Density-Aware Curriculum Learning for Crowd Counting
IEEE Transactions on Cybernetics(2022)
摘要
Recently, crowd counting draws much attention on account of its significant meaning in congestion control, public safety, and ecological surveys. Although the performance is improved dramatically due to the development of deep learning, the scales of these networks also become larger and more complex. Moreover, a large model also entails more time to train for better performance. To tackle these problems, this article first constructs a lightweight model, which is composed of an image feature encoder and a simple but effective decoder, called the pixel shuffle decoder (PSD). PSD ends with a pixel shuffle operator, which can display more density information without increasing the number of convolutional layers. Second, a density-aware curriculum learning (DCL) training strategy is designed to fully tap the potential of crowd counting models. DCL gives each predicted pixel a weight to determine its predicting difficulty and provides guidance on obtaining better generalization. Experimental results exhibit that PSD can achieve outstanding performance on most mainstream datasets while training under the DCL training framework. Besides, we also conduct some experiments about adopting DCL on existing typical crowd counters, and the results show that they all obtain new better performance than before, which further validates the effectiveness of our method.
更多查看译文
关键词
Curriculum
AI 理解论文
溯源树
样例
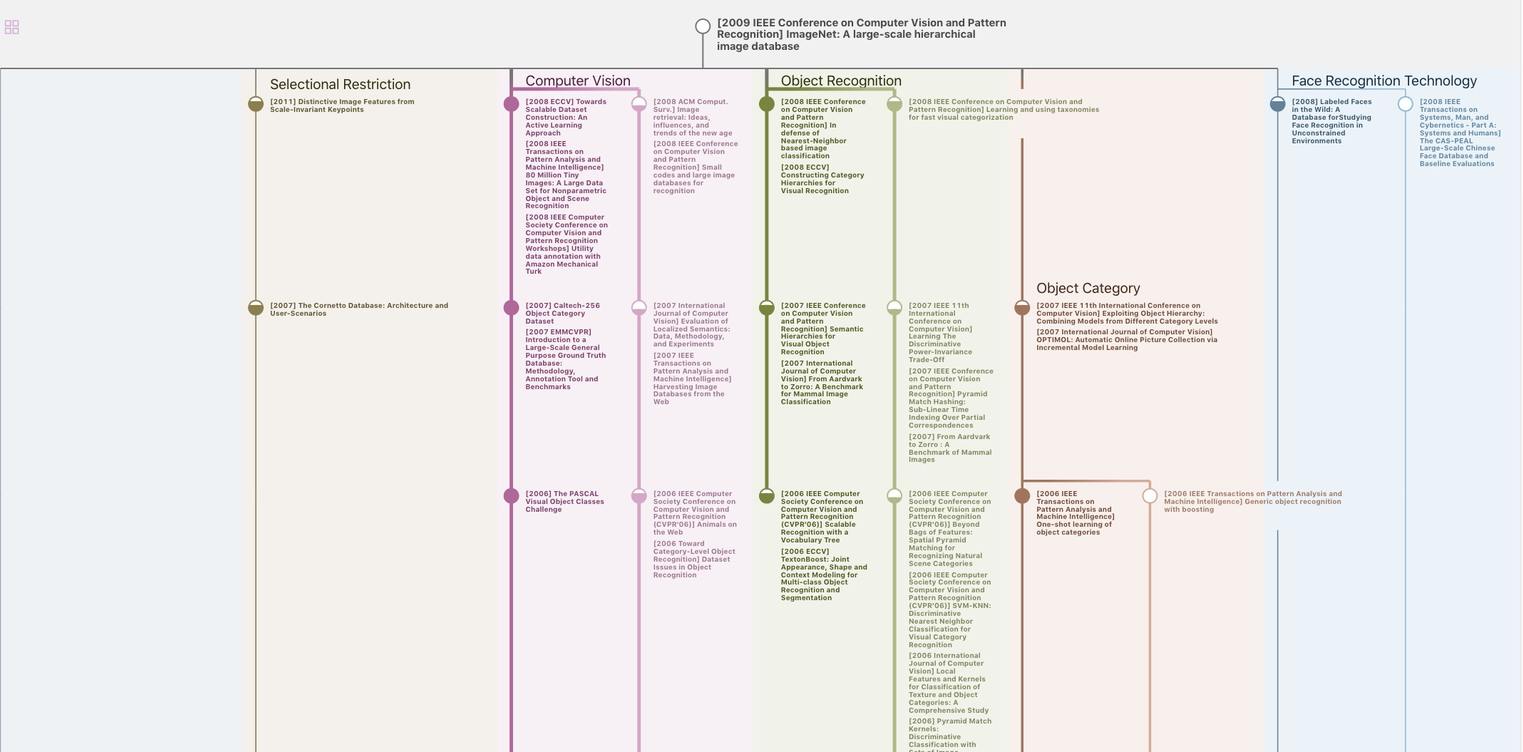
生成溯源树,研究论文发展脉络
Chat Paper
正在生成论文摘要