Molecular optimization of phase III trial failed anticancer drugs using target affinity and toxicity-centered multiple properties reinforcement learning
CLINICAL CANCER RESEARCH(2020)
摘要
Despite the potential of anticancer drugs in the early stages of drug development, many clinical trials have failed at phase III because of low efficacy and/or high toxicity profiles. To improve such issues, effective lead optimization based on machine learning (ML) is needed. Many ML-based lead optimization models have been proposed, but they are capable of optimizing only a single property of a drug; therefore, multiple models are required and would likely be significantly different from the original drug as processed by multiple models. Our contributions are as follows: 1) We devised a new deep learning architecture based on a long short-term memory on a convolutional neural network for predicting binding affinity score (AS) and absorption, distribution, metabolism, excretion, and toxicity (ADMET) prediction. 2) We adapted the Molecule Deep Q-Networks (MoleDQN) to our new reward combinations so that a single model can consider multiple properties such as quantitative estimate of drug-likeness (QED), AS, synthetic accessibility scores (SAS), and toxicity scores. 3) Binding affinity estimator and ADMET estimator are used to indicate drug efficacy and toxicities. Iniparib, brivanib, and rebimastat, which failed in phase III clinical trials, were used for this analysis, and the top two optimized candidates of each drug with an improved score were selected. Iniparib was developed as a PARP inhibitor, but QED and AS were not predicted as a potential drug in our model (QED, 0.51; SAS, 2.23; AS, 5.02). This may be related to the low efficacy reported in clinical trials. Thus, iniparib was optimized for improved QED and AS to increase efficacy. Optimized candidates OPT-INI-01 (QED, 0.85; SAS, 3.33; AS, 7.77) and OPT-INI-02 (QED, 0.83; SAS, 3.15; AS, 7.93) were qualified with QED higher than 0.8 and demonstrated AS higher than 7.0, which is equivalent to Kd Citation Format: Sungsoo Park, Yoon Ho Ko, Bora Lee, Bonggun Shin, Bo Ram Beck. Molecular optimization of phase III trial failed anticancer drugs using target affinity and toxicity-centered multiple properties reinforcement learning [abstract]. In: Proceedings of the AACR Special Conference on Advancing Precision Medicine Drug Development: Incorporation of Real-World Data and Other Novel Strategies; Jan 9-12, 2020; San Diego, CA. Philadelphia (PA): AACR; Clin Cancer Res 2020;26(12_Suppl_1):Abstract nr 35.
更多查看译文
AI 理解论文
溯源树
样例
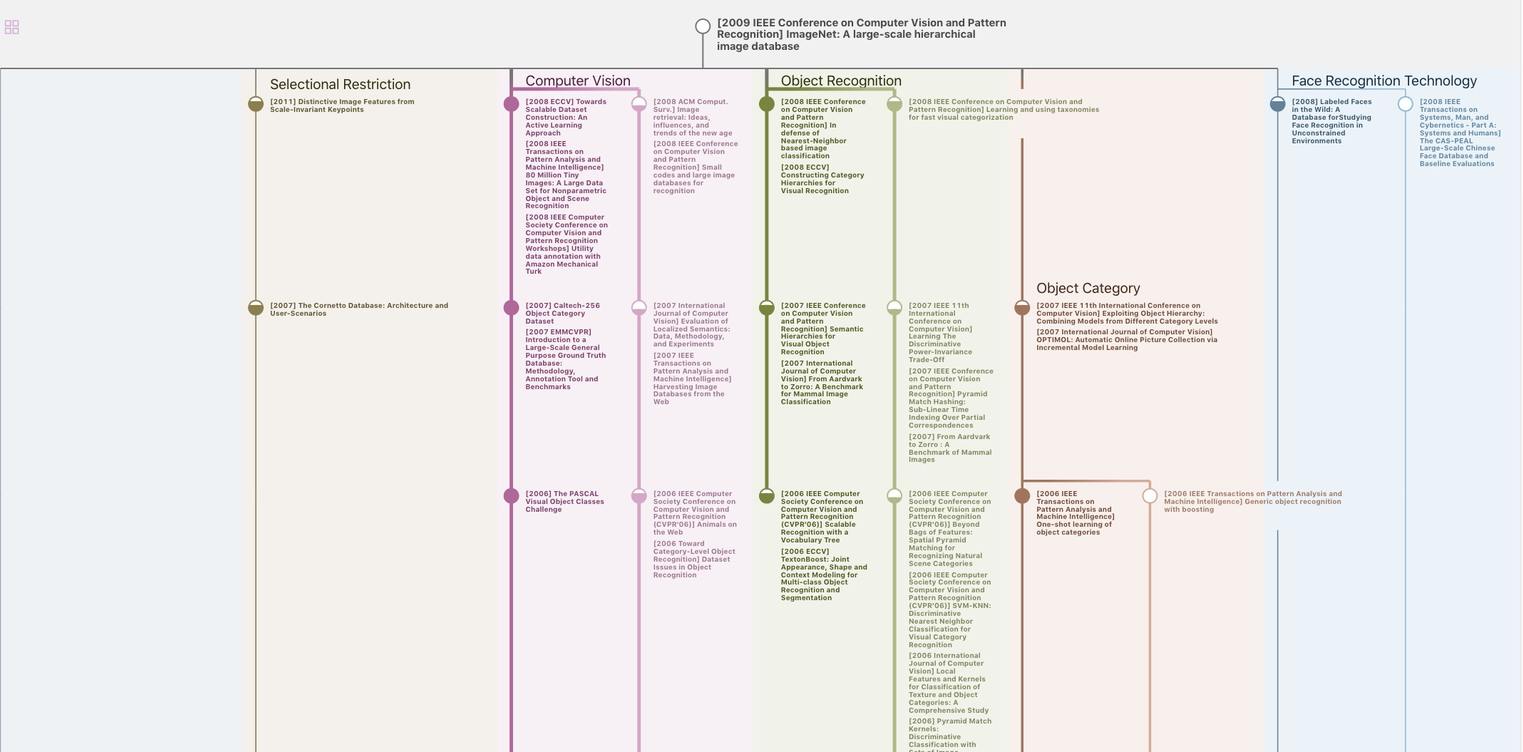
生成溯源树,研究论文发展脉络
Chat Paper
正在生成论文摘要