Optimized One vs One Approach in Multiclass Classification for Early Alzheimer's Disease and Mild Cognitive Impairment Diagnosis
IEEE ACCESS(2020)
摘要
The detection of Alzheimer's Disease in its early stages is crucial for patient care and drugs development. Motivated by this fact, the neuroimaging community has extensively applied machine learning techniques to the early diagnosis problem with promising results. The organization of challenges has helped the community to address different raised problems and to standardize the approaches to the problem. In this work we use the data from international challenge for automated prediction of MCI from MRI data to address the multiclass classification problem. We propose a novel multiclass classification approach that addresses the outlier detection problem, uses pairwise t-test feature selection, project the selected features onto a Partial-Least-Squares multiclass subspace, and applies one-versus-one error correction output codes classification. The proposed method yields to an accuracy of 67% in the multiclass classification, outperforming all the proposals of the competition.
更多查看译文
关键词
Alzheimer's disease,CAD,error correcting output codes,mild cognitive impairment,multiclass classification,one versus one,partial least squares,random forests,support vector machines
AI 理解论文
溯源树
样例
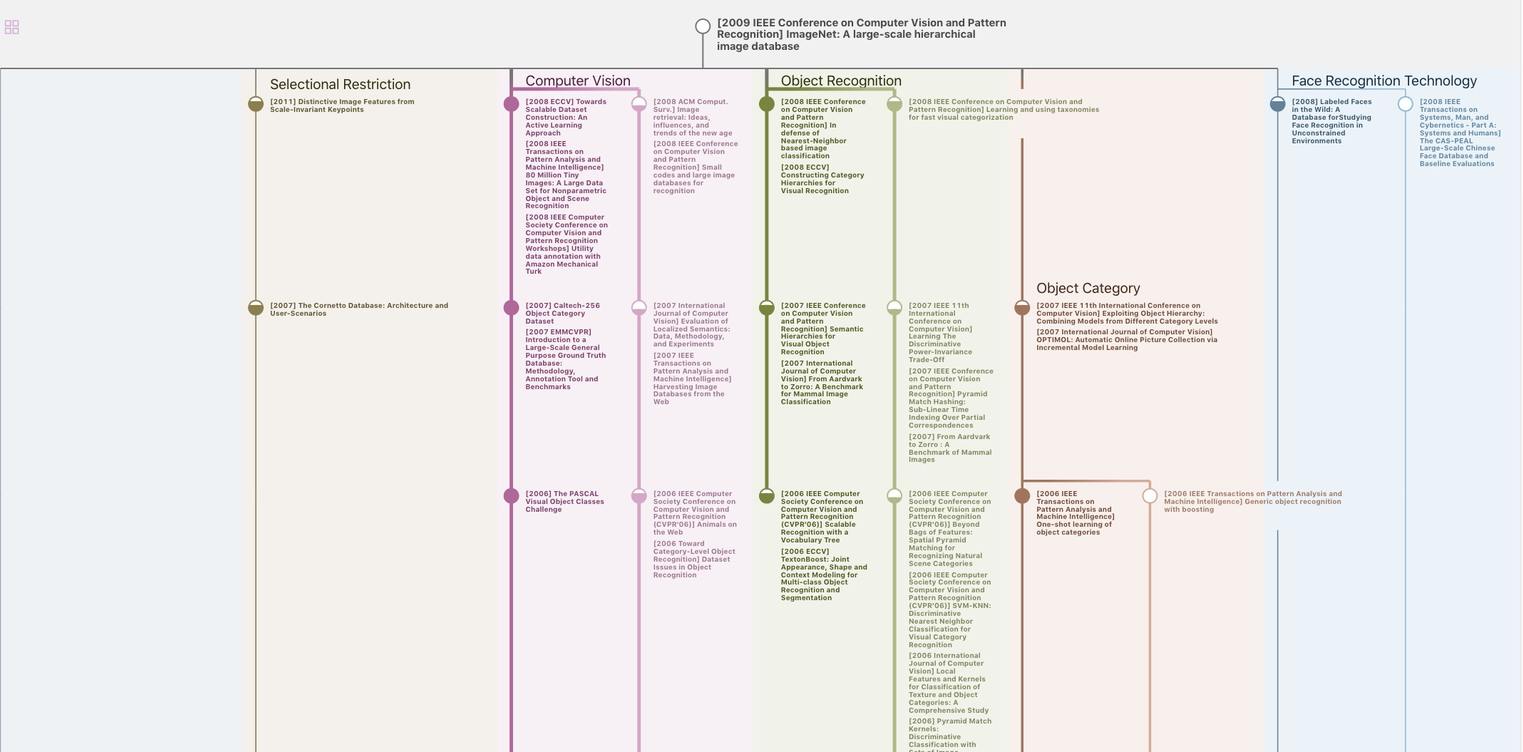
生成溯源树,研究论文发展脉络
Chat Paper
正在生成论文摘要