Generative Models for Sampling of Lattice Field Theories
arxiv(2020)
摘要
We explore a self-learning Markov chain Monte Carlo method based on the Adversarial Non-linear Independent Components Estimation Monte Carlo, which utilizes generative models and artificial neural networks. We apply this method to the scalar φ^4 lattice field theory in the weak-coupling regime and, in doing so, greatly increase the system sizes explored to date with this self-learning technique. Our approach does not rely on a pre-existing training set of samples, as the agent systematically improves its performance by bootstrapping samples collected by the model itself. We evaluate the performance of the trained model by examining its mixing time and study the ergodicity of generated samples. When compared to methods such as Hamiltonian Monte Carlo, this approach provides unique advantages such as the speed of inference and a compressed representation of Monte Carlo proposals for potential use in downstream tasks.
更多查看译文
AI 理解论文
溯源树
样例
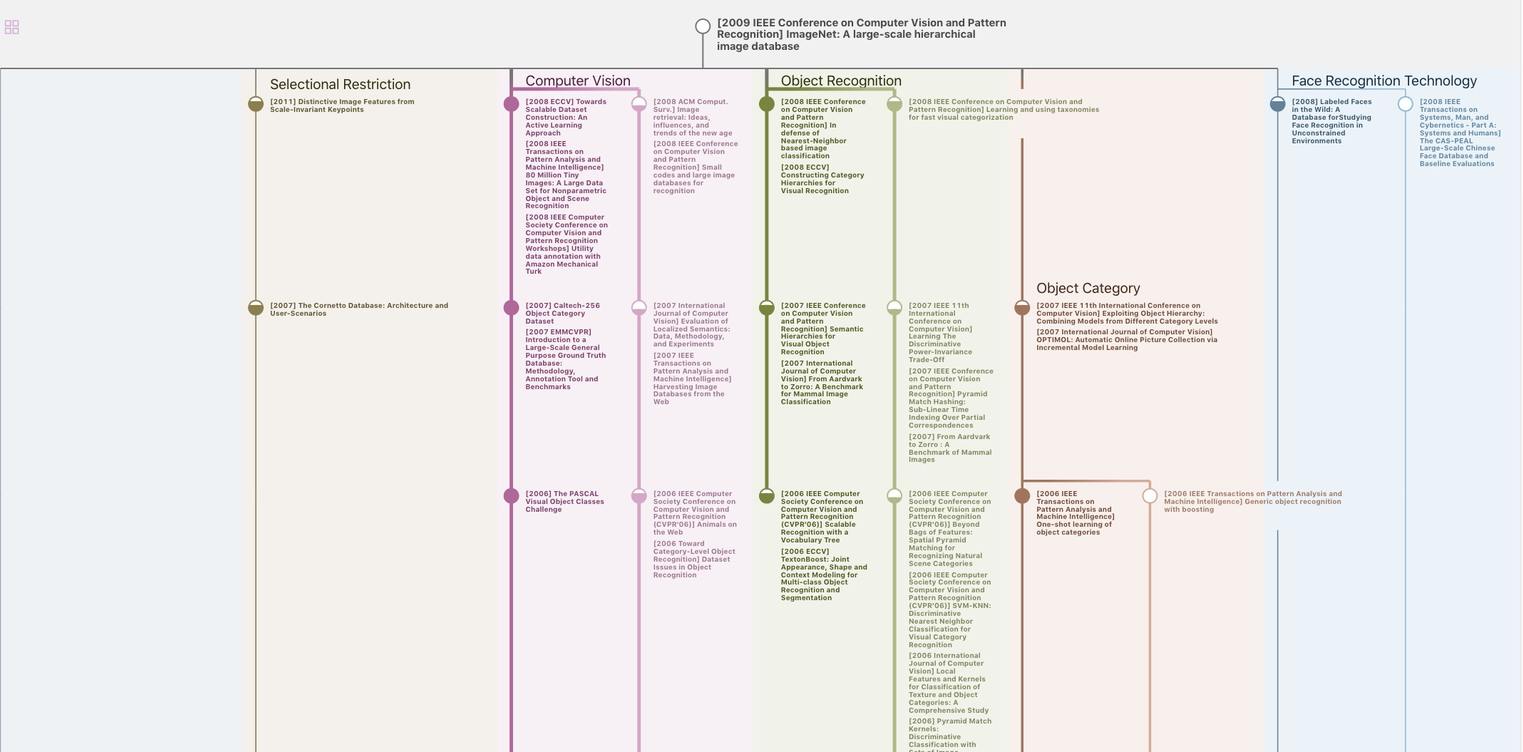
生成溯源树,研究论文发展脉络
Chat Paper
正在生成论文摘要