Machine learning and hybrid metrology using scatterometry and LE-XRF to detect voids in copper lines
Proceedings of SPIE(2019)
摘要
Voids in copper lines are a common failure mechanism in the back end of line (BEOL) of integrated circuits manufacturing, affecting chip yield and reliability. As subsequent process nodes continue to shrink metal line dimensions, monitoring and control of these voids gain more and more importance [1]. Currently, there is no quantitative in-line metrology technique that allows voids to be identified and measured. This work aims to develop a new method to do so, by combining scatterometry (also referred to as Optical Critical Dimension or Optical CD) and low-energy x-ray fluorescence ( LE-XRF), as well as machine learning techniques. By combining the inputs from these tools in the form of hybrid metrology, as well as with the incorporation of machine learning methods, we create a new metric, referred to as V-xo, to characterize the quantity of void. Additionally, the results are compared with in-line electrical test data, as higher amounts of voids were expected to increase the measured resistivity. This was not found to be the case, as the impact of the voids was much less of a factor than variation in the cross-sectional area of the lines.
更多查看译文
关键词
Scatterometry,machine learning,XRF,hybridized metrology,BEOL,voids
AI 理解论文
溯源树
样例
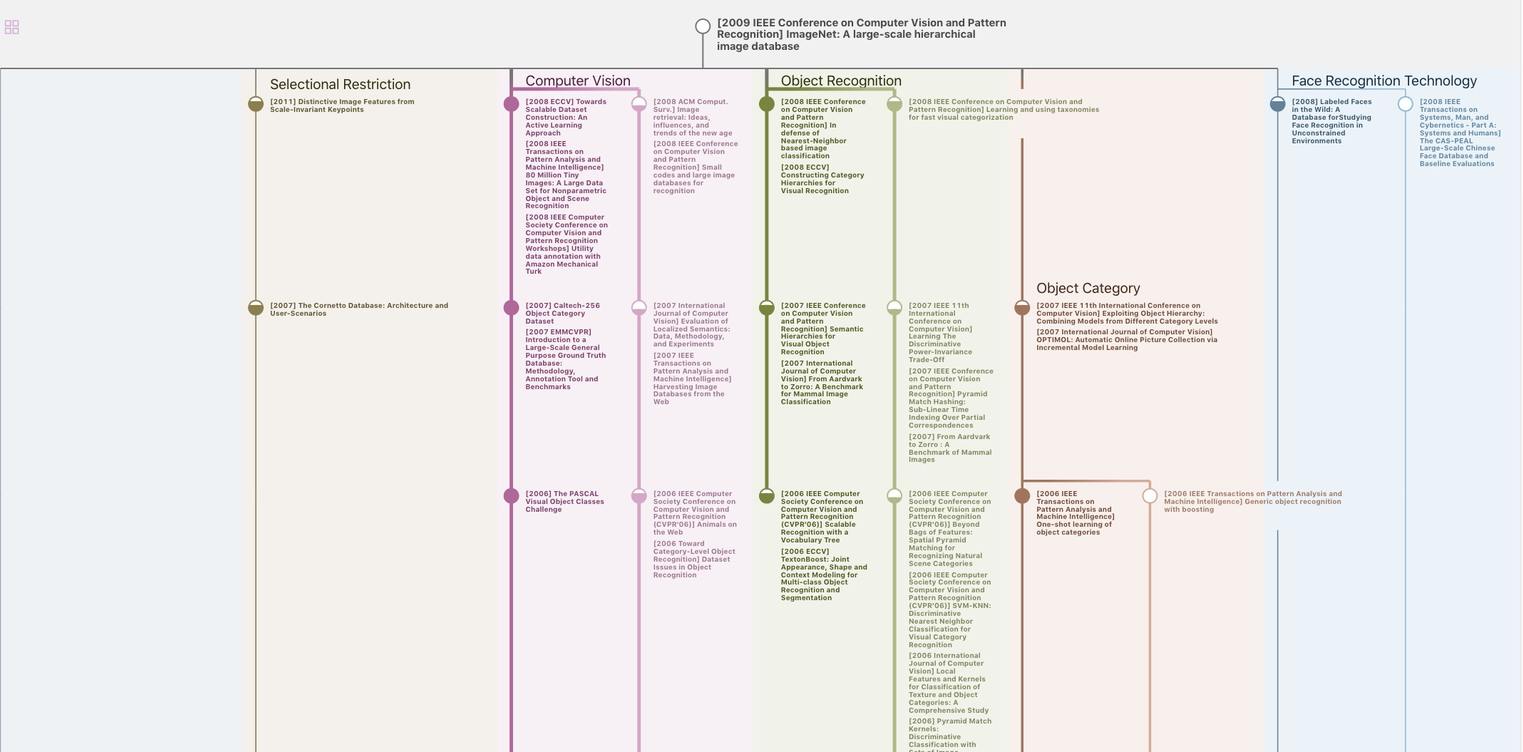
生成溯源树,研究论文发展脉络
Chat Paper
正在生成论文摘要