Detect, Reject, Correct: Crossmodal Compensation of Corrupted Sensors
2021 IEEE INTERNATIONAL CONFERENCE ON ROBOTICS AND AUTOMATION (ICRA 2021)(2021)
摘要
Using sensor data from multiple modalities presents an opportunity to encode redundant and complementary features that can be useful when one modality is corrupted or noisy. Humans do this everyday, relying on touch and proprioceptive feedback in visually-challenging environments. However, robots might not always know when their sensors are corrupted, as even broken sensors can return valid values. In this work, we introduce the Crossmodal Compensation Model (CCM), which can detect corrupted sensor modalities and compensate for them. CMM is a representation model learned with self-supervision that leverages unimodal reconstruction loss for corruption detection. CCM then discards the corrupted modality and compensates for it with information from the remaining sensors. We show that CCM learns rich state representations that can be used for manipulation policies learned with reinforcement learning, even when input modalities are corrupted during policy rollout in ways not seen during training.
更多查看译文
关键词
corrupted sensors,sensor data,multiple modalities,proprioceptive feedback,visually-challenging environments,crossmodal compensation model,CCM,sensor modalities,unimodal reconstruction loss,corruption detection,corrupted modality,reinforcement learning,robots
AI 理解论文
溯源树
样例
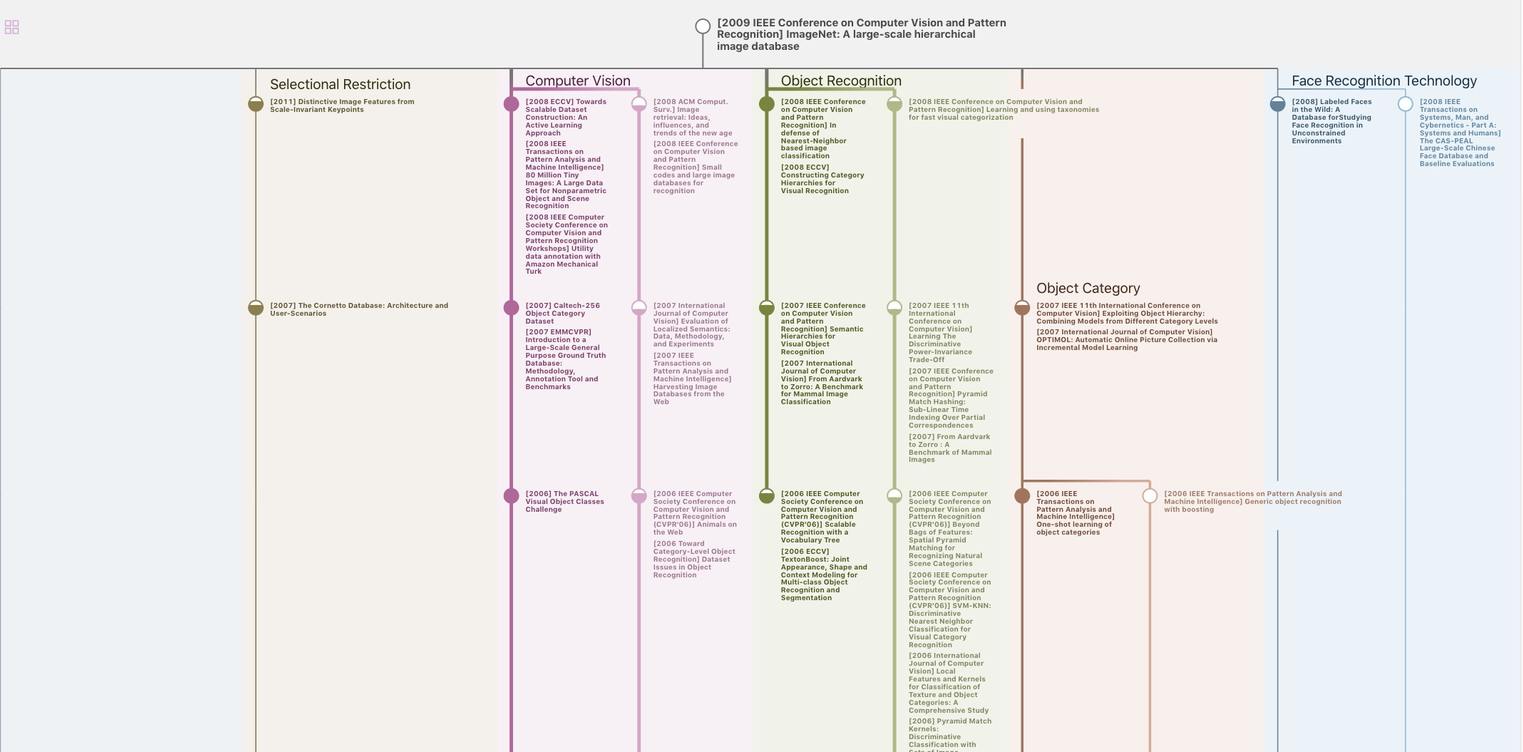
生成溯源树,研究论文发展脉络
Chat Paper
正在生成论文摘要