INVARIANT SDR TRAINING CRITERIA FOR MULTI-CHANNEL REVERBERANT SPEECH SEPARATION
2021 IEEE INTERNATIONAL CONFERENCE ON ACOUSTICS, SPEECH AND SIGNAL PROCESSING (ICASSP 2021)(2021)
摘要
Time-domain training criteria have proven to be very effective for the separation of single-channel non-reverberant speech mixtures. Likewise, mask-based beamforming has shown impressive performance in multi-channel reverberant speech enhancement and source separation. Here, we propose to combine neural network supported multi-channel source separation with a time-domain training objective function. For the objective we propose to use a convolutive transfer function invariant Signal-to-Distortion Ratio (CI-SDR) based loss. While this is a well-known evaluation metric (BSS Eval), it has not been used as a training objective before. To show the effectiveness, we demonstrate the performance on LibriSpeech based reverberant mixtures. On this task, the proposed system approaches the error rate obtained on single-source non-reverberant input, i.e., LibriSpeech test clean, with a difference of only 1.2 percentage points, thus outperforming a conventional permutation invariant training based system and alternative objectives like Scale Invariant Signal-to-Distortion Ratio by a large margin.
更多查看译文
关键词
Multi-channel source separation, acoustic beamforming, complex backpropagation, Signal-to-Distortion Ratio
AI 理解论文
溯源树
样例
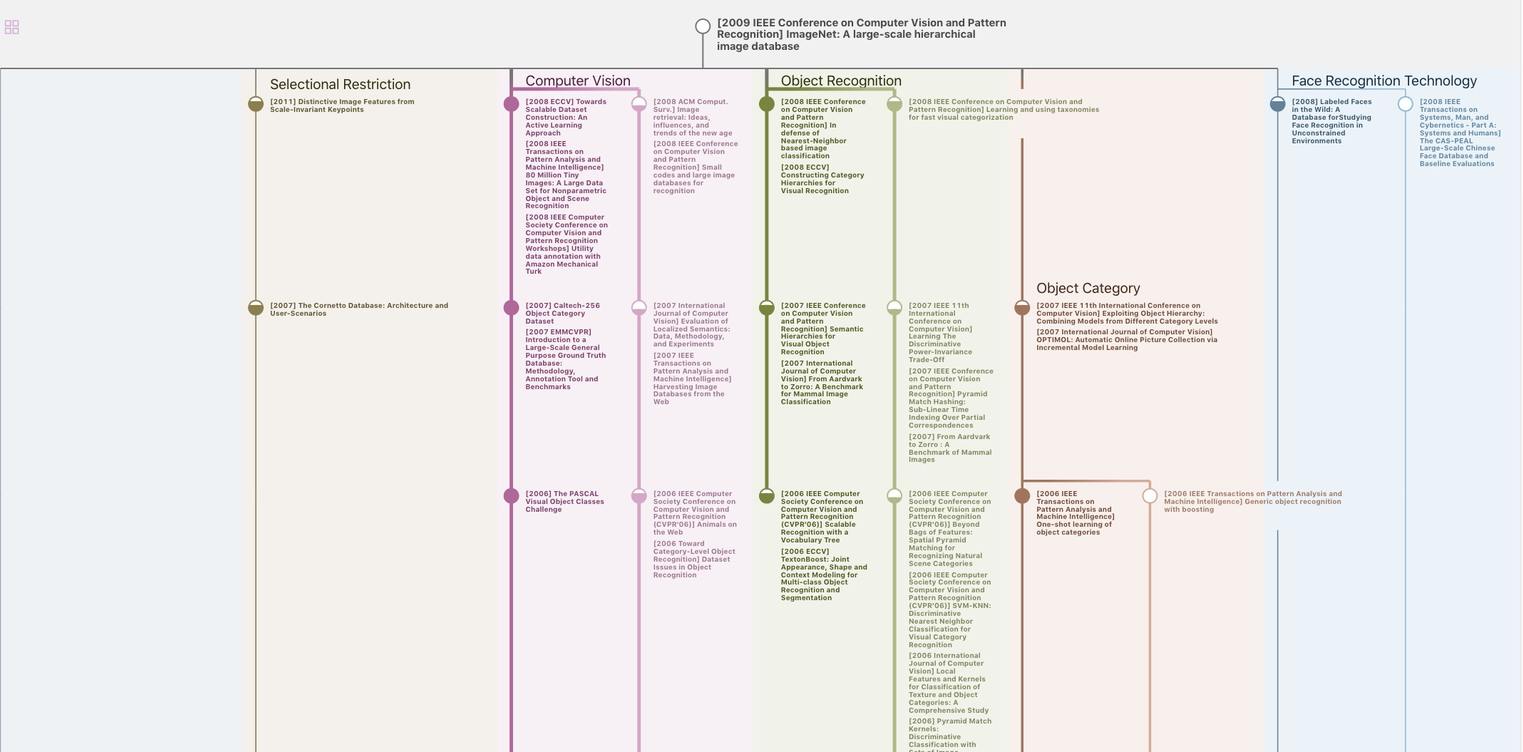
生成溯源树,研究论文发展脉络
Chat Paper
正在生成论文摘要